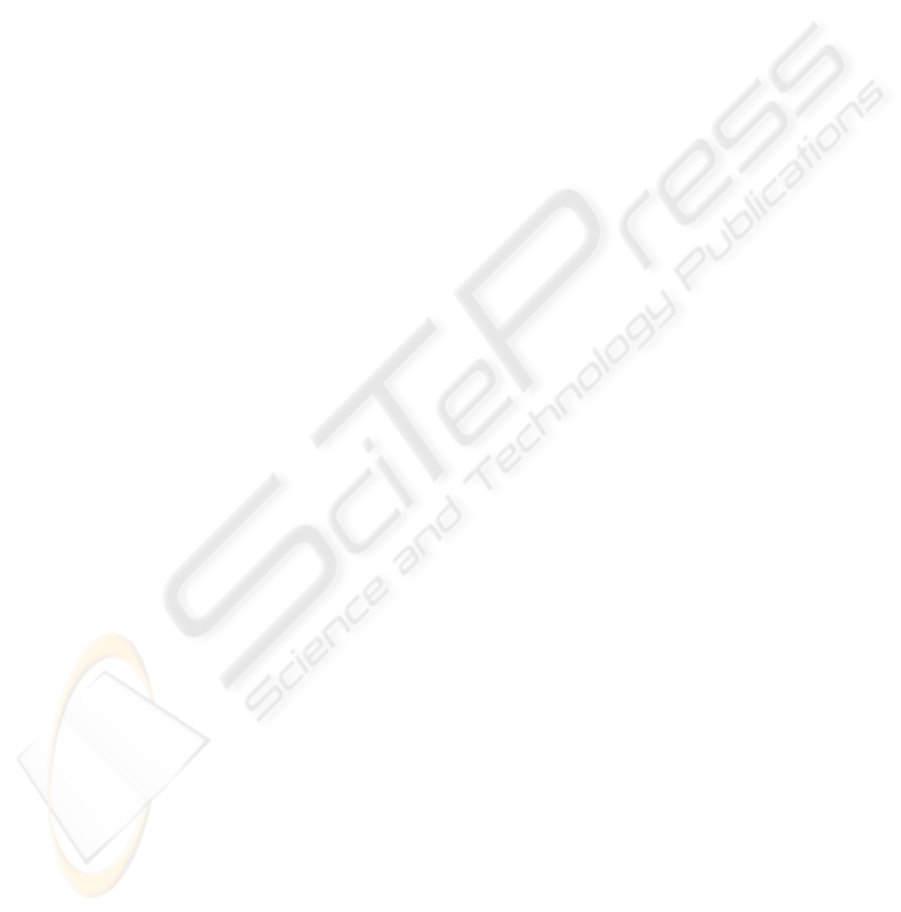
different database. Therefore, in the table 2 is
showed the number of writer and its success. For
this present work has been obtained a better success
rate with more writers. In the future works, we are
working on the increase of our database and the
creation of novel and discriminative parameter.
6 CONCLUSIONS
For Graphologist or Forensic Experts, the combined
features (classical and novel graphologist
parameters) contribute more reliable information in
order to identify a person.
Therefore in this work, we have developed a
combined parameterization between classical and
novel graphologist characteristics, in order to be
used in the writer identification from handwriting
documents. In fact, the use of these novel parameters
has improved the classical system.
We have used a back-propagation NN for the
classification. And in order to improve results, we
are implemented a ‘more voted’ algorithm. The
success rate is 96% for 70 writers.
The experiments carried out with our database to
evaluate the power of discrimination of the
implemented characteristics, it is allowing us to
show the considerable increase of the success rate
with all the developed characteristics.
ACKNOWLEDGEMENTS
This present work has been supported by Education
and Science Ministry from Spanish Government
(TEC2006-13141-C03-01).
REFERENCES
Otsu, N., 1979. A threshold selection method from gray-
level histograms. In IEEE Transaction on Systems,
Man and Cybernetics, Vol. 9, Issue 1, pp 62-66.
Gupta, S., Namboodiri, A.M., 2007. Repudiation
Detection in Handwritten Documents. In Lecture
Notes in Computer Science. Vol. 4642, pp. 356-365.
Leedham, G., Chachra, S., 2003. Writer Identification
using Innovate Binarised Features of Handwritten
Numerals. In Proceeding of the 7th International
Conference on Document Analysis and Recognition.
Vol. 1, pp. 413-416.
Romero, C.F., Travieso, C.M., Alonso, J.B., Ferrer, M.A.,
2007. Using Off-line Handwritten Text for Writer
Identification. In WSEAS Transactions on Signal
Processing. Issue 1, Vol. 3, pp. 56-61.
Hertel, C., Bunke, H., 2003. A Set of Novel Features for
Writer Identification. In Proceedings of the Audio and
Video Based Biometric Person Authentication. pp.
679-687.
Chin, W., Harvey M., Jennings, A., 1997. Skew Detection
in Handwritten Scripts. In IEEE Region 10 Annual
Conference. Speech and Image Technologies for
Computing and Telecommunications. Vol. 1, p. 319-
322.
Bishop, C.B., 1995. Neural Networks for Pattern
Recognition, Oxford University Press.
Juang, B.H., Rabiner, L.R., 1992. Spectral representations
for speech recognition by neural networks-a tutorial.
In Proceedings of the Proceedings of the Workshop
Neural Networks for Signal Processing. pp. 214 – 222.
Said, H.E.S., Peake, G.S., Tan, T.N., Baker, K.D., 1998.
Writer Identification from Non-uniformly Skewed
Handwriting Images. In Proceedings of the 9th British
Machine Vision Conference. pp. 478-487.
Zois, E.N., Anastassopoulus, V., 2000. Morphological
Waveform Coding for Writer Identification. In Pattern
Recognition. Vol. 33, Nº3, pp. 385-398.
Marti, U.V., Messerli, R., Bunke, H., 2001. Writer
Identification Using Text Line Based Features. In
Sixth International Conference on Document Analysis
and Recognition, pp. 101-105.
Srihari, S., Cha, S.H., Arora, H., Lee, S., 2001.
Individuality of Handwriting: A Validity Study. In
Proceedings ICDAR’01. pp 106-109.
Bensefia, A., Pasquet, T., Heutte, L., 2005. Handwritten
Document Analysis for Automatic Writer
Recognition. In Electronic Letters on Computer Vision
and Image Analysis. pp. 72-86.
Schomaker, L., Bulacu, M., 2004. Automatic Writer
Identification Using Connected-Component Contours
and Edge-Based Features of Uppercase Western
Script. In IEEE Transactions on Pattern Analysis and
Machine Intelligence. Vol. 26, No. 6, pp. 787 – 798.
Romero, C.F., Travieso, C.M., Alonso, J.B., Ferrer, M.A.,
2006. Writer Identification by Handwritten Text
Analysis. In Proceeding of the 5th WSEAS int. Conf.
on System Science and Simulation in Engineering. pp.
204-208.
BIOSIGNALS 2009 - International Conference on Bio-inspired Systems and Signal Processing
454