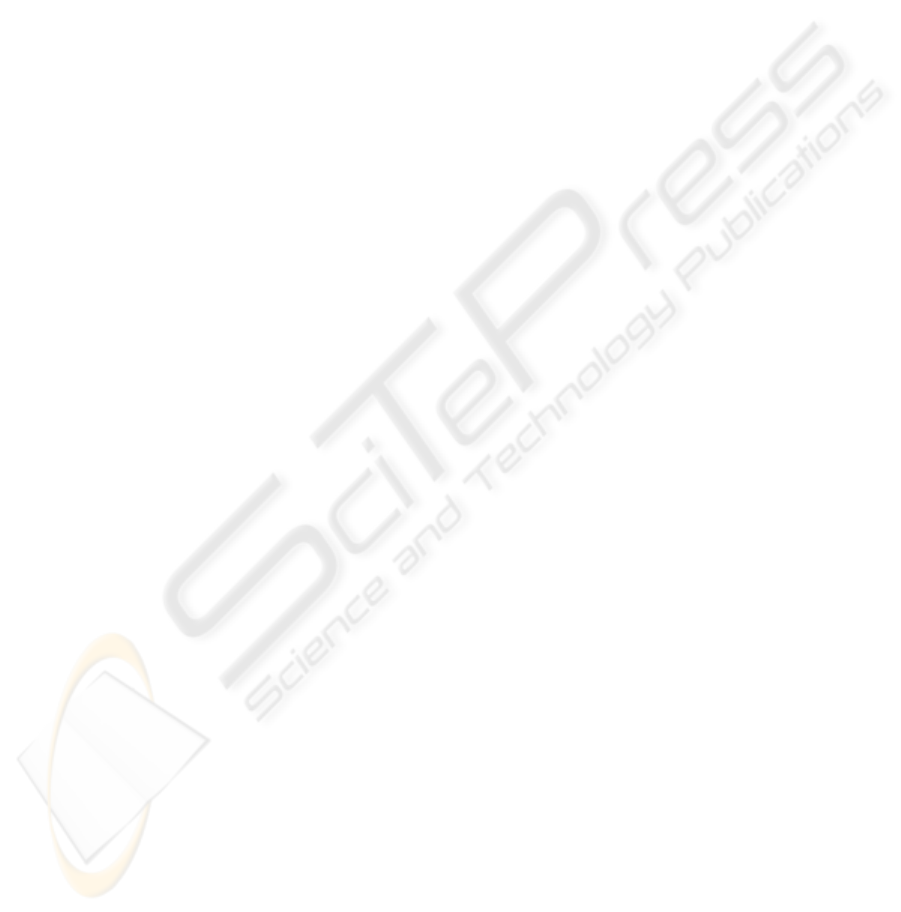
The Bhattacharyya distance was then calculated
to quantify the data group separation visually
inspected. The results on Figure 6 show that the
separation between groups M1 and M3 are bigger,
while the overlap is more evident between groups
M1- M2 and M2-M3. Therefore, as the movements
involved are more distant or different from each
other, more the set of signals become separated in its
own group or intra-class. This result was expected
but the experiment, especially with BTG group, has
showed the possibility of discriminating different
sets of data from different tests and obtaining a
reasonably understandable set of data, where classes
and groups could be linearly separated.
5 CONCLUSIONS
Our experimental results have suggested that
analyzing a given myoelectrical set of signals by
descriptive statistical tools is a hard task due to a
high data dimensionality and noise presence.
Acquiring myoelectrical signals with superficial
electrodes means to deal with a highly noisy
susceptible set of data due to electrical variances of
the skin and electrodes displacements during muscle
movements. The use of linear transformation can
make the multivariate data set analysis easier.
Considering the arm horizontal movement and
the acquired set of data used in this research, a
discriminant linear analysis showed that it is
possible not only to characterize the angular joint
position, but also to infer that different movements
recruit similar amounts of energy to be executed.
Our experimental results confirm that using
multivariate statistical analysis, myoelectric signal
recognition can be significantly improved after
linear transformations, which are practical and
feasible methods to analyze such multivariate high
dimension and small sample size data for further
classification.
ACKNOWLEDGEMENTS
The authors would like to thank the support
provided by the State of São Paulo Research
Foundation (FAPESP) under the grant 05/02899-4.
REFERENCES
Bittar, L. M. and Castro, M. C. F., 2008. Elbow Flexion
and Extension Movements Characterization by Means
of EMG. Biodevices 2008, Portugal, pp. 147-150.
Fukunaga, K., 1990. Introduction to statistical pattern
recognition, 2
nd
edition. Academic Press, Boston.
Hargrove, L., Englehart, K. and Hudgins, B., 2008. A
training strategy to reduce classification degradation
due to electrode displacements in pattern recognition
based myoeletric control. Biomedical Signal
Processing and Control, 3(2), pp.175-180.
Henneberg, K., 2000. Principles of Electromyography. In:
Bronzino, J. D. (Eds.), The biomedical Engineering
Handbook, Vol. 1, 2
nd
edition. CRC Press, New York.
cap. 14.
Jain, A. K. and Chandrasekaran, B., 1982, Dimensionality
and Sample Size Considerations in Pattern
Recognition Practice. In: Krishnaiah, P. R. and Kanal,
L. N. (Eds.), Handbook of Statistics, Vol. 2. North-
holland, Amsterdam. pp. 835-855.
Kleissen, R. F. M., Buurke, J. H., Harlaar, J., Zivold, G. ,
1998. Electromyography in the biomechanical analysis
of human movement and its clinical application. Gait
and Posture, 8(2), pp.143-158.
Thomaz, C. E. and Gillies, D. F., 2005. A Maximum
Uncertainty LDA-based approach for Limited Sample
Size problems - with application to Face Recognition.
In: Proceedings of SIBGRAPI’05, IEEE CS Press, pp.
89-96.
Thomaz, C. E., Gillies, D. F. and Feitosa, R. Q., 2004. A
New Covariance Estimate for Bayesian Classifiers in
Biometric Recognition, IEEE Trans. Circuits Syst.
Video Techn., 14(2), pp. 214-223.
BIOSIGNALS 2009 - International Conference on Bio-inspired Systems and Signal Processing
232