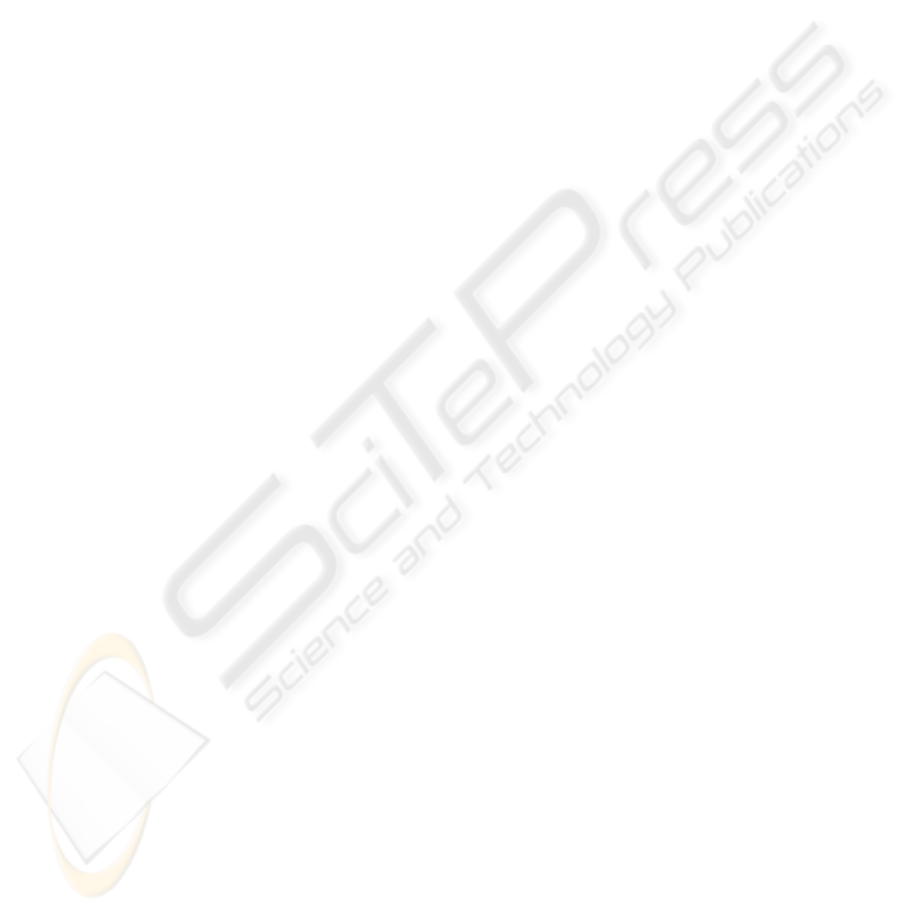
Two main typologies of image mining frameworks can be individuated: function-
driven, which are focused on the functionalities of the components to be integrated, and
information-driven, which are designed as a hierarchical structure with special empha-
sis on the information needs at various levels of the hierarchy.
Within the medical field, some image mining systems have been presented [13], es-
pecially within histology and cytology [3], [4], [10]; however the main focus of their
development has been reserved to the problem of image retrieval by content, thus miss-
ing the real interesting challenge of extracting new knowledge. Exploiting experiences
matured within the EU Network of Excellence MUSCLE (Multimedia Understand-
ing through Semantics, Understanding and Learning), the STREP EU project HEART-
FAID (A knowledge based platform of services for supportingmedical-clinical manage-
ment of the heart failure within the elderly population) and the Italian-Russian bilateral
project, we are working on the development of an Image Mining Medical Warehouse
(IMMW) which is meant to supply all the image mining functionalities, ranging from
image storage to novel knowledge discovery. IMMW has been designed by integrating
and extending the infrastructure we have developed within the MUSCLE initiative for
managing multimedia metadata (the so-called 4M infrastructure) [1] by integrating a
semantic apparatus for suggesting suitable image analysis algorithms and appropriate
decisions on medical diagnosis.
In this paper, current results of the design activity are presented since interesting
aspects have come forth as worthy of discussion. The main components of IMMW are
also introduced and future activities discussed.
2 The Infrastructure for an Image Mining Medical Warehouse
IMMW infrastructure has been conceived for aiding the investigation and diagnostic
processes of medical problems, by providing local and remote access to known cases,
the facility for retrievingimages by content, and the possibility of mining image patterns
relevant to medical decision making, e.g., diagnoses and prognoses.
Several practical scenarios can be supplied for highlighting the usefulness of IMMW
functionalities:
· Case-based reasoning: a physician is presented with magnetic resonance imaging
(MRI) data of a patient’s brain, but imaged patterns raise some uncertainty in the
diagnosis. Retrieving similar cases according to patient’s information and the im-
agery data can help him making a final decision by investigating other physicians’
diagnoses;
· Exploration of concurrentsituations: a histopathologist researcher is experimenting
with a new marker on breast tissue. For comparing its performance, he can search
for the effects of the same marker on different tissues, e.g., on liver tissue, or for
other markers resulting in the same effects on breast tissue;
· Extraction of new knowledge: a cardiologist wants to explore the prognostic value
of some parameters extracted from imaging data, e.g., of the ejection fraction com-
puted from cardiac MRI. Applying data mining techniques on opportunely defined
patterns, he can find out the relationship between prognosis and the set of parame-
ters.
8484