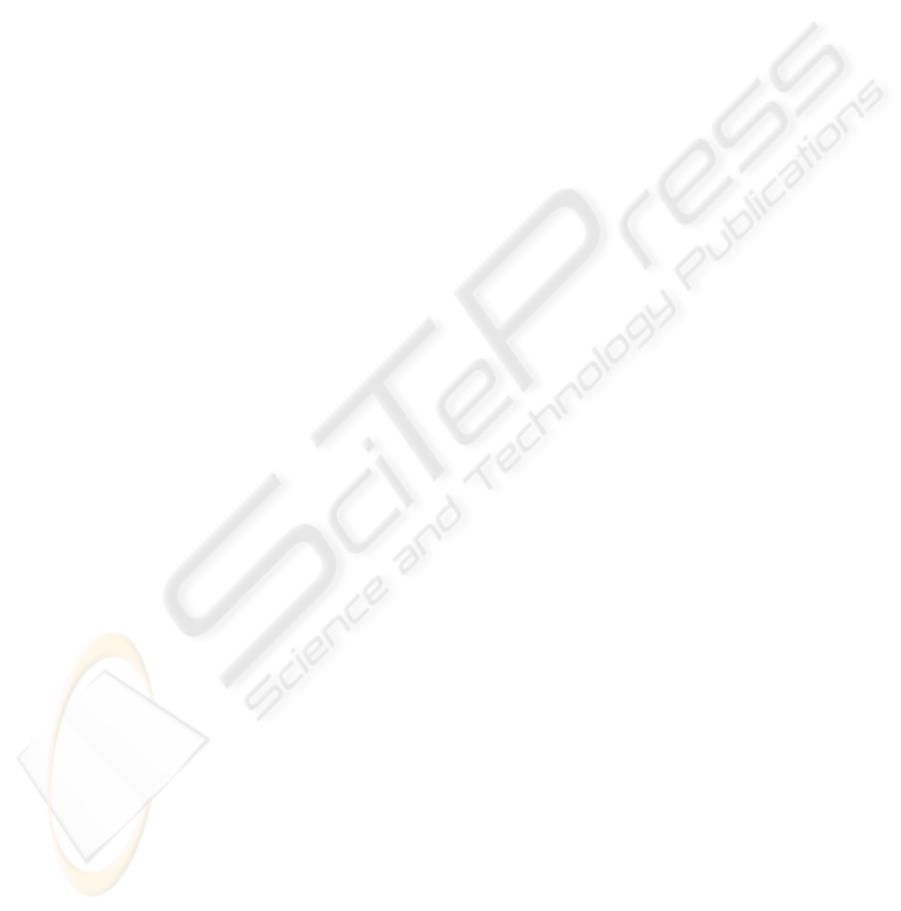
specifically tailored to a given classifier, the choice
of linear or non-linear classifiers become less impor-
tant (
˚
Aberg and Wessberg, 2007). However, stochas-
tic feature selection methods tend to be computation-
ally intensive, and since the univariate feature selec-
tion method is substantially faster it can be preferred
in time-limited circumstances such as real-time anal-
ysis.
This study does not use concurrently registered
EEG and fMRI data. In fact, different experimen-
tal conditions are used (motor actions versus tactile
stimulus), but the underlying problem of single-trial
classification and the implications thereof remain. A
simultaneous EEG and fMRI registration study is cur-
rently in progress, as is further research into the uti-
lization of a unified pattern recognition approach to
the analysis of both modalities.
5 CONCLUSIONS
Pattern recognition, where classification models are
entirely data-driven, is a suitable approach to a uni-
fied, conceptually identical analysis of fMRI and EEG
data. Using classifier-based techniques, it is possi-
ble to automatically identify and label cortical pat-
terns related to given experimental conditions present
in singe-trial data – for signals acquired with EEG as
well as with fMRI. Investigating state-of-the-art clas-
sifiers, the support vector machine was found to out-
perform the artificial neural networks, whereas non-
linear classifiers performed better than linear such for
EEG data and vice versa for fMRI data.
ACKNOWLEDGEMENTS
This study was supported by the Swedish Research
Council (grant 3548), the Sahlgrenska University
Hospital (grant ALFGBG 3161), and the foundation
of Magnus Bergvall.
REFERENCES
˚
Aberg, M. C., L
¨
oken, L., and Wessberg, J. (2008). An evo-
lutionary approach to multivariate feature selection for
fMRI pattern analysis. Proceedings of the Interna-
tional Conference on Bio-inspired Systems and Signal
Processing.
˚
Aberg, M. C. and Wessberg, J. (2007). Evolutionary op-
timization of classifiers and features for single trial
EEG discrimination. BioMedical Engineering Online,
6(32).
Cox, D. D. and Savoy, R. L. (2003). Functional magnetic
resonance imaging (fMRI) ’brain reading’: detecting
and classifying distributed patterns of fMRI activity
in human visual cortex. Neuroimage, 19(2 Pt 1):261–
270.
Davatzikos, C., Ruparel, K., Fan, Y., Shen, D., Acharyya,
M., Loughead, J., Gur, R., and Langleben, D. (2005).
Classifying spatial patterns of brain activity with ma-
chine learning methods: Application to lie detection.
Neuroimage, 28:663–668.
Fisch, B. J. (1999). Fisch & Spehlmann’s EEG Primer;
Basic Principles of Digital and Analog EEG. Elsevier
Science, third edition edition.
Friston, K. J., Holmes, A. P., Worsley, K. J., Poline, J. P.,
Frith, C. D., and Frackowiak, R. S. J. (1994). Statisti-
cal parametric maps in functional imaging: A general
linear approach. Human Brain Mapping, 2(4):189–
210.
Kamitani, Y. and Tong, F. (2005). Decoding the visual and
subjective contents of the human brain. Nature Neu-
roscience, 8(5):679–685.
LaConte, S., Strother, S., Cherkassky, V., Anderson, J., and
Hu, X. (2005). Support vector machines for temporal
classification of block design fMRI data. Neuroimage,
26(2):317–29.
Mitchell, T. M., Hutchinson, R., Niculescu, R. S., Pereira,
F., Wang, X., Just, M., and Newman, S. (2004). Learn-
ing to decode cognitive states from brain images. Ma-
chine Learning, 57(1-2):145–175.
Mour
˜
ao-Miranda, J., Bokde, A. L., Born, C., Hampel, H.,
and Stetter, M. (2005). Classifying brain states and de-
termining the discriminating activation patterns: Sup-
port vector machine on functional MRI data. Neu-
roImage, 28(4):980–95.
Norman, K. A., Polyn, S. M., Detre, G. J., and Haxby, J. V.
(2006). Beyond mind-reading: multi-voxel pattern
analysis of fMRI data. Trends in Cognitive Sciences,
10(9):424–430.
Pfurtscheller, G., Flotzinger, D., Mohl, W., and Peltoranta,
M. (1992). Prediction of the side of hand movements
from single-trial multi-channel EEG data using neural
networks. Electroencephalography and Clinical Neu-
rophysiology, 82(4):313–5.
Polyn, S. M., Natu, V. S., Cohen, J. D., and Norman,
K. A. (2005). Category-specific cortical activity
precedes retrieval during memory search. Science,
310(5756):1963–6.
Trejo, L. and Shensa, M. (January 1999). Feature extraction
of event-related potentials using wavelets: An appli-
cation to human performance monitoring. Brain and
Language, 66:89–107(19).
BIOSIGNALS 2009 - International Conference on Bio-inspired Systems and Signal Processing
278