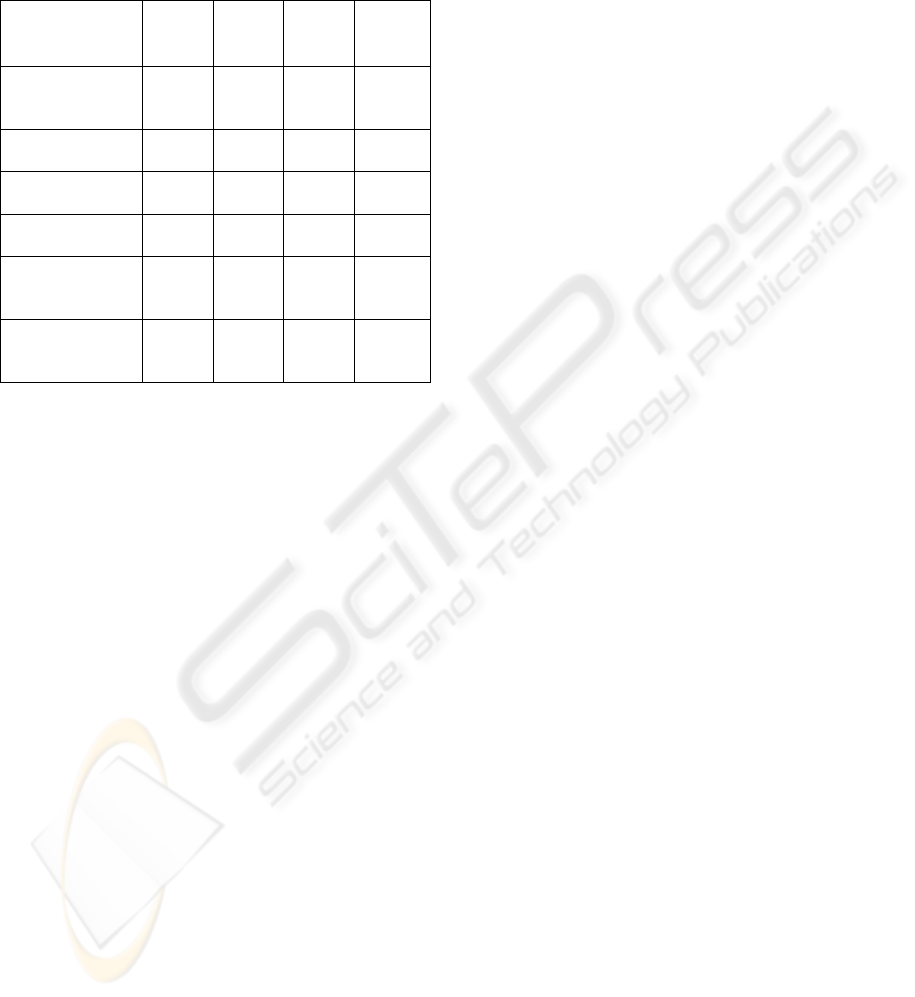
results show a significant improvement to the
classification accuracy obtained from combining the
new feature with the HZCRR, the LSTER, or both.
Table 2: Classification errors (percentage) with different
combinations of the three features using SVM and GMM.
As observed, the total errors introduced using the
three features are 4.69% and 3.17% with the SVM
and the GMM classifiers, respectively. To ensure the
effectiveness of the proposed features, evaluation of
the classification performance is extended to file-
level, in addition to the segment-level evaluation
(one-second window) described earlier. We made this
evaluation based on a majority voting strategy at file-
level. We used the same speech-music database in
this test and reached just 1.63% error, i.e. one speech
file out of 61 speech-music test files.
As shown in table 2, better classification results
are achieved over music files, as compared to speech,
when the
BDFV is used. Most sounds generated by
musical instruments have a harmonic structure,
which is not the case with speech signals that may
have a mixed harmonic/non-harmonic structure due
to their diverse voicing characteristics. This diversity
is well identified by the sinusoidal model that
measures the harmony of the audio signals.
Nevertheless, the BDFV feature of the sinusoidal
model plus the HZCRR and the LSTER form a
powerful feature set for speech/music discrimination.
Still, further performance improvement could be
expected to achieve by combining other features of
the sinusoidal model as an extension to this work.
6 CONCLUSIONS
In this study, we have proposed a new feature based
on the sinusoidal model, called BDFV, for audio
classification to speech and music. This feature is the
variance of the birth-death frequencies in the
sinusoidal model of an audio signal, as a measure of
the harmony. Our classification results show a high
discriminating performance of this feature, as
compared to typical features such as the HZCRR and
the LSTER features that are widely used for audio
classification. It is also revealed that a higher
classification performance is achieved, by combining
this new feature with the HZCRR and the LSTER,
which has been evaluated using the model-based,
insensitive to threshold GMM and the SVM
classifiers. Through this work, it has been shown that
the sinusoidal model features are very effective in
audio classification, due to capability of the model to
identify the harmonic structure.
REFERENCES
Ei-Maleh, K., Klein, M., Petrucci, G., kabal, P. 2000.
Speech/music discrimination for multimedia
Applications. In Proc ICASSP- 2000, pp. 2445-2448.
Ajmera, J., McCowan, I., Bourlard, H., 2002. Robust
HMM based speech/music segmentation. In Proc
ICASSP- 2002, pp. 297-300.
Saunders, J., 1996. Real-time discrimination of broadcast
speech/music. In Proc ICASSP-96, pp. 993-996.
Scheirer, E., Slaney, M., 1997. Construction and evaluation
of a robust multifeature speech/music discriminator. In
Proc. ICASSP- 97, pp. 21-24.
Lu, L., Zhang, H.-J., 2002. Content Analysis for Audio
Classification and Segmentation. In IEEE Trans.
Speech & Audio Proc., vol. 10, pp. 504 – 516.
Li, S. Z., 2000. Content-based audio classification and
retrieval using the nearest feature line method.In IEEE
Trans. Speech & Audio Proc., vol. 8, pp. 619 – 625.
McAulay, R., Quatieri, T., 1986. Speech analysis/synthesis
based on a Sinusoidal representation. In IEEE Trans.
Acous., Speech & Sig. Proc., Vol. ASSP-34, No.4, pp.
744-754.
Smith, J. O., Serra, X., 1987. PARSHL: An
analysis/synthesis program for non-harmonic sound
based on Sinusoidal representation. In http://www-
ccrma.stanford.edu/~jos/parshl/parshl.pdf.
Berenzweig, A. L., Ellis, D. P. W., 2001. Locating singing
voice segments within music signals. In Proc IEEE
WASPAA, Mohonk NY, pp. 119–122.
Guo, G., Li, S. Z., 2003. Content-based audio
classification and retrieval by support vector machines.
In IEEE Trans. Neural Networks Proc., vol. 14, pp.
209-215.
915 300 315 300
Total Length
(sec)
→
Total
Vocal
Music
Non-
Vocal
Music
Speech
Features/
Classifier
↓
12.13 10.66 15.87 9.66 HZCRR+
LSTER/SVM
5.46 0.66 2.53 13.33 HZCRR+
BDFV/SVM
4.91 0.33 2.22 12.33 LSTER+
BDFV/SVM
4.69
0 2.22 12 HZCRR+
LSTER+
BDFV/SVM
3.17
0.66 1.58 9.66 HZCRR+
LSTER+
BDFV/GMM
SIGMAP 2008 - International Conference on Signal Processing and Multimedia Applications
144