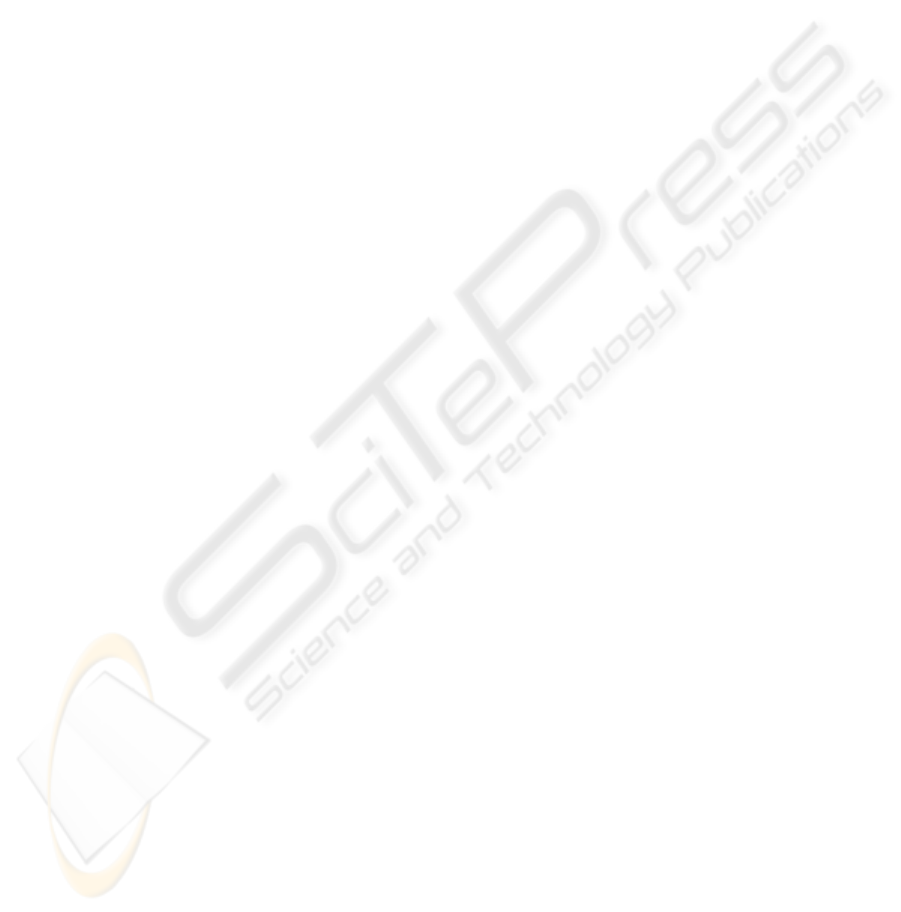
7. N. Poh, S. Bengio, How do correlation and variance of base-experts affect fusion in biomet-
ric authentication tasks?, IEEE Transactions on Acoustics, Speech, and Signal Processing,
vol. 53, 2005, pp. 4384–4396.
8. R. Zhao, W. I. Grosky, Narrowing the semantic gap - improved text-based web document
retrieval using visual features, IEEE Trans. on Multimedia, 4(2), 2002, 189–200.
9. S. C. Dass, A. K. Jain, K. Nandakumar, A principled approach to score level fusion in multi-
modal biometric systems, Proc. of Audio- and Video-based Biometric Person Authentication
(AVBPA), 2005, pp. 1049–1058.
10. S. Wu, S. McClean, Performance prediction of data fusion for information retrieval, Infor-
mation Processing and Management, 42, 2006, 899–915.
11. D. M. Squire, W. Mller, H. Mller, and J. Raki, Content-based query of image databases,
inspirations from text retrieval: inverted files, frequency-based weights and relevance feed-
back, in the 10th Scandinavian Conference on Image Analysis (SCIA’99), (Kangerlussuaq,
Greenland), 1999, pp. 143–149.
12. T. Kolenda, O. Winther, L.K. Hansen, J. Larsen, Independent component analysis for under-
standing multimedia content, Neural Networks for Signal Processing, 2002, 757– 766.
13. T. Westerveld, A. P. de Vries, Multimedia retrieval using multiple examples, In International
Conference on Image and Video Retrieval (CIVR’04), 2004, 344-352.
14. Y. Wu, K. Chen-Chuan Chang, E. Y. Chang and J. R. Smith, Optimal multimodal fusion for
multimedia data analysis, MULTIMEDIA ’04: Proc. of the 12th annual ACM international
conference on Multimedia, ACM Press, 2004, pp. 572–579.
15. R. Yan, A. G. Hauptmann, The combination limit in multimedia retrieval, MULTIMEDIA
’03: Proceedings of the eleventh ACM international conference on Multimedia, ACM Press,
2003, pp. 339–342.
16. A. Vinokurow, D.R. Hardoon and J. Shawe-Taylor, Learning the Semantics of Multime-
dia Content with Application to Web Image Retrieval and Classification, in Proceedings
of Fourth International Symposium on Independent Component Analysis and Blind Source
Separation, Nara, Japan, 2003.
17. A. Jakulin, I. Bratko, Quantifying and Visualizing Attribute Interactions, ArXiv Computer
Science e-prints, Provided by the Smithsonian/NASA Astrophysics Data System, 2003.
18. A. Jakulin, I. Bratko, Analyzing Attribute Dependencies, Proc. of Principles of Knowledge
Discovery in Data (PKDD), 2838, 2003, 229–240.
19. A. Hyvarinen, E. Oja, Independent Component Analysis: Algorithms and Applications, Neu-
ral Networks, 2000, 13(4-5),pp. 411-430.
20. N. Vasconcelos, G. Carneiro, What is the Role of Independence for Visual Recognition?,
European Conference on Computer Vision, Copenhagen, 2002, 297 - 311.
21. I. Nemenman, Information theory, multivariate dependence and genetic networks, eprint
arXiv:q-bio/0406015, ARXIV, 2004.
22. M.J. Pazzani, Searching for Dependencies in Bayes Classifiers, 1996, Learning from Data:
AI and Statistics, Springer Verlag.
23. A.J. Bell, The Co-Information Lattice, 4th Int. Symposium on Independent Component Anal-
ysis and blind Signal Seperation (ICA2003), 2003, pp. 921–926.
24. T. Westerveld and R. van Zwol, Multimedia Retrieval at INEX 2006, 2007, ACM SIGIR
Forum, 41(1), pp. 58-63.
25. I. Kononenko, E. Simec and M. Robnik-Sikonja, Overcoming the myopia of inductive learn-
ing algorithms with RELIEFF, Applied Intelligence, 7(1), 1997, pp. 39-55, Springer Nether-
lands.
26. I. Perez, Learning in presence of complex attribute interactions: An Approach Based on
Relational Operators, PhD dissertation, University of Illinois at Urbana-Champaign, 1997.
3232