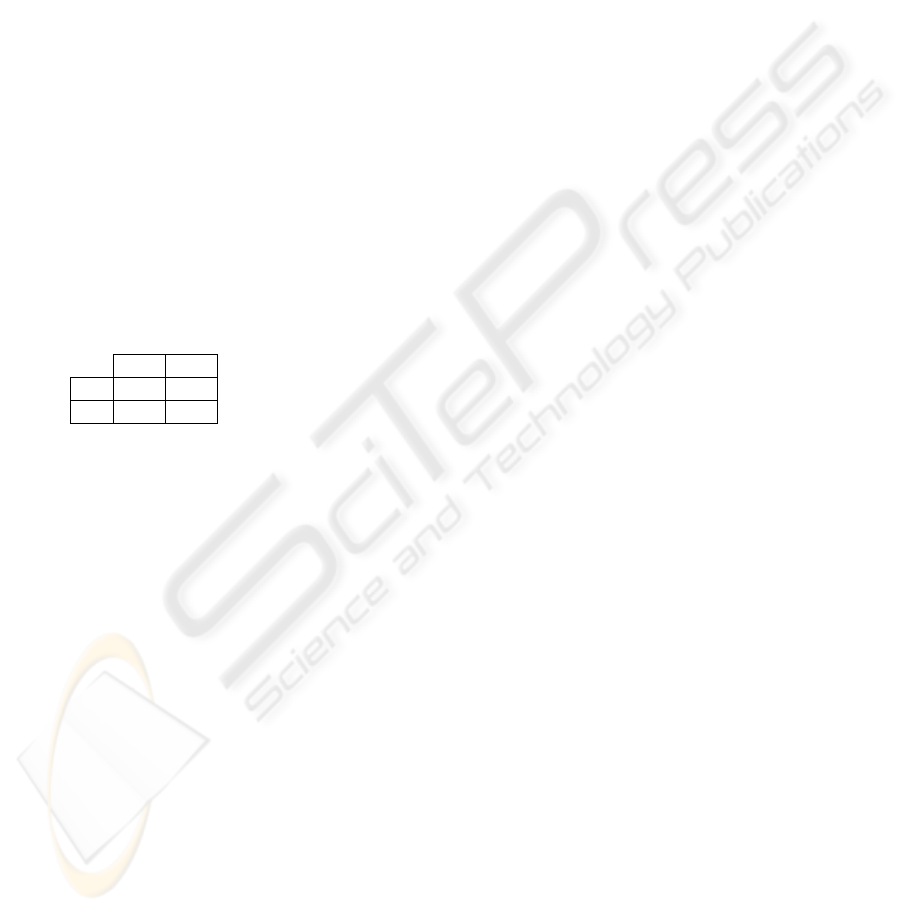
number of required genes, but also improves the
success % obtained. These genes, located as
following indicated, are able to carry out the
classification with 2 errors on 10 cases of the testing
set.
868; 929; 920; 1170; 792; 1050; 556; 680; 458
The final GA result obtains only one failure on
10 testing cases and 7 optimal genes, therefore the
classification has improved: 3 genes less and lower
error rate. The 7 optimal genes are located as
follows:
929; 920; 792; 1050; 556; 680; 458
Once the proposed GA was tested with the data
reported by Roberts et al, this algorithm was applied
establishing 100 as maximum number of genes
(maximum length of the individual). In this way, the
GA will randomly select for each individual n
positions (genes) among the existing 12625 by
using, as it was during the previous case, 79 cases
for training and 10 for testing.
The final result obtained by the proposed GA is a
group of 6 genes that achieve 100% accuracy during
training and testing (Table 3).
Table 3: Testing confusion matrix.
+ -
+ 3 0 (true pos)
- 0 7 (true neg)
These genes are located as follows:
955; 1149; 920; 1168; 71; 903
6 CONCLUSIONS
This paper shows a general outline for the selection
and classification of genes obtained from data of
DNA microarrays. The proposed GA-SVM in this
paper starting with individuals of the GA provided
by Roberts et al achieves better predictive
capability, 90% success rate with 7 genes, that the
method proposed by Roberts, who achieved 80%
success rate with 10 genes. The results prove that is
a method capable of achieving highly precise
classifications. More specifically, in the case showed
here, the success rate has been 100% using only 6
genes (see Table 3).
ACKNOWLEDGEMENTS
This work was partially supported by the Spanish
Ministry of Education and Culture (Ref TIN2006-
13274) and the European Regional Development
Funds (ERDF), grant (Ref. PIO52048 and
RD07/0067/0005) funded by the Carlos III Health
Institute, grant (Ref. PGIDIT 05 SIN 10501PR) and
(Ref. PGIDIT 07 TMT011CT) from the General
Directorate of Research of the Xunta de Galicia and
grant (File 2006/60) from the General Directorate of
Scintific and Technologic Promotion of the Galician
University System of the Xunta de Galicia. The
work of Juan L. Pérez is supported by an FPI grant
(Ref. BES-2006-13535) from the Spanish Ministry
of Education and Science.
REFERENCES
Bonilla, D., Duval, B., Hao, J., 2006. A Hybrid GA/SVM
Approach for Gene Selection and Classification of
Microarray Data. In EvoWorkshops, LNCS 3907: 34-
44.
Brown, M., Grundy, W., Lin, D., Cristianini, N., Sugnet,
C., Ares, M., Haussler, D., 1999. Support vector
machine classification of microarray gene expression
data. University of California, Santa Cruz, Technical
Report: Ucsc-Crl-99-09.
Furey, T.S., Cristianini, N., Duffy, N., Bednarski, D.W.,
Schummer, M., Haussler, D., 2000. Support vector
machine classification and validation of cancer tissue
samples using microarray expression data.
Bioinformatics, 16(10):906-914.
Guyon, I., Elisseeff, A., 2003. An introduction to variable
and feature selection. In Journal of Machine Learning
Research, 3:1157-1182.
Guyon, I., Weston, J., Barnhill, S., Vapnik, V., 2002. Gene
selection for cancer classification using support vector
machines. Machine Learning, 46(1-3): 389-422.
Huang, E., Cheng, SH., Dressman, H., Pittman, J., Tsou,
MH., Horng, CF., Bild, A., Iversen, ES., Liao, M.,
Chen, CM., West, M., Nevins, JR., Huang, AT., 2003.
Gene Expression Predictors of Breast Cancer
Outcomes. Lancet, 361(9369): 1590-1596.
Lee, Y-J., Mangasarian, O.L., Wolberg, W.H., 2000.
Breast cancer survival and chemotherapy: a support
vector machine analysis. Dimacs Series In Discrete
Mathematics and Theoretical Computer Science, vol
55:1-10.
Nahar, J., Phoebe, Y., Shawkat, ABM., 2007. Microarray
classification and rule based cancer identification. In
International Conference on Information and
Communication Technology. Bangladesh.
Reddy, A.R., Deb, K., 2003. Classification of two-class
cancer data reliably using evolutionary algorithms.
Technical Report. DanGAL.
Roberts. S., 2005. Using Genetic Algorithms to Select a
Subset of Predictive Variables from a High-
Dimensional Microarray Dataset. Matlab Digest.
Sewell M., 2008. Martin Sewell web site.
http://martinsewell.com/
HYBRID SYSTEM FOR DATA CLASSIFICATION OF DNA MICROARRAYS WITH GA AND SVM
307