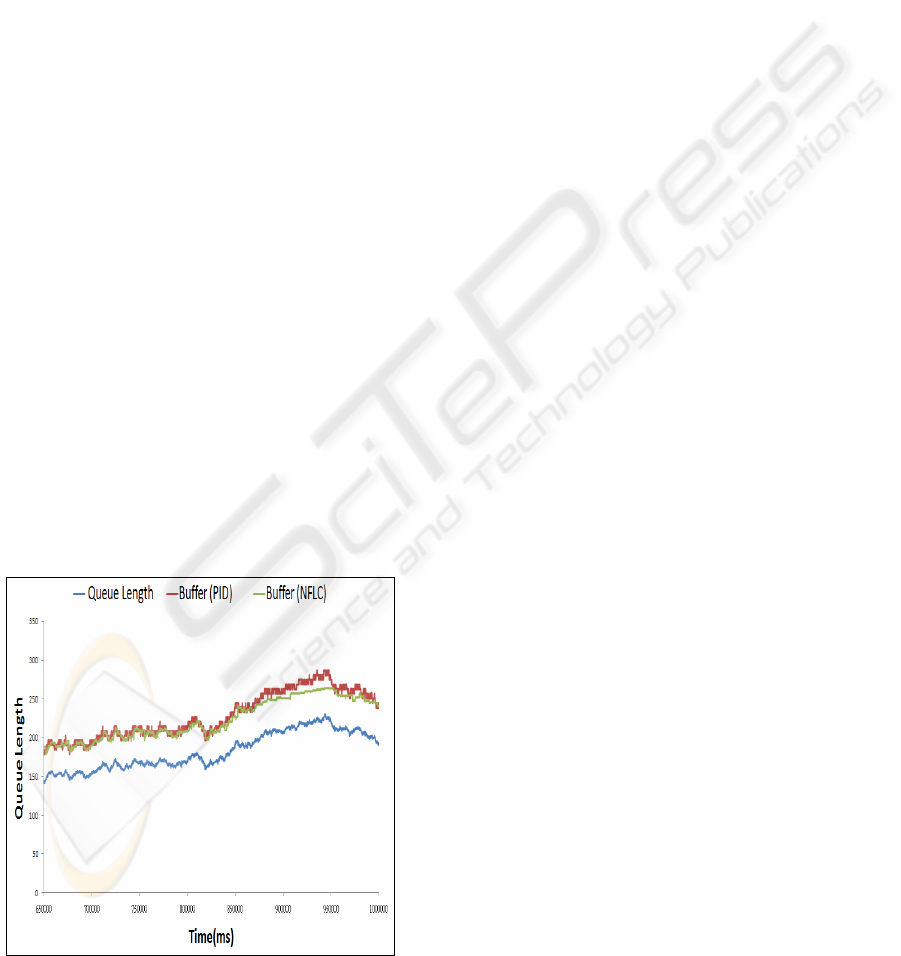
among the requests to the surrogate SAP. These
waveforms verified that the “NFLC + T-WTS”
combination indeed worked stably in known traffic
conditions. In the second category wireless traces
collected in the Hong Kong Polytechnic University
(Lin) were used to verify that the same combination
indeed worked for real situations. In all simulations
the same waveform would simultaneously excite the
two dynamic buffer tuners running in parallel: PIDC
and NFLC. The aim was to compare the results by
NFLC and PIDC under the same traffic conditions.
The comparison should confirm if the NFLC was the
right choice to yield a faster T-WTS response. Many
simulations were conducted: i) T-WTS with no
dynamic buffer tuner support; (ii) T-WTS with
NFLC, and iii) T-WTS with PIDC. The preliminary
results indicate that NFLC converges faster to the
steady state reference than PIDC. There was less
oscillation in the control process as well. When
dynamic buffer tuning was absent the T-WTS
produced frequent surrogate buffer overflows.
Figure 5 shows how NFLC rectified the PIDC
problem, which was locking too much buffer
memory even when it was no longer needed. This
PIDC problem lowered T-WTS performance
because it deprived other T-WTS tasks of needed
memory. The NFLC achieved this by rigorously
maintaining the given safety margin
Δ
between the
buffer length and the queue length on the fly. Surely,
the NFLC is a more accurate, smoother, faster, and
usable dynamic buffer tuner than PIDC. The benefit
of settling quickly to the steady state is less or no
buffer overflow and thus shorter roundtrip time
(RTT) (i.e. quicker system response).
Figure 5: NFLC unlocked unused buffer space.
5 CONCLUSIONS
The NFLC (Neuro-Fuzzy Logic Controller) is
proposed to yield shorter T-WTS response. This is
achieved for NFLC produces more dependable
client/server interaction over an end-to-end channel.
This advantage becomes obvious if NFLC
performance is compared to the PIDC’s. TCM
physicians in a smart space need to hook onto the
dedicated surrogate to conduct pervasive medical
consultations. The response, however, can be
seriously affected by the transient mass through the
smart space. This mass can create unpredictable
traffic volume and pattern for the merged traffic that
enters the queue of the dedicated surrogate. If
dynamic buffer tuning is absent, the merge traffic
could surge the queue to overflow the surrogate
buffer. If this happens, the clients in the smart space
would suffer from long service RTT and their
chance to benefit from the cyber foraging in the
pervasive computing infrastructure. The NFLC
prevents surrogate buffer overflow by ensuring that
the buffer always cover the queue by the given
safety margin. This makes the channel
dependable, as confirmed by the simulation results.
The focus of this research is to explore if the
proposed NFLC can indeed prevent user-level buffer
overflow effectively. But, we analyzed the
simulation results as well for any possible
correlation between traffic patterns and NFLC
accuracy. Our analysis indicated that such a
correlation exists. Internet traffic aggregates (time
series) can be stationary or chaotic (unstable), and
stationary traffic is either SRD (short-range-
dependence) or LRD (long-range dependence). SRD
includes Markovian traffic time series and LRD has
self-similar and heavy-tailed patterns. As observed
from the “T-WTS + NFLC” simulations, each traffic
pattern could produce distinctive ill effect on NFLC
control accuracy and convergence smoothness to the
steady state. Therefore, the next logical step for the
research is to explore and establish the correlation
between traffic patterns and their ill effects (e.g.
oscillatory convergence).
ACKNOWLEDGEMENTS
The authors thank the Hong Kong Polytechnic
University for the A-PA9H research grant.
APPLYING NEURO-FUZZY DYNAMIC BUFFER TUNING TO MAKE WEB-BASED TELEMEDICINE
SUCCESSFUL
155