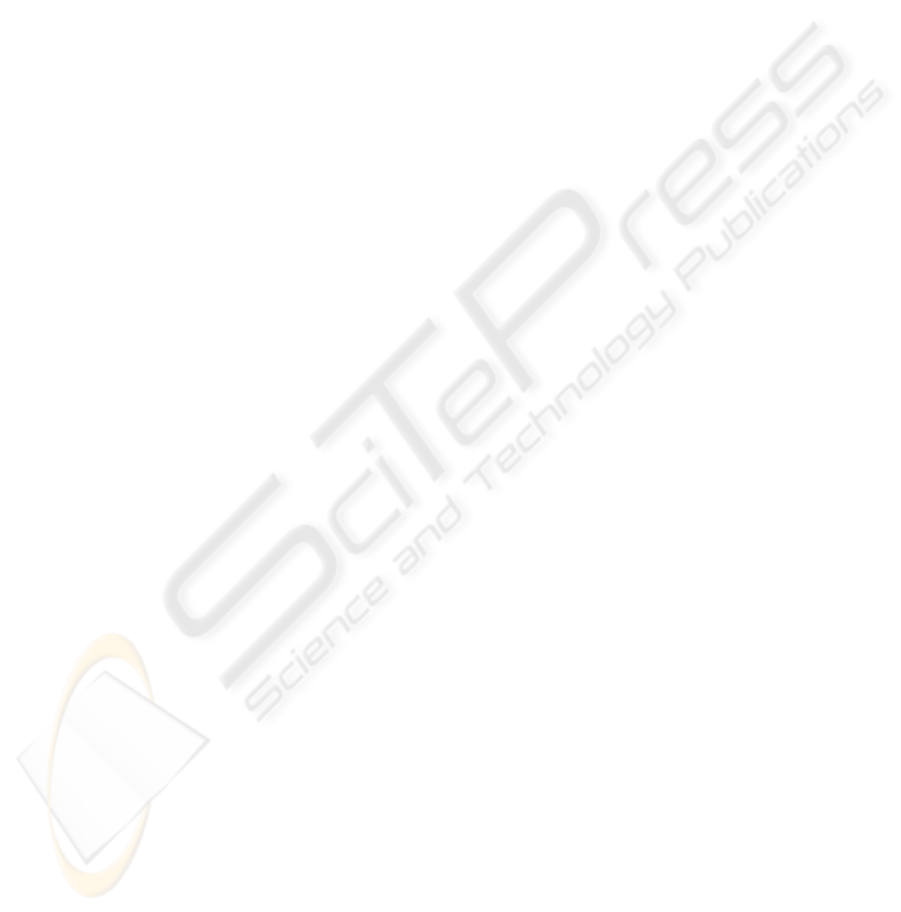
gramming of several saccades in parallel. In fact,
we tested three models: the “1M” model program-
ming four saccades in parallel from the same foveated
point, the “2M” model programming two saccades in
parallel and the “4M” model programming only one
saccade at a time. This study showed that the “1M”
model is not realistic and the “4M” model seems to be
the most realistic. The behaviour of the “2M” model
illustrates that recomputing saliency in updating the
foveated point is beneficial. The best performance
comes however from the “4M” model presenting ef-
fectiveness of the reinitialization at each fixation. We
can conclude that saccade programming in parallel
seems not to be used by subjects when they have to
look freely at natural images.
Secondly, this study shows the positive effect of
the spatially variant retinal resolution on the pre-
diction quality. Whatever the saccade programming
strategy is, the models including the spatially vari-
ant retinal resolution greatly outperform the models
with constant resolution in terms of the quality of
fixation prediction and saccade distribution. The pa-
rameter α which controls the resolution decrease has
an important impact on saccade distribution (disper-
sion and mode position). Moreover, we found the
expected range of value for this parameter using our
model to compute saliency maps. We also notice that
if we have the same mode position, the dispersion of
the saccade distribution remains smaller on predicted
data than on experimental data, as we only consider a
bottom-up model and we have only one parameter to
adjust.
In our models, a foveated point for the next sac-
cade is selected from subjects’ fixations instead of be-
ing looked for in the present saliency map. While fix-
ations are different from one subject to another, the
model is a subject-dependent model. If we want to go
further in creating a more general model of predict-
ing eye movements automatically, the model would
take into account human task, for example catego-
rization or information search, and hence passes from
a region-predicting model to a scanpath-predicting
model.
ACKNOWLEDGEMENTS
This work is partially supported by grants from the
Rhˆone-Alpes Region with the LIMA project. T. Ho-
Phuoc’s PhD is funded by the French MESR.
REFERENCES
Beaudot, W., Palagi, P., and Herault, J. (1993). Realistic
simulation tool for early visual processing including
space, time and colour data. In International Work-
shop on Artificial Neural Networks, LNCS, volume
686, pages 370–375, Barcelona. Springer-Verlag.
Egeth, H. E. and Yantis, S. (1997). Visual attention: Con-
trol, representation, and time course. In Annual Re-
view of Psychology, volume 48, pages 269–297.
Geisler, W. S. and Perry, J. S. (1998). A real-time foveated
multiresolution system for low-bandwidth video com-
munication. In Human Vision and Electronic Imaging,
Proceedings of SPIE, volume 3299, pages 294–305.
Hansen, T., Sepp, W., and Neumann, H. (2001). Recurrent
long-range interactions in early vision. In S. Wermter,
J. A. and Willshaw, D., editors, Emergent Neural
Computational Architectures Based on Neuroscience,
LNCS/LNAI, volume 2036, pages 139–153.
Henderson, J. M. (2003). Human gaze control in real-world
scene perception. In Trends in Cognitive Sciences,
volume 7, pages 498–504.
Itti, L. (2006). Quantitative modeling of perceptual salience
at human eye position. In Visual Cognition, vol-
ume 14, pages 959–984.
Itti, L., Koch, C., and Niebur, E. (1998). A model of
saliency-based visual attention for rapid scene anal-
ysis. In IEEE Transactions on Pattern Analysis and
Machine Intelligence, volume 20, pages 1254–1259.
Koch, C. and Ullman, S. (1985). Shifts in selective visual
attention: towards the underlying neural circuitry. In
Human Neurobiology, volume 4, pages 219–227.
McPeek, R. M., Skavenski, A. A., and Nakayama, K.
(1998). Adjustment of fixation duration in visual
search. In Vision Research, volume 38, pages 1295–
1302.
McPeek, R. M., Skavenski, A. A., and Nakayama, K.
(2000). Concurrent processing of saccades in visual
search. In Vision Research, volume 40, pages 2499–
2516.
Navon, D. (1977). Forest before trees: the precedence of
global features in visual perception. In Cognitive Psy-
chology, volume 9, pages 353–383.
Parkhurst, D., Law, K., and Niebur, E. (2002). Modeling
the role of salience in the allocation of overt visual
attention. In Vision Research, volume 42, pages 107–
123.
Perry, J. S.(2002). http://fi.cvis.psy.utexas.edu/software.shtml.
Torralba, A., Oliva, A., Castelhano, M. S., and Henderson,
J. M. (2006). Contextual guidance of eye movements
and attention in real-world scenes: The role of global
features in object search. In Psychological Review,
volume 113, pages 766–786.
Treisman, A. and Gelade, G. (1980). A feature integra-
tion theory of attention. In Cognitive Psychology, vol-
ume 12, pages 97–136.
Wandell, B. A. (1995). Foundations of Vision. Stanford
University.
BIOSIGNALS 2009 - International Conference on Bio-inspired Systems and Signal Processing
64