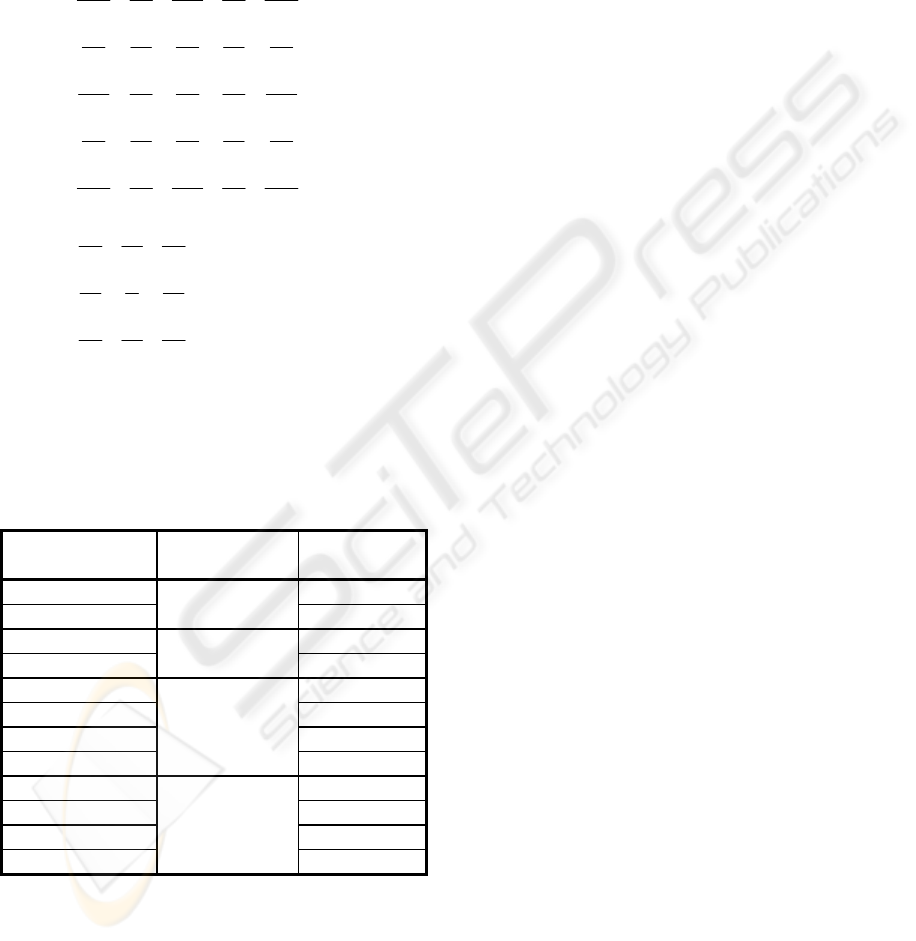
algorithm NFPCA against MMSE (Umbaugh,
1998), AMVR (Umbaugh, 1998), and GMNR.
The values of the variances to model the noise in
images processed by NFPCA represent the
maximum of the variances per pixel resulted from
the decorrelation process. The implementation of the
GMNR algorithm used the masks
⎟
⎟
⎟
⎟
⎟
⎟
⎟
⎟
⎟
⎟
⎟
⎠
⎞
⎜
⎜
⎜
⎜
⎜
⎜
⎜
⎜
⎜
⎜
⎜
⎝
⎛
=
256
1
64
1
128
3
64
1
256
1
64
1
16
1
32
3
16
1
64
1
128
3
32
3
64
9
32
3
128
3
64
1
16
1
32
3
16
1
64
1
256
1
64
1
128
3
64
1
256
1
1
h and
⎟
⎟
⎟
⎟
⎟
⎟
⎠
⎞
⎜
⎜
⎜
⎜
⎜
⎜
⎝
⎛
=
20
1
10
1
20
1
10
1
5
2
10
1
20
1
10
1
20
1
2
h
A synthesis of the comparative analysis on the
quality and efficiency corresponding to the
restoration algorithms presented in the paper is
supplied in Table 1.
Table 1.
Restoration
algorithm
Type of
noise
Mean
error/pixel
MMSE 52.08
AMVR
U(30,80)
10.94
MMSE 50.58
AMVR
U(40,70)
8,07
MMSE 37.51
AMVR 11.54
GMNR 14.65
NFPCA
N(40,200)
12.65
MMSE 46.58
AMVR 9.39
GMNR 12.23
NFPCA
N(50,100)
10.67
REFERENCES
Bacchelli, S., Papi S. 2006, Image denoising using
principal component analysis in the wavelet domain.
In Journal of Computational and Applied
Mathematics, Volume 189, Issues 1-2, 1 May 2006,
Pages 606-621
Balster, E. J., Zheng, Y. F., Ewing, R. L. 2003, Fast,
Feature-Based Wavelet Shrinkage Algorithm for
Image Denoising. In International Conference on
Integration of Knowledge IntensiveMulti-Agent
Systems. KIMAS '03: Modeling, Exploration, and
Engineering Held in Cambridge, MA on 30
September-October 4, 2003
Cocianu, C., State, L., Stefanescu, V., Vlamos, P., 2006,
PCA-Based Data Mining Probabilistic and Fuzzy
Approaches with Applications in Pattern Recognition,
Proceedings of ICSOFT 2006, Portugal, pp. 55-60,
2006
Cocianu, C., State, L., Vlamos, P.,2002, On a Certain
Class of Algorithms for Noise Removal in Image
Processing:A Comparative Study, In Third IEEE
Conference on Information Technology ITCC-2002,
Las Vegas, Nevada, USA, April 8-10, 2002
Cocianu, C., State, L., Stefanescu, V., Vlamos, P., 2004,
On the Efficiency of a Certain Class of Noise Removal
Algorithms in Solving Image Processing Tasks, In:
Proceedings of the ICINCO 2004, Setubal, Portugal
Diamantaras, K.I., Kung, S.Y., Principal Component
Neural Networks: theory and applications, John
Wiley &Sons, 1996
Gonzales, R., Woods, R., Digital Image Processing,
Prentice Hall, 2002
Haykin, S., Neural Networks A Comprehensive
Foundation, Prentice Hall,Inc. 1999
Hyvarinen, A., Karhunen, J., Oja,E., Independent
Component Analysis, John Wiley &Sons, 2001
Hyvarinen, A., Hoyer, P., Oja, P., 1999. Image Denoising
by Sparse Code Shrinkage,
www.cis.hut.fi/projects/ica,
Pitas, I., 1993, Digital Image Processing Algorithms,
Prentice Hall
Portilla, J. 2005, Image Restoration using Gaussian Scale
Mixtures in Overcomplete Oriented Pyramids. In
SPIE's International Symposium on Optical Science
and Technology, SPIE's 50th Annual Meeting, Proc. of
the SPIE, vol. 5914, pp. 468-82, San Diego, CA, Aug
2005
Sonka, M., Hlavac, V., 1997, Image Processing, Analyses
and Machine Vision, Chapman & Hall Computing
Stark, J.L., Murtagh, F., Bijaoui, A., 1995, Multiresolution
Support Applied to Image Filtering and Restoration,
Technical Report
State, L, Cocianu, C, Vlamos, P.., 2001, Attempts in
Using Statistical Tools for Image Restoration
Purposes, In Proceedings of SCI2001, Orlando, USA,
July 22-25, 2001
Umbaugh, S., 1998, Computer Vision and Image
Processing, Prentice Hall
SIGMAP 2008 - International Conference on Signal Processing and Multimedia Applications
196