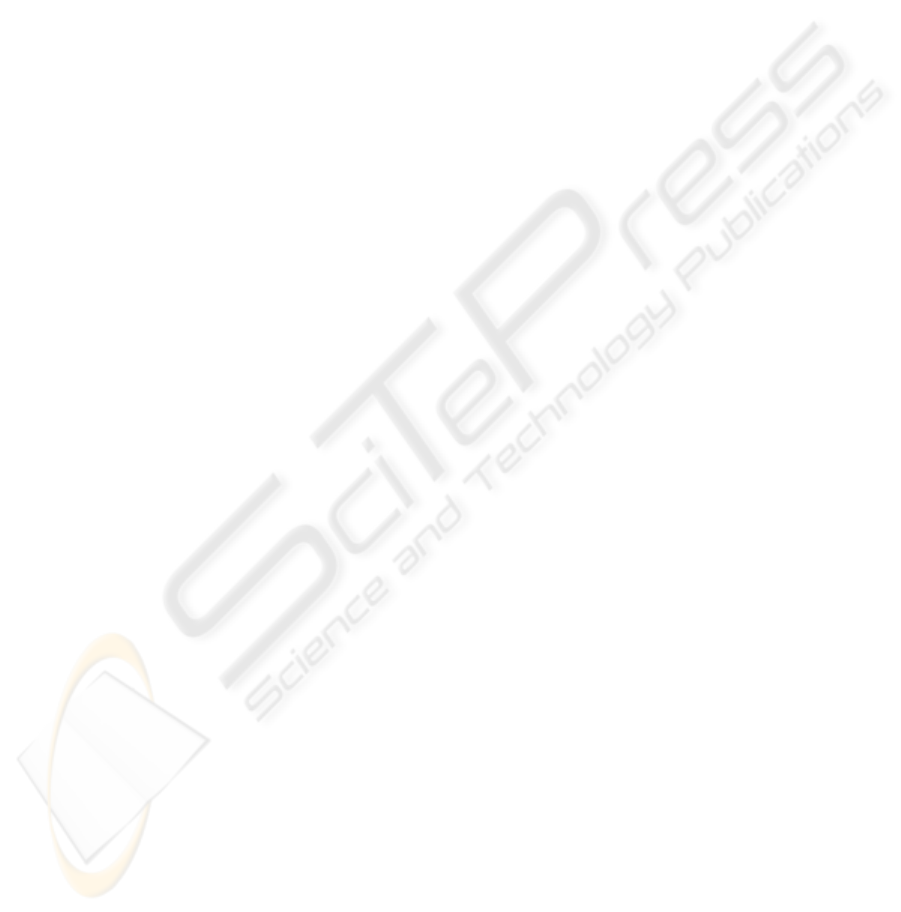
among various vaccination program, safe childbirth
practice and IMR, and secondly to note which one of
the data mining techniques could be more suitable to
explain such relationships. From our experiment
based on the archived data of ten countries, we have
noticed the following,
One-way ANOVA result shows that OPV, M,
DPT, BCG and ADR are significantly dissimilar
from each other (p<0.05; F score>F
crit
) and thus can
be suitably used for predictive modeling
(regressions) as different attributes though under the
same construct (child immunization program)
Simple regressions fail to predict any significant
correlation between any of the QA (OPV, M, DPT,
BCG and ADR) and the CA, i.e. IMR as indicated
by R-sq values <50% for each analysis. But the
attempt is said to be a good one as the residual plots
are almost linear in nature without any visible
outlier.
QAR relies on 2-D grid combinations of QA and
generation of AR from confidence (c) level. From
the association rules, thus generated, it is found that
QAR is a better approach to engineer this kind of
data where direct relations cannot be statistically
predicted, but assumed. In this experiment it is
found that all the QA(s) are closely associated and
correlated with each other. From the experiment it is
found that with a combination of OPV, M and ADR
the IMR is quite low in Maldives and Thailand,
however in contrast to that Bhutan and Sri Lanka
shows a higher values. This may be due to influence
of other factors, e.g. general healthcare facilities,
literacy rate, crude birth rate and so forth. From the
other combinations DPT-BCG-ADR it is found that
rules 2,4,5 are strong rules that tells that if a baby is
born under a skilled health worker it receives DPT
and BCG vaccines and vice versa. It is true for
Thailand and Bangladesh i.e. this combination may
have reduced the IMR (but not with Bhutan).
From the ‘lift’ value, it may be observed that
there is a negative correlation between vaccination
and skilled childbirth with that of IMR, i.e. for
higher the number of immunisations and skilled
childbirth under supervisions, lower is the incidence
of IMR in a population.
However, it is important to mention here that
association mining based on a mathematical
approach may not always explain a real-world
scenario, as seen in the contrasting results of Bhutan
and Thailand. Therefore, consideration of other
health indicators could be considered in this type of
study.
REFERENCES
http://www.who.int/whosis/database/core/core_select.cfm
El-Ghannam A. R., 2003. The global problems of child
malnutrition and mortality in different world regions,
J Health Soc Policy 16(4), 1-26.
D'souza R. M., Bryant J. H., 1999. Determinants of
childhood mortality in slums of Karachi, Pakistan, J
Health Popul Dev Ctries 2(1), 33-44.
Byass P., 2003. FilaBavi Study Group, Patterns of
mortality in Bavi, Vietnam, 1999-2001, Scand J
Public Health Suppl. 62, 8-11.
Hynes M., Sheik M., Wilson H. G., Spiegel P., 2002.
Reproductive health indicators and outcomes among
refugee and internally displaced persons in post
emergency phase camps, JAMA, 288(5), 595-603.
Bhatia S., Dranyi T., Rowley D., 2002. A social and
demographic study of Tibetan refugees in India, Soc
Sci Med. 54(3), 411-22.
Hossain M. B., Phillips J. F., Pence B., 2007. The effect of
women's status on infant and child mortality in four
rural areas of Bangladesh, J Biosoc Sci. 39(3), 355-66.
Lindström C., Lindström M., 2006. Social capital," GNP
per capita, relative income, and health: an ecological
study of 23 countries, Int J Health Serv. 36(4), 679-96.
Hales S., Howden-Chapman P., Salmond C., Woodward
A., Mackenbach J., 1999. National infant mortality
rates in relation to gross national product and
distribution of income, Lancet, 354(9195), 2047.
Wu J. C., Chiang T. L., 2007. Comparing child mortality
in Taiwan and selected industrialized countries, J
Formos Med Assoc., 106(2), 177-180.
http://www.unicef.org/infobycountry/stats_popup1.htm1.
King G., Zeng L., 2001. Improving forecasts of state
failure, World Politics 53(4), 623–658.
International statistical classification of diseases and
related health problems, 1993. 10
th
Revision. Vol 2.
Geneva, Switzerland: World Health Organization,
129.
http://www.unicef.org/publications/index_18108.html.
MacDorman M. .F., Callaghan W. M., Mathews T. J.,
Hoyert D. L., Kochanek K. D., 2007. Trends in
preterm-related infant mortality by race and ethnicity,
United States, 1999-2004, Int J Health Serv 37(4),
635-41.
Huy T. Q., Johansson A., Long N. H., 2007. Reasons for
not reporting deaths: a qualitative study in rural
Vietnam, World Health Population 9(1), 14-23.
Rastogi V. B., 2006. Fundamental of Biostatistics,
Rashtriya Printers, New Delhi, India, 77-196.
Han J., Kamber M., 2006. Data Mining Concepts and
Techniques, 2
nd
Edition, Morgan Kauffmann
Publishers, An imprint of Elsevier, San Francisco, CA,
USA, 259-261.
ICSOFT 2008 - International Conference on Software and Data Technologies
320