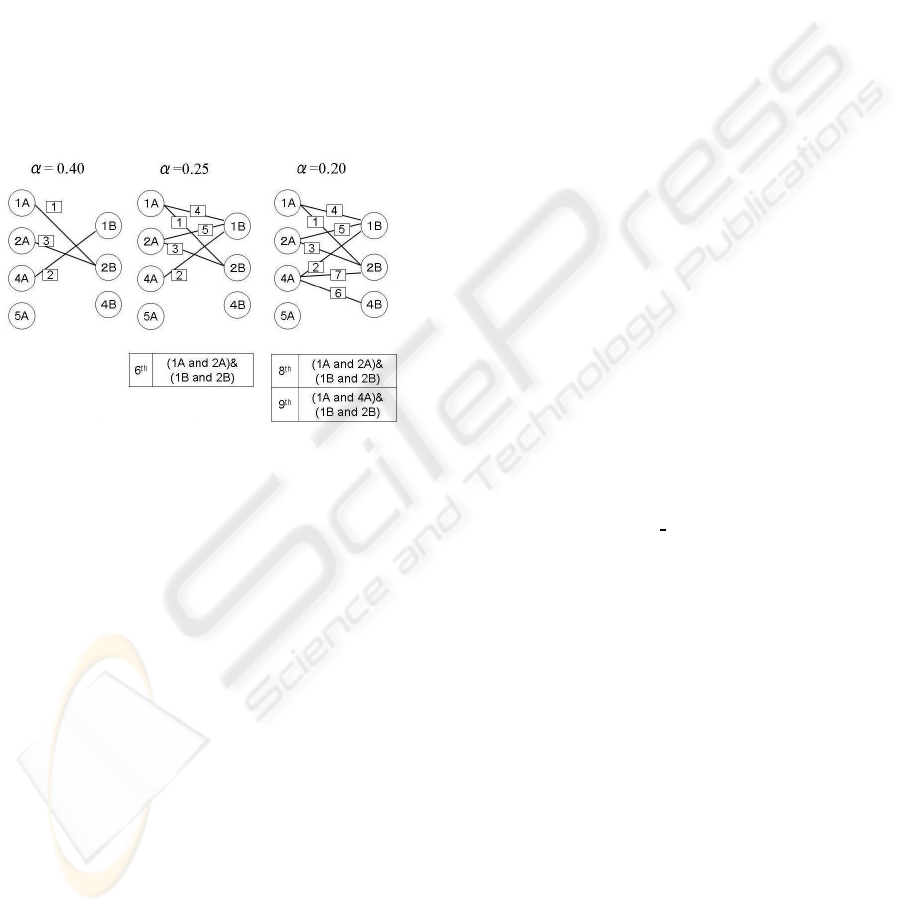
old as a parameter. The number of candidates in-
creases when the threshold decreases. This is because
of the weakness of co-occurrence. The number of
pairs with a transition rate grows when the threshold
decreases. The 6th choice is (1A and 2A) & (1B and
2B) when α = 0.25. 1A and 2A correspond to ”Inter-
net” and ”VoIP” respectively, while 1B and 2B cor-
respond to ”connection is OK” and ”no connection”
respectively. We can classify the text in a semantic
sense, e.g., ”Internet is OK, but VoIP has no connec-
tion”.
This type of proposed data classification can get
an overview of all the possible patterns of a problem
and establish coping processes in advance. Further-
more, it has possibilities to mine the potential cus-
tomer requirement that leads to new business.
Figure 7: Threshold and pairs.
6 CONCLUSIONS
A classification technique for customer enquiries is
needed due to the increasing complexity of the con-
nections in end-to-end networks in the telecom oper-
ating field. In this paper, we proposed one method
for analyzing and classifying customer enquiries that
enables quick and efficient responses. Because cus-
tomer enquiries are generally stored as unstructured
textual data, this method is based upon morphologi-
cal analysis and co-occurrence techniques to enable
classification of a large amount of unstructured data
into patterns. We applied the proposed method to
1000 customer enquiries and evaluated its effective-
ness. The method can apply not only to establish cop-
ing processes in advance but also to mine potential
requirement for new business.
We are currently conducting further study on ap-
plying this method to large amounts of data and on
determining a threshold for telecom operation.
REFERENCES
Benzecri, J.-P. (1992). Correspondence Analysis Hand-
book. Marcel Dekker.
Cutting, D., Kager, D., and Tukey, J. (1992). Scatter/gather:
A cluster-based approach to browsing large document
colelctions. In Proc. 15th Annual International ACM
SIGIR Conference on Research and Development in
Information Retrieval.
Hayashi, C. (1993). Quantification -Theory and Method.
Asakura-shoten.
Ho, X., Ding, C., Zha, H., and Simon, H. (2001). Automatic
topic identification using webpage clustering. In Proc.
2001 IEEE International Conference on Data Mining.
Leuski, A. (2001). Evaluating document clustering for in-
teractive information retrieval. In Proc. 2001 ACM
International Conference on Information and Knowl-
edge Management.
Masuo, Y., Ohsawa, Y., and Ishizuka, M. (2001). Document
as a small word. In Proc. JSAI 2001, International
workshop (LNAI2253), pages 444–448.
Naganuma, K., Isonishi, T., and Aikawa, T. (2005). Diamin-
ing: Text mining solution for customer relationship
management. Mitsubishi Technical Report, 79-4:259–
262.
Newman, M. (2005). Power laws, pareto distributions and
zipf’s law. Contemporary Physics, 46:323–351.
Ohsawa, Y., Benson, N., and H.Yachida (1997). Keygraph:
Automatic indexing by co-occurrence graph based on
building construction metaphor. In Proc. IEEE Forum
on Research and Technology Advances in Digital Li-
braries.
Ohsumi, N. (2006). Mining of textual data. recent trend
and its direction. http://wordminer.comquest.co.jp
/wmtips/pdf/20060910
1.pdf.
Rodoriguezd, M., Gomez-Ilidalgo, J., and Diaz-Agudo, B.
(1998). Using wordnet to complement training in-
formation in text categorization. In Proc. Recent Ad-
vances in Natural Language Processing.
Sato, S., Fukuda, K., Sugawara, S., and Kurihara, S. (2007).
On the relationship between word bursts in docu-
ment streams and clusters in lexical co-occurrence
networks. IPSJ, 48-SIG14:69–81.
Sullivan, D. (2001). Document Warehousing and Text Min-
ing. John Wiley.
Takahashi, S. (1996). Correspondence Analysis by Excel.
Ohm-sya.
Toda, H., Kataoka, R., and Kitagawa, H. (2005). Clustering
news articles using named entities. IPSJ SIG Techni-
cal Report, 2005-DBS-137:175–181.
Uejima, H., Miura, T., and Shioya, I. (2004). Improving
text categorization by synonym and polysemy. Trans.
on IECIE, J87-D-I, No. 2:137–144.
Zipf, G. (1949). Human Behavior and the Principle of Least
Effort. Addison-Wesley.
USING CO-OCCURRENCE TO CLASSIFY UNSTRUCTURED DATA IN TELECOMMUNICATION SERVICES
17