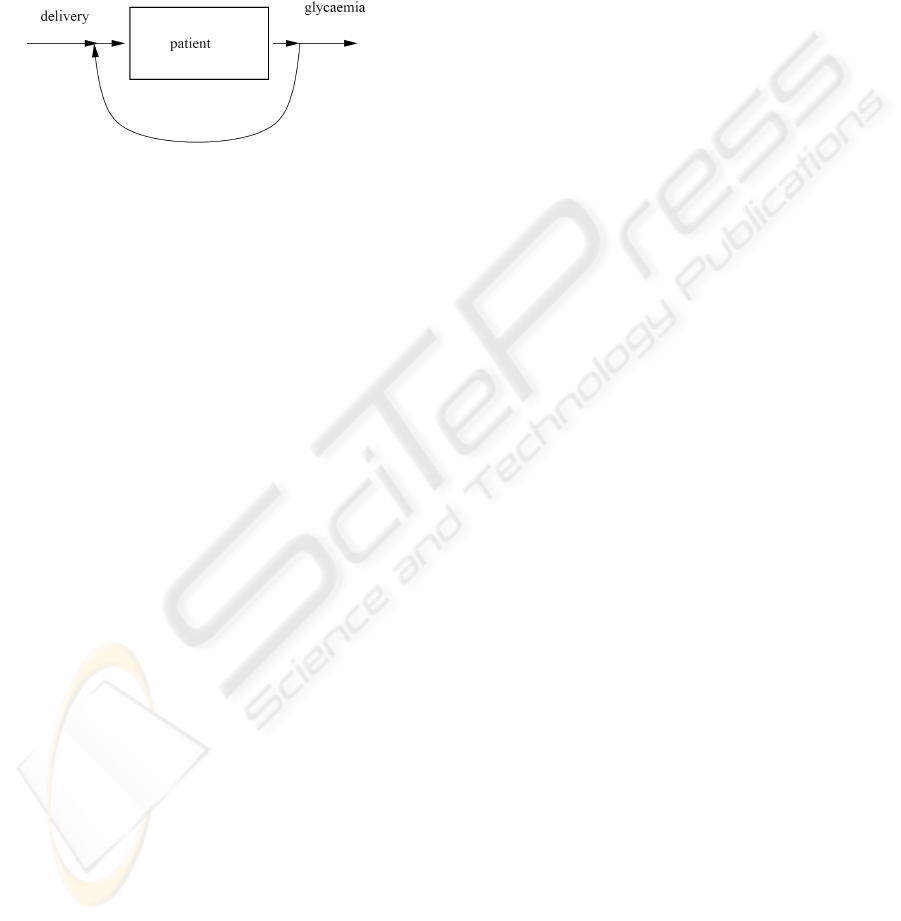
Then we proposed a bilinear modeling giving a
good approximation of the behavior “insulin deliv-
ery/glycaemia” on an interval of 15 minutes, in stan-
dard conditions (C. Hespel, 2000).
Once the model is known, the regulation consists
in inverting the input/output behavior of the system
(M. V. Foursov, 2003; M. V. Foursov, 2004). One has
to calculate the input in terms of the output function
one wishes to obtain (Fig.1). This regulation is par-
Figure 1: Regulation.
tially closed-loop because the glycaemic values are
only used every 15 minutes in order to compute the in-
sulin delivery. On constant intervals of time [t
i
,t
i+1
]
i
,
we compute some model M
i
and function insulin de-
livery u
i
(t) in order to follow an ideal trajectory y
i
(t)
for the glycaemia. On every time interval, the trajec-
tory is recalculed because of the variation between the
ideal trajectory and the true trajectory.
The crucial point consists in determining the size of
the time intervals, i.e. the frequency of the changes of
the insulin delivery. The study of the stability leads
us to reduce the size of the interval when the system
is unstable.
2 PRELIMINARIES: MODELING,
REGULATION
Two techniques are used to achieve this goal:
Phenomenological modeling requires a knowledge of
the equations governing the evolution of the process.
Such models of the glycaemic behavior of diabetics
were developed (Albisser, ).
In the behavioral modeling, the system is regarded as
a black box (J. Sjoberg, 1995). The goal is to con-
struct a model that approximates the system with a
desired precision (Chen and Chen, ). The parame-
ters involved in the obtained system of equations have
no practical significance, but their number depends on
the required precision.
A commonly-used class of models is formed by linear
dynamical systems. Linear-model-based regulation is
simple to implement and it gives quite satisfactory re-
sults in many cases. It did not seem to be sufficient
for regulating the glycaemia of diabetics.
Another class of models consists of bilinear systems.
A bilinear system is quite similar to a linear one: it is
additionally linear as a function of the input. One has
thus more leeway to approximate the real system with
a better precision. We choose to model the system by
a bilinear system whose dimension is not fixed.
Since a diabetic does not respond in the same way to
equal doses of insulin at different times of the day, we
suppose that she is described by different systems at
different time instants. So we construct a collection
of models that describe the behavior of the glycaemia
under certain conditions. Each model is thus valid
only for a certain period of time (at least 15 minutes).
Mathematically, the problem is to identify locally
(near t
0
) up to a given order k, a single input sys-
tem with drift considered as a black box, when only a
sample of the input/output data is known. Our method
involves the identification up to order k of the gener-
ating series G of the unknown system and the con-
struction of a bilinear system (B
k
) approximating the
unknown system up to k.
An advantage of this method is the possibility to pro-
vide, in terms of the order k, a system (B
k
) approxi-
mating the unknown system. (B
k
) is chosen so that its
output and the output of the unknown system coincide
up to k. The problem of identification of dynamical
systems in a neighborhood of t
0
is the following: to
determine the generating series of the unknown sys-
tem, up to a given order k, given the Taylor series of
the input and corresponding output functions.
The identification involves the following 3 steps. Dur-
ing the first step, one obtains a system of linear equa-
tions that express the relationship between the deriva-
tives of the input and the output. The unknown pa-
rameters are certain linear combinations of the coeffi-
cients of the generating series. On the next step, these
linear combinations of the coefficients are identified
from the available data, by choosing appropriate in-
put/output sets. Finally, the coefficients are identified
by solving another system of linear equations.
A bilinear system corresponding to a rational series of
minimal rank is constructed providing a local model.
2.1 The Bilinear Model
A bilinear system (B) with a single input u
1
(t) and a
drift u
0
(t) ≡ 1 is given by its state equations
(B)
x
(1)
(t) = (M
0
+ u
1
(t)M
1
)x(t)
y(t) = λ.x(t)
(1)
x(t) ∈ an R−vector space Q, M
0
, M
1
, λ are R−linear.
We consider the alphabet Z = {z
0
, z
1
}, where z
0
codes
the drift and z
1
codes the input. The expansion of the
generating series G built on Z, by noting w a word
∈ Z
∗
, is G =
∑
w∈Z
∗
hG|wiw.
BIODEVICES 2009 - International Conference on Biomedical Electronics and Devices
336