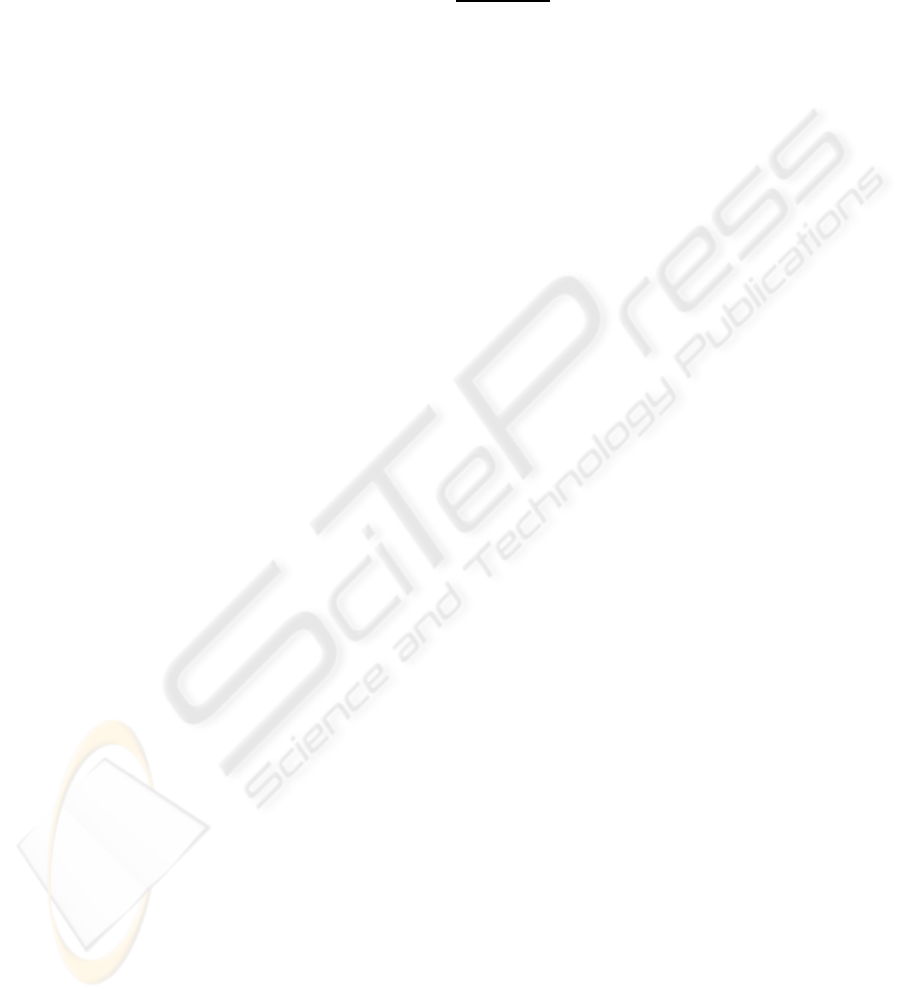
direction r, divided by the average speed of the target previously measured in the last f
frames:
j = kC
v
− E
r
k/
P
f
i=1
step
i
f
!
(3)
where step
i
represents the distance covered by the target between frames i and i − 1.
4 Results and Conclusions
The automatic target search problem has been faced in a general real case study for
video surveillance application to control unauthorized access in restricted access areas.
The videos were acquired using a thermocamera in the 8 − 12µ
m
wavelength range,
mounted on a moving structure covering 360
◦
pan and 90
◦
tilt, and equipped with 12
◦
and 24
◦
optics to have 320 × 240 pixel spatial resolution.
The database for the CBIR was built taking into account different image sequences
relative to different classes of the monitored scenes. In particular, the human class has
been composed taking into account three different postures (i.e. upstanding, crouched,
crawling) considering three different people typologies (short, medium, tall).
The estimated number of operations performed for each frame when tracking per-
sons consists of about 5 · 10
5
operations for the identification and characterization
phases, while the active tracking requires about 4 · 10
3
operations. This assures the
real time functioning of the procedure on a personal computer of medium power. The
automatic search process can require a higher number of operations, but it is performed
when the target is partially occluded or lost due to some obstacles, so it can be reason-
able to spend more time in finding it, thus loosing some frames. Of course, the number
of operations depends on the relative dimension of the target to be followed, i.e. bigger
targets require a higher effort to be segmented and characterized. The acquired images
are pre-processed to reduce the noise. In Figure 3 example frames of video sequences
are shown (top) together with their relative shape extraction.
While in Figure 4 the same frames are processed, and shape contour with distribu-
tion histogram of the normal are shown.
A methodology has been proposed for the Content Based Image Retrieval problem
in a video-surveillance task, when the tracking target is lost or occluded, and needs to
be grabbed back again. Target recognition during active tracking has been performed,
using a Hierarchical Artificial Neural Network (HANN). In case of automatic search-
ing of a masked or occluded target, a Content-Based Image Retrieval paradigm has been
used for the retrieval and comparison of the currently extracted features with the previ-
ously stored in a reference database. The reference database has been implemented and
populated following an approach based on semantic categorization of the information.
Acknowledgements
This work was partially supported by EU NoE MUSCLE - FP6-507752. We would
like to thank Eng. M. Benvenuti, head of the R&D Dept. at TDGroup S.p.A., Pisa, for
allowing the use of proprietary instrumentation for test purposes.
119119