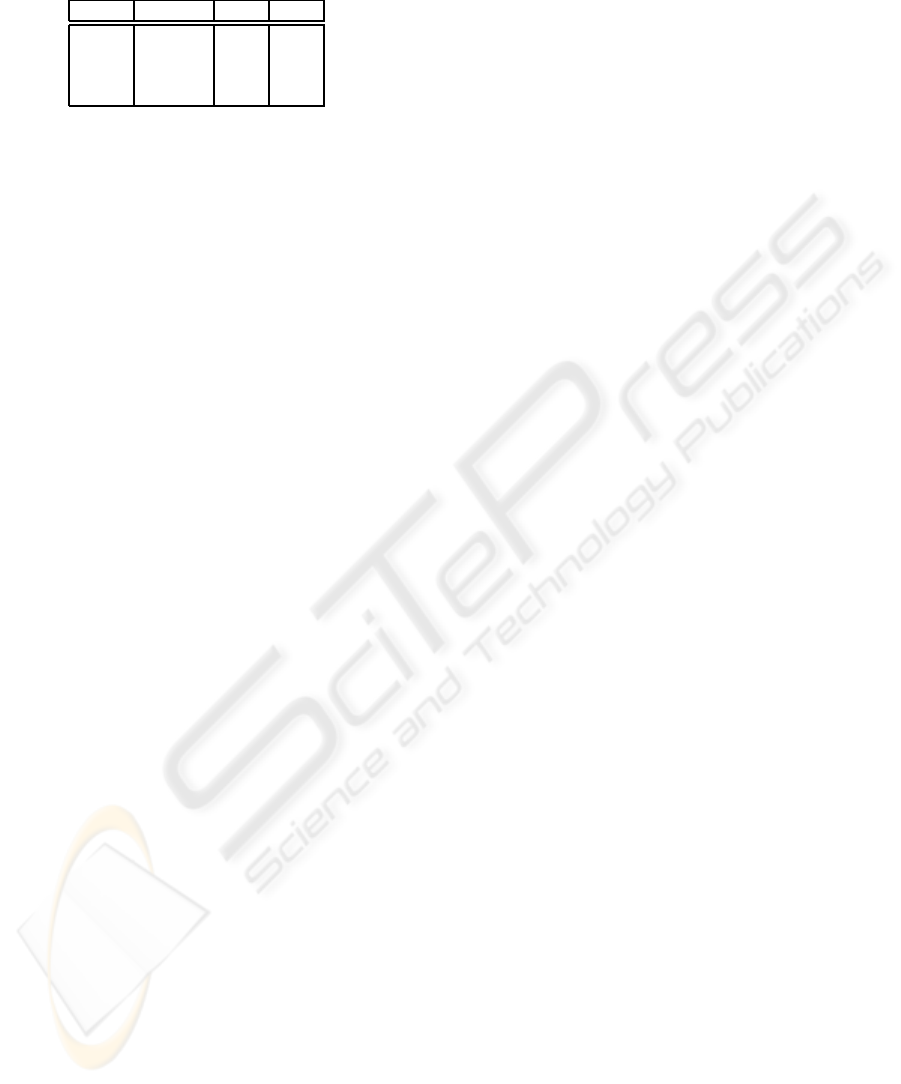
Table 3: Inter-patient correct classification rate, when merg-
ing the L R and N types.
N+L+R V P
LI 81.1 80.7 92.6
CSI 81.3 80.7 92.7
DTW 74.4 79.2 90.0
TS 60.0 72.6 72.6
6 CONCLUSIONS
In this work, four time-alignment methods are evalu-
ated and compared in the context of supervised heart
beat classification. Amongst these methods are three
time series resampling algorithms by linear interpo-
lation, cubic splines interpolation and trace segmen-
tation. The fourth method is a realignment algorithm
by dynamic time warping. The multiple sources of
noise and artifacts are filtered by means of a time-
frequency decomposition by discrete wavelet trans-
form. The downsampling induced by DWT approx-
imation coefficients also reduces the dimension of the
observations. Since it only relies on a dissimilarity
measure between observations, the KNN classifier is
a natural choice for supervised classification of time
series in multiple classes.
Experiments are conducted on a representative
dataset built from the standard public MIT-BIH ar-
rhythmia database. The five main types of heart beats
are considered. The experimental dataset is created
so as to include an equal number of beats per class
with all patients being fairly represented. For intra-
patient classification, very similar results are obtained
by the two interpolation methods with an average cor-
rect classification rate of 90%. Surprisingly, the dy-
namic time warping algorithm provides slightly lower
results with an average of 88%. Amongst the four
methods, the trace segmentation obtains the worst re-
sults with an average of only 70%. On the other hand,
the results obtained for inter-patient classification are
unsatisfactory, especially for the L and R types. How-
ever, the low yield obtained for these classes are
preliminary, since the number of patients with these
pathologies in the MIT-BIH database is insufficient
for generalization. When merging these three classes
together as recommended by the AAMI, the results
achieved are then comparable to intra-patient classifi-
cation.
Very few other studies work on a reliable dataset
with multi-class, inter and intra patient classification
and comparisons to other works are difficult to ob-
tain. Further works will include a comparison with
the results of other QRS classification and feature ex-
traction methods using the same dataset.
ACKNOWLEDGEMENTS
G. de Lannoy is funded by a Belgian F.R.I.A. grant.
This work was partly supported by the Belgian
“R´egion Wallonne” ADVENS 4994 project.
REFERENCES
Chudacek, V., Petrik, M., Georgoulas, G., Cepek, M., Lhot-
ska, L., and Stylios, C. (2007). Comparison of seven
approaches for holter ecg clustering and classification.
Engineering in Medicine and Biology Society, 2007.
EMBS 2007. 29th Annual International Conference of
the IEEE, pages 3844–3847.
Clifford, G. D., Azuaje, F., and McSharry, P. (2006). Ad-
vanced Methods And Tools for ECG Data Analysis.
Artech House, Inc., Norwood, MA, USA.
Cuesta-Frau, D., Pres-Corts, J. C., and Andreu-Garcia, G.
(2003). Clustering of electrocardiographic signals in
computer-aided holter analysis. Computer Methods
and Programs in Biomedicine, 72:179–196.
De Boor, C. (1978). A practical guide to splines. Springer-
Verlag, New York.
Goldberger, A., Amaral, L., Glass, L., Hausdorff, J., Ivanov,
P., Mark, R., Mietus, J., Moody, G., Peng, C.-K.,
and Stanley, H. (2000). PhysioBank, PhysioToolkit,
and PhysioNet: Components of a new research re-
source for complex physiologic signals. Circulation,
101(23):e215–e220.
Ham, F. and Han, S. (1996). Classification of cardiac ar-
rhythmias using fuzzy artmap. IEEE Transactions on
Biomedical Engineering, 43(4):425–429.
Jekova, I., Botrolan, G., and Christov, I. (2008). As-
sessment and comparison of different methods for
heartbeats classification. Medical Engineering and
Physics, 30:248–257.
Maier, C., Dickhaus, H., and Gittinger, J. (1999). Unsuper-
vised morphological classification of qrs complexes.
Computers in Cardiology 1999, pages 683–686.
Mallat, S. (1999). A Wavelet Tour of Signal Processing, Sec-
ond Edition (Wavelet Analysis and Its Applications).
IEEE press, San Diego. ISBN 978-0124666061.
Moraes, J., Seixas, M., Vilani, F., and Costa, E. (2002). A
real time qrs complex classification method using ma-
halanobis distance. Computers in Cardiology, 2002,
pages 201–204.
Myers, C. S. and Rabiner, L. (1981). A comparative study
of several dynamic time-warping algorithms for con-
nected word recognition. The Bell System Technical
Journal, 60:1389–1409.
Rodriguez-Sotela, J. L., Cuesta-Frau, D., and Castellanos-
Dominguez, G. (2007). An improved method for un-
supervised analysis of ecg beats based on wt features
and j-means clustering. Computers in Cardiology,
34:581–584.
BIOSIGNALS 2009 - International Conference on Bio-inspired Systems and Signal Processing
244