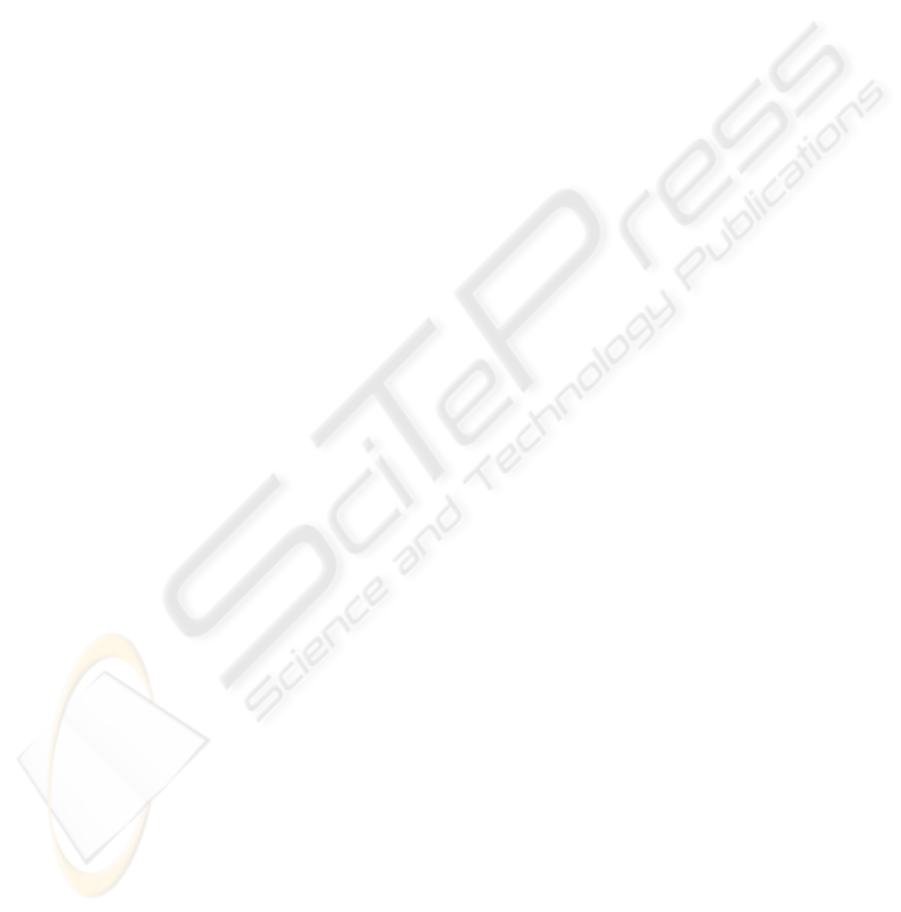
5 CONCLUSIONS
This paper evaluates the use of two set of features
(PSD components and AAR/RLS parameters of an
EEG signal) as inputs for a SVM classifier, in order to
distinguish between two mental tasks related to hands
motor imagery.
The approach based on PSD (Welch’s Method)
components and a SVM (RBF kernel) generated the
best results. The highest classification rates are re-
lated to the middle of the experiment, usually be-
tween seconds 4 and 6. It can be explained taking
into account that the subject needs some time to setup
him/herself (the cue, an arrow, is presented to the sub-
ject at instant t = 3 s) to the end of the trial (the trial
finishes at t = 9 s).
The best results can be accomplished using only
2 channels, four electrodes placed around positions
[C
3
C
4
] of the 10-20 International System.
After evaluating the system with the UAH dataset,
the algorithms were applied to the Graz dataset and
the best classification rate (accuracy) was 97.1%
(99.4% to mental task 1 and 93.5% to mental task 2).
The replacement of the method currently used to
select a symbol in a PDA, which requires a brief eye-
closing, by another based on motor imagery, such as
the one here discussed, is the next step of our research.
In other words, the idea is that motor imagery of a
hand (the one with higher accuracy) it is enough to
the user without eyes control to select desired symbols
in the PDA that will be translated into commands to
the robotic wheelchair or into some communication
outputs, also available in this system.
ACKNOWLEDGEMENTS
The authors thank CAPES, a foundation of the
Brazilian Ministry of Education (Project 150/07),
and FACITEC/PMV, a fund of the Vitoria City Hall
to support scientific and technological development
(Process 061/2007), for their financial support to this
research.
REFERENCES
Boser, B. E., Guyon, I. M., and Vapnik, V. N. (1992). A
training algorithm for optimal margin classifiers. In
COLT’92: Proceedings of the Fifth Annual Workshop
on Computational Learning Theory, pages 144–152,
New York, USA.
Chang, C.-C. and Lin, C.-J. (2001). LIBSVM: a library for
support vector machines. Software available at
http:
//www.csie.ntu.edu.tw/
˜
cjlin/libsvm
.
Guler, I. and Ubeyli, E. (2007). Multiclass support vector
machines for EEG-signals classification. 11(2):117–
126.
Haykin, S. (2001). Adaptive Filter Theory (4th Edition).
Prentice Hall.
Khachab, M., Kaakour, S., and Mokbel, C. (2007). Brain
imaging and support vector machines for brain com-
puter interface. In Proc. 4th IEEE International Sym-
posium on Biomedical Imaging: From Nano to Macro
ISBI 2007, pages 1032–1035.
McEwen, J. A. and Anderson, G. B. (1975). Modeling the
stationarity and gaussianity of spontaneous electroen-
cephalographic activity. (5):361–369.
Mouri˜no, J. (2003). EEG-based Analysis for the Design
of Adaptive Brain Interfaces. PhD thesis, Universitat
Polit`ecnica de Catalunya, Barcelona, Spain.
Nicolaou, N., Georgeou, J., and Polycarpou, M. (2008).
Autoregressive features for a thought-to-speech con-
verter. In Proceedings of the International Conference
on Biomedical Electronics and Devices - BIODE-
VICES 2008, pages 11–16, Funchal, Portugal.
Pons, J. L. (2008). Wearable Robots: Biomechatronic Ex-
oskeletons (1st Edition). Wiley, Madrid, Spain.
Sajda, P., Muller, K.-R., and Shenoy, K. (2008). Brain-
computer interfaces [from the guest editors]. Signal
Processing Magazine, IEEE, 25(1):16–17.
Schl¨ogl, A. (2003). Data set: BCI-experiment.
http://ida.first.fraunhofer.de/projects/
bci/competition\_ii
.
Schl¨ogl, A., Neuper, C., and Pfurtscheller, G. (1997). Sub-
ject specific EEG patterns during motor imaginary
[sic.: for imaginary read imagery]. In Neuper, C., edi-
tor, Proc. 19th Annual International Conference of the
IEEE Engineering in Medicine and Biology society,
volume 4, pages 1530–1532.
Shoker, L., Sanei, S., and Sumich, A. (2005). Distin-
guishing between left and right finger movement from
EEG using svm. In Sanei, S., editor, Proc. 27th
Annual International Conference of the Engineering
in Medicine and Biology Society IEEE-EMBS 2005,
pages 5420–5423.
BIODEVICES 2009 - International Conference on Biomedical Electronics and Devices
12