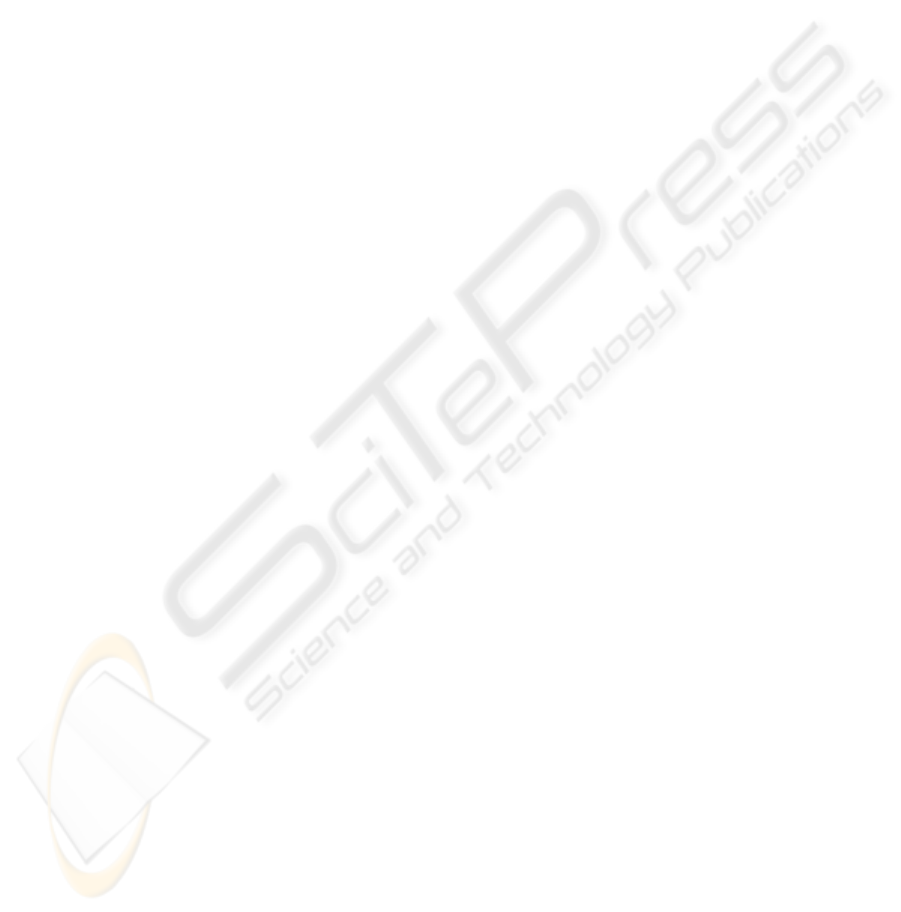
5 EVALUATING MOBILE
SOLUTIONS
Our research agenda has been evolving around the
theme of finding relevant content that will satisfy a
user’s query. To this extent, we have been proposing
various algorithms to: select relevant content, based
on dynamically inferred tags semantics; rank filtered
content based on quality, by dynamically assessing
content sources’ reputation. Will these algorithms
become enabling technologies for pervasive content
sharing applications? In order to answer this question,
we (and the research community working on these
topics) is faced with a big challenge: how to evalu-
ate these algorithms.
Data about content and content sharing abound on
the Internet; however,conducting studies on such data
inevitably fails to measure what would happen in a
truly distributed setting. On the other hand, there ex-
ist plenty of experimental observations of how peo-
ple move while carrying their portable devices; in this
case, though, there is little or no information about
what content people produce and consume.
As a short-term solution, researchers can “mimic”
what would happen in a real pervasive system, by
overlaying these different datasets; however, doing so
in a meaningful way is a research question of its own.
Simulation should be coupled with controlled exper-
iments; the problem in so doing is that those studies
are expensive, so one tends to trade off between (user)
sample size, time requirements, and monetary costs;
the generality of the results obtained thus becomes
questionable. To help solve this problem, PARC re-
searchers have recently proposed to collect user mea-
surements from micro-task markets (such as Ama-
zon’s Mechanical Turk) (Kittur et al., 2008). In the
long run, an actual large-scale system deployment
will be needed.
6 CONCLUSIONS
In this paper, we have discussed distributed mech-
anisms with which mobile users can find content
of interest and of high quality. Compared to ex-
isting (centralized) mechanisms, distributed mecha-
nisms promise to scale and be fully open to innova-
tion. However, to deliver on this this promise, we still
need to study how effective those mechanisms are in
practice. The lack of real datasets, combining mobil-
ity with user’s interests and content, makes evaluating
these mechanisms an open challenge.
REFERENCES
Anderson, R. (1996). The Eternity Service. In Proc. of
Pragocrypt.
Bassoli, A., Brewer, J., Martin, K., Dourish, P., and Main-
waring, S. (2007). Underground Aesthetics: Rethink-
ing Urban Computing. IEEE Pervasive Computing,
6(3):39–45.
Buchegger, S. and Boudec, J.-Y. L. (2004). A robust repu-
tation system for P2P and mobile ad-hoc networks. In
Proc. of the 2
nd
Workshop on the Economics of Peer-
to-Peer Systems.
Capra, L. (2005). Reasoning about Trust Groups to Coordi-
nate Mobile Ad-Hoc Systems. In Proc. of the 1
st
IEEE
Workshop on the Value of Security Through Collabo-
ration, Athens, Greece.
Foucault, M. (1998). Of other space. The visual culture
reader.
Halpin, H., Robu, V., and Shepherd, H. (2007). The com-
plex dynamics of collaborative tagging. In Proc. of
the 16th Intl. Conference on World Wide Web, pages
211–220, NY, USA.
Harada, T., Gyota, T., Kuniyoshi, Y., and Sato, T. (2007).
Development of Wireless Networked Tiny Orienta-
tion Device for Wearable Motion Capture and Mea-
surement of Walking Around, Walking Up and Down,
and Jumping Tasks. In Proceedings of the IEEE Con-
ference of Intelligent Robots and Systems, San Diego,
US.
Herbster, M., Pontil, M., and Wainer, L. (2005). Online
learning over graphs. In Proc. of the 22
nd
Int. Confer-
ence on Machine Learning.
Heymann, P., Koutrika, G., and Garcia-Molina, H. (2007).
Can Social Bookmarking Improve Web Search? Re-
source Shelf.
Hsu, W. H., Lancaster, J., Paradesi, M. S., and Weninger,
T. (2007). Structural Link Analysis from User Pro-
files and Friends Networks: A Feature Construction
Approach.
Kelkar, S., John, A., and Seligmann, D. (2007). An
Activity-based Perspective of Collaborative Tagging.
Intl. Conference on Weblogs and Social Media.
Kinateder, M. and Rothermel, K. (2003). Architecture and
Algorithms for a Distributed Reputation System. In
Proc. of the 1
st
Intl. Conference on Trust Manage-
ment, pages 48–62, Crete.
Kittur, A., Chi, E., and Suh, B. (2008). Crowdsourcing
User Studies With Mechanical Turk. In Proceedings
of the ACM Conference on Human-factors in Comput-
ing Systems, Florence, Italy.
Koutrika, G., Effendi, F. A., Gy¨ongyi, Z., Heymann, P., and
Garcia-Molina, H. (2007). Combating spam in tag-
ging systems. In Proc. of the 3rd Intl. Workshop on
Adversarial Information Retrieval on the Web, pages
57–64, NY, USA.
L. McNamara, C. Mascolo and L. Capra (2007). Content
Source Selection in Bluetooth Networks. In Proc. of
SELECTING TRUSTWORTHY CONTENT USING TAGS
507