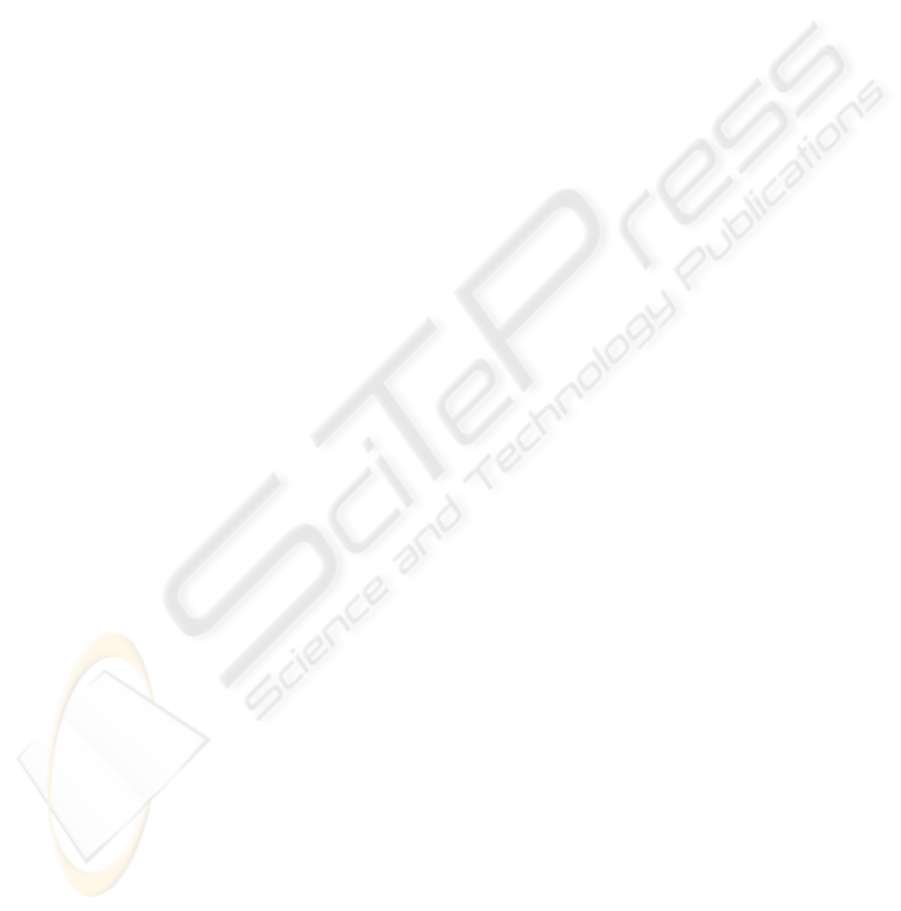
5 Conclusions
We presented two strategies for automatic image annotation that build on existing knowl-
edge from labeled image databases, so called visual folksonomies. On the one hand, we
implemented a supervised learning approach for classification using a SVM and a con-
trolled vocabulary. On the other hand, we presented a tag propagation system that uses
content-based image retrieval for automatic annotation that works with an uncontrolled
folksonomic vocabulary. The proposed approaches use state-of-the-art image features
and compute tags in less than 1 second per image which enables real-world applications
on top of large-scale image databases.
Experiments on a set of images from Flickr’s pool Fruit&Veg show that classifi-
cation works with a high precision of 71% on a limited set of target concepts. The
behaviour of the tag propagation approach heavily depends on the amount of propa-
gated tags per image. When only few tags are propagated, high precision but low recall
is achieved, while propagating many tags leads to low precision and high recall.
Although the classification setup presented in this work only implements the pre-
diction of one concept per image, it is planned to extend the approach and learn several
binary classifier for each concept individually for annotating one image with multiple
concepts. Future work might include the automatic definition of concepts and experi-
ments using more general and larger visual folksonomies.
Acknowledgements
The authors would like to thank their colleagues Marcus Thaler and Werner Haas for
their support and feedback. The Know-Center is funded by the Austrian Competence
Center program Kplus under the auspices of the Austrian Ministry of Transport, Innova-
tion and Technology (http://www.ffg.at), by the State of Styria and by the City of Graz.
The research leading to this paper was partially supported by the European Commis-
sion under contract FP6-027026 (K-Space), FP6-027122 (SALERO) and FP6-045032
(SEMEDIA).
References
1. I.T. Young, L.J. van Vliet, M. van Ginkel: Recursive Gabor Filtering. ICPR ’00: Proceedings
of the Int. Conf. on Pattern Recognition, Vol. 50, Issue 11, Nov 2002, 2798 - 2805
2. D. Forsyth, J. Ponce.: Computer Vision: A Modern Approach. Prentice Hall, 2002.
3. Datta, Ritendra, Li, Jia, Wang, James Z.: Content-Based Image Retrieval - Approaches and
Trends of the New Age. MIR ’05: Proc. of the 7th ACM SIGMM International Workshop on
Multimedia Information Retrieval, 2005
4. A.W.M. Smeulders, M. Worring, S. Santini, A. Gupta, R. Jain: Content-Based Image Re-
trieval at the End of the Early Years. IEEE Transactions on Pattern Analysis and Machine
Intelligence, 2000
5. A. Yavlinsky, E. Schofield, S. M. R
¨
uger: Automated Image Annotation Using Global Fea-
tures and Robust Nonparametric Density Estimation. Proc. of the 4th Int. Conf. on Image
and Video Retrieval (CIVR), Vol. 3568, 507-517, July 2005
6565