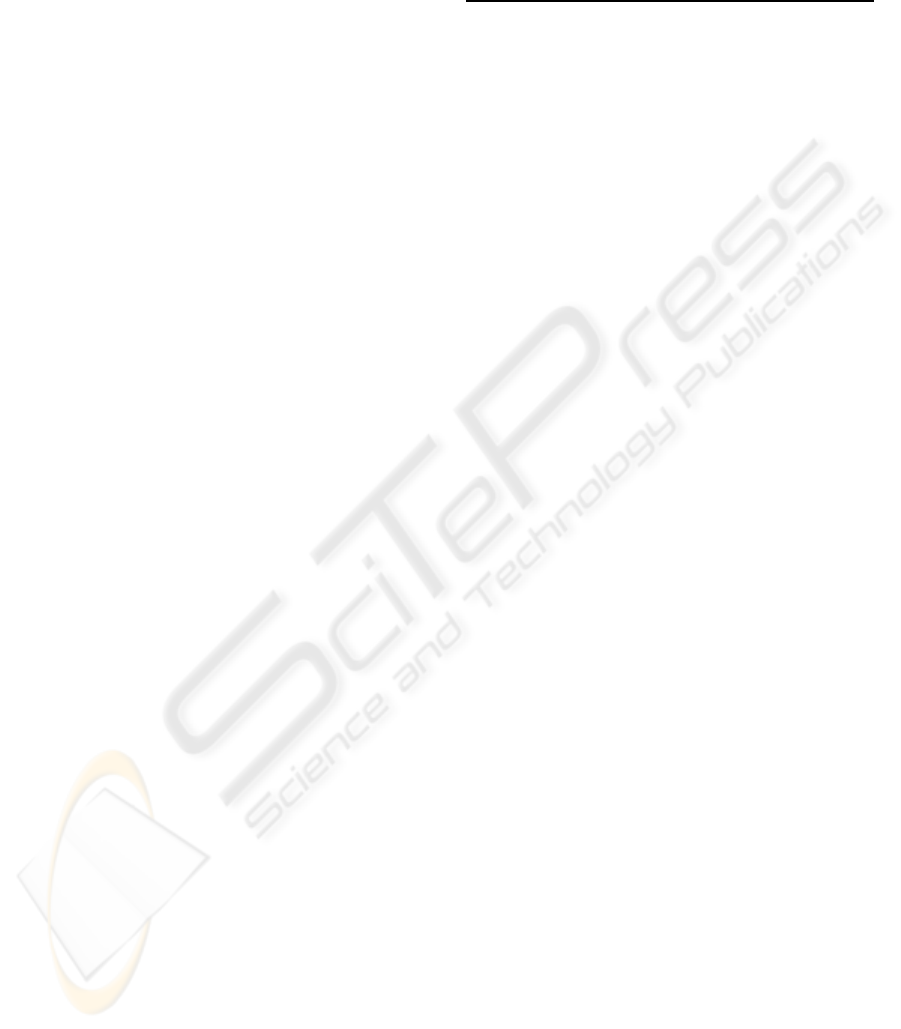
displays the most relevant ads according to the au-
dience stereotypes, while the individuals can receive
personalized offers in their mobile phones. In a given
moment, Alice presses a YELLOW button in her mo-
bile to indicate that she wants to learn more about
the product currently advertised in the bar’s TV, but
later (RED would mean that she is not at all inter-
ested in that stuff, while GREEN would mean that she
wants to learn more about the offer right now). Fol-
lowing this indication, once the first half of the game
is over, Alice’s mobile vibrates to face her with an in-
teractive application that lets her browse t-shirts of
different clubs, prices and so on. Alice could buy
one item using the application, but now she doesn’t
want to. However, thanks to the incentive schemes of
INCENTV-SPORTS, she can still benefit from provid-
ing some information about the offered products. The
more information, the greater the reward:
• By simply providing numerical ratings (from 0 to
10), she collects points that she may exchange for
backgrounds, screensavers and tunes for her mo-
bile phone.
• By tagging products with words from a given vo-
cabulary, she gets points to exchange for products
also advertised on INCENTV-SPORTS.
• By entering new tags to classify products, she wins
discounts to watch the next match at home (e.g.
e0.1 per tag, to a maximum of e2). Textual com-
ments would provide additional discounts of 1e.
Prior to sending it out, Alice can review the data
that will be sent to INCENTV-SPORTS, finding that
she can supplement what she entered with context in-
formation given by the bar owner (e.g. describing the
bar’s atmosphere or common likings of its clients).
Thus, the bar owner can also get rewards, propor-
tional to the amount of feedback gathered from his
clients and the amount of products they buy. In fact,
next week he will be able to offer a new match for free.
On Friday, the INCENTV-SPORTS managersstart
processing all the feedback gathered from the football
match. Firstly, it is noticeable that there has been very
little activity around tourist destinations ads. This in-
dicates that a football match is not a suitable place to
advertise travels, so the stereotype “people who like
travelling” loses relevance with regard to football. In
contrast, there has been much activity around sports
stuff, measuring great disparity in the viewers’ reac-
tions to certain items that fitted well within the stereo-
type of “people who like football”. In response to
these observations, an analyst suggests to specialize
the stereotype, introducing subclasses of “supporters
of A.C. Milan” and “supporters of Inter Milan”. As
a result, during the next Champions League round,
the supporters of A.C. Milan are not faced with mer-
chandising of Inter Milan and vice versa. Everyone
is happier with the publicity received and an increase
occurs in the sales figures.
5 CONCLUSIONS
With the growing amount of contents available
through different media, personalized information
services face a risk of starvation due to lack of meta-
data to reason about. In order to solve this problem,
we have argued that it is necessary to turn the users
into active providers of metadata, for which they must
be given suitable incentives in a social network. Real-
izing this view will require much research in incentive
schemes, business models, user interfaces, knowledge
modeling and data mining techniques, among others.
ACKNOWLEDGEMENTS
The visions presented herein will drive the authors’
research within the Ministerio de Educación y Cien-
cia (Gobierno de España) project TSI2007-61599,
also supported by the Consellería de Educación e Or-
denación Universitaria (Xunta de Galicia) incentives
file 2007/000016-0, and by the Consellería de In-
novación, Industria e Comercio (Xunta de Galicia)
project PGIDIT05PXIC32204PN.
REFERENCES
Adomavicius, G. and Tuzhilin, A. (2005). Towards the
next generation of recommender systems: A survey
of the state-of-the-art and possible extensions. IEEE
Transactions on Knowledge and Data Engineering,
17(6):739–749.
Ardissono, L., Gena, C., Torasso, P., Bellifemine, F.,
Chiarotto, A., Difino, A., and Negro, B. (2004). User
modeling and recommendation techniques for person-
alized Electronic Program Guides. In Personalized
Digital Television. Targeting programs to individual
users. Kluwer Academic Publishers.
Burke, R. (2002). Hybrid recommender systems: Survey
and experiments. User Modeling and User-Adapted
Interaction, 12(4):331–370.
Cao, Y. and Li, Y. (2007). An intelligent fuzzy-based rec-
ommendation system for consumer electronic prod-
ucts. Expert Systems with Applications, 33(1):230–
240.
Chorianopoulos, K. (2008). Personalized and mobile digital
TV applications. Multimedia Tools & Applications,
36(1):1–10.
SIGMAP 2008 - International Conference on Signal Processing and Multimedia Applications
334