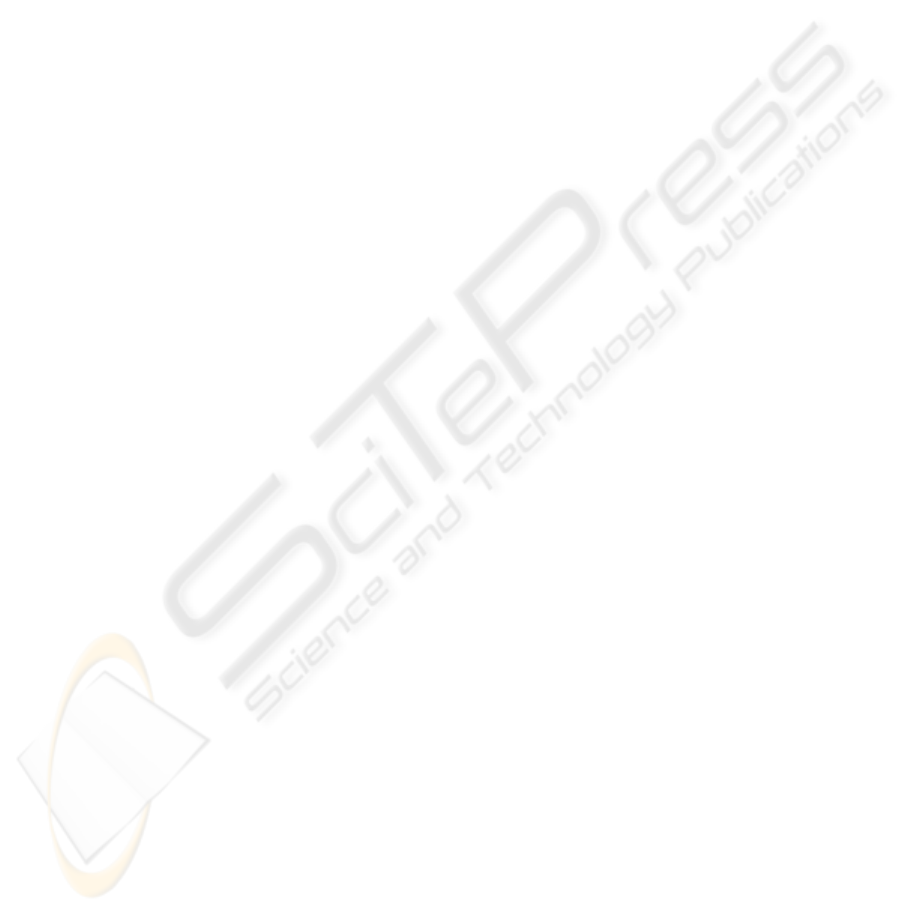
Also is interesting to observe that with 13
independent components we obtain a very good
result of 80.40% ± 0.78 that outperforms the
previous results showed in (Briceño et al., 2002). As
the number of components is small, we simplify the
classification step.
7 CONCLUSIONS
In this present work, we have presented an
improvement of an automatic leaves recognition
system using Independent Component Analysis and
classifying with multilayer perceptron neural
network. The transformation and reduction of data
contribute to increase its discrimination, from
78.33% using contour parameterization + HMM
(Briceño et al., 2002), to 80.77% using contour
parameterization + ICA + Neural Network.
The advantage of using ICA is twofold: first, we
increase the classification results, specially
diminishing the variance, and second we reduce the
features dimension, giving as a result a less complex
classifier.
Future work will be done exploring other
different ICA algorithms combined with other
classifiers in order to diminish the complexity of the
whole classification system.
ACKNOWLEDGEMENTS
The first author acknowledges support from the
Ministerio de Educación y Ciencia of Spain under
the grant TEC2007-61535/TCM, and from the
Universitat de Vic under the grant R0912.
REFERENCES
Lu, F., Milios, E. E., 1994. Optimal Spline Fitting to
Planar Shape. In Elsevier Signal Processing, No. 37,
pp 129-140.
Loncaric, S., 1998. A Survey of Shape Analysis
Techniques. In Pattern Recognition, Vol. 31 No. 8, pp
983-1001.
Rabiner, L., Juang, B H., 1993. Fundamentals of Speech
Recognition. Prentice Hall, Englewood Cliffs, New
Jersey.
Briceño, J. C., Travieso, C. M., Ferrer, M. A., 2002.
Automatic Recognition of Simple Laurisilva
Canariensis Leaves, by Perimeter Characterization. In
IASTED International Conference on Signal
Processing, Pattern Recognition and its Applications,
pp. 249 – 254.
Burges, C.J.C., 1998. A Tutorial on Support Vector
Machines for Pattern Recognition. In Data Mining and
Knowledge Discovery, Vol. 2, Nº2, pp. 121-167.
Jolliffe I.T., 2002. Principal Component Analysis. Series:
Springer Series in Statistics, Springer, NY 2nd edition.
Jutten, C., Herault, J., 1991. Blind separation of sources,
Part 1: an adaptive algorithm based on neuromimetic
architecture. In Signal Processing (Elsevier), Vol. 24,
Issue 1, pp. 1-10
Hyvärinen, A., Karhunen, J., Oja, E., 2001. Independent
Component Analysis, New York, USA: John Wiley &
Sons.
Duda, R.O., Hart, P.E., Stork, D.G., 2000. Pattern
Classification. Wiley Interscience, 2nd Edition.
Bishop, C., 1996. Neural Networks for Pattern
Recognition. Clarendon, UK: Oxford University Press.
Lu, F., Milios, E.E., 1994. Optimal Spline Fitting to Planar
Shape. In Elsevier Signal Processing No. 37, pp. 129-
140.
Loncaric, S., 1998. A Survey of Shape Analysis
Techniques. In Pattern Recognition. Vol. 31 No. 8,
pp. 983-1001.
Huang, Z., Cohen, F., 1996. Affine-Invariant B-Spline
Moments for Curve Matching. In IEEE Transactions
on Image Processing, Vol. 5. No. 10. pp. 824-836.
Mitchell, T.M., 1997. Machine Learning. McGraw-Hill.
Sanchez-Poblador, V., Monte-Moreno, E., Solé-Casals, J.,
2004. ICA as a preprocessing technique for
Classification. In Independent Component Analysis
and Blind Signal Separation, Lecture Notes in
Computer Science, Springer-Verlag Volume
3195/2004, pp. 1165-1172.
Cardoso, J.F., Souloumiac, A., 1996. Jacobi angles for
simultaneous diagona-lization, In SIAM. Journal
Mathematics Analysis Application, pp. 161-164.
AUTOMATIC RECOGNITION OF LEAVES BY SHAPE DETECTION PRE-PROCESSING WITH ICA
467