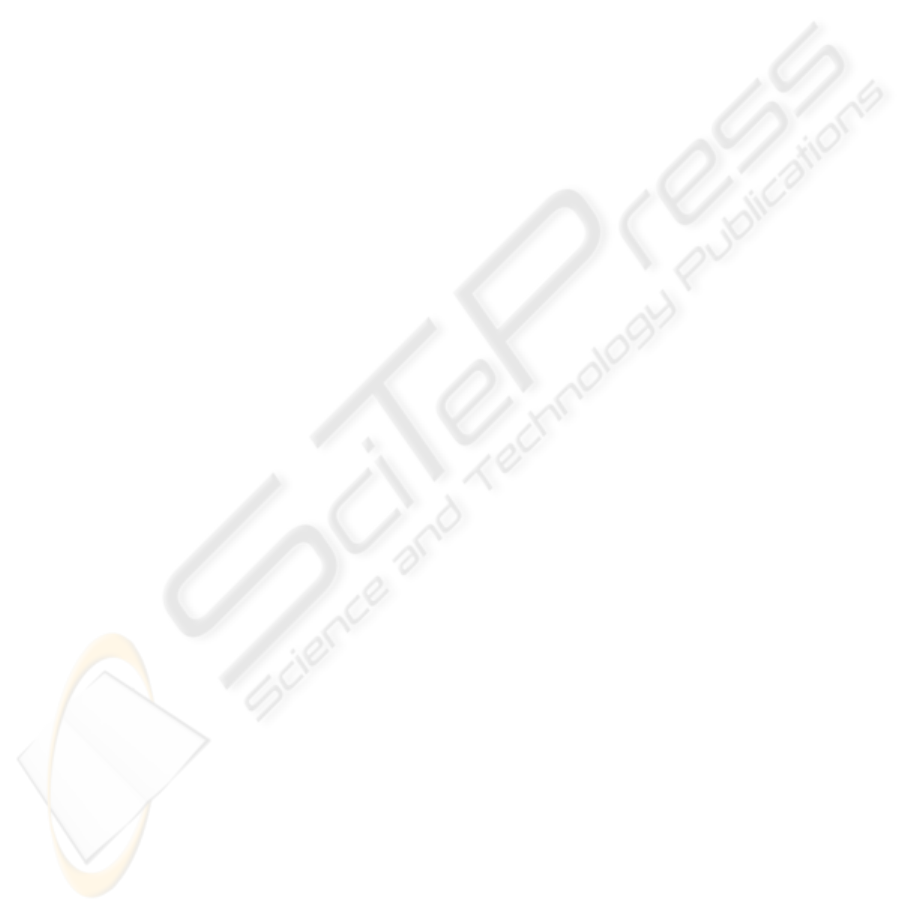
extended our affective educational system by
providing mobile interaction between the users and
handheld device. The system is based on mobile
technology and incorporates the quite recent theory
of Affective Computing.
In view of the above, in this paper we describe a
novel mobile educational system that incorporates
bi-modal emotion recognition. The proposed system
collects evidence from the two modes of interaction
and analyses them in terms of some attributes for
emotion recognition. Finally the system associates
the users’ input data through a multi-attribute model
and makes final assumptions about the user’s
emotional state. For the effective application of the
multi-attribute decision making model, we
conducted an empirical study with the participation
of human experts as well as possible users of the
system.
2 EMPIRICAL STUDY FOR
ATTRIBUTES
DETERMINATION
In order to collect evidence about which information
could be used for emotion recognition, we
conducted an empirical study.
2.1 Settings of the Experiment
The empirical study that we have conducted
concerns the audio-lingual emotion recognition, as
well as the recognition of emotions through
keyboard evidence. The audio-lingual mode of
interaction is based on using a mobile device’s
microphone as input device. The empirical study
aimed at identifying common user reactions that
express user feelings while they interact with mobile
devices. As a next step, we associated these
reactions with particular feelings.
Individuals’ behaviour while doing something
may be affected by several factors related to their
personality, age, experience, etc. Therefore, the
empirical study involved a total number of 100 male
and female users of various educational
backgrounds, ages and levels of familiarity with
computers.
The participants were asked to use a mobile
educational application, which incorporated a user
monitoring component. The user monitoring
component that we have used can be incorporated in
any application, since it works in the background
recording each user’s input actions. Part of the
interaction included knowledge tests, while
participants were asked to use oral interaction via
their mobile device’s microphone. Our aim was not
to test the participants’ knowledge skills, but to
record their oral and written behaviour. Thus, the
educational application incorporated the monitoring
module that was running unnoticeably in the
background. Moreover, users were also video-taped
while they interacted with the mobile application.
After completing the interaction with the
educational application, participants were asked to
watch the video clips concerning exclusively their
personal interaction and to determine in which
situations they where experiencing changes in their
emotional state.
As the next step, the collected transcripts were
given to 20 human expert-observers who were asked
to perform audio emotion recognition with regard to
the six emotional states, namely happiness, sadness,
surprise, anger, disgust and neutral. All human
expert-observers possessed a first and/or higher
degree in Psychology and, to analyze the data
corresponding to the audio-lingual input only, they
were asked to listen to the video tapes without
seeing them. They were also given what the user had
said in printed form from the computer audio
recorder. The human expert-observers were asked to
justify the recognition of an emotion by indicating
the weights of the attributes that they had used in
terms of specific words and exclamations, pitch of
voice and changes in the volume of speech.
2.2 Analysis of the Results
The analysis of the data collected by both the human
experts and the monitoring component, revealed
some statistical results that associated user input
actions through the mobile keyboard and
microphone with possible emotional states of the
users. More specifically, considering the keyboard
we have the following categories of user actions: a)
user types normally b) user types quickly (speed
higher than the usual speed of the particular user) c)
user types slowly (speed lower than the usual speed
of the particular user) d) user uses the “delete” key
of his/her mobile device often e) user presses
unrelated keys on the keyboard f) user does not use
the keyboard.
Considering the users’ basic input actions
through the mobile device’s microphone we have 7
cases: a) user speaks using strong language b) users
uses exclamations c) user speaks with a high voice
volume (higher than the average recorded level) d)
user speaks with a low voice volume (low than the
average recorded level) e) user speaks in a normal
voice volume f) user speaks words from a specific
list of words showing an emotion g) user does not
say anything.
Therefore, each moment the system records a
vector of input actions through the keyboard (k1, k2,
MULTI-ATTRIBUTE DECISION MAKING FOR AFFECTIVE BI-BIMODAL INTERACTION IN MOBILE DEVICES
377