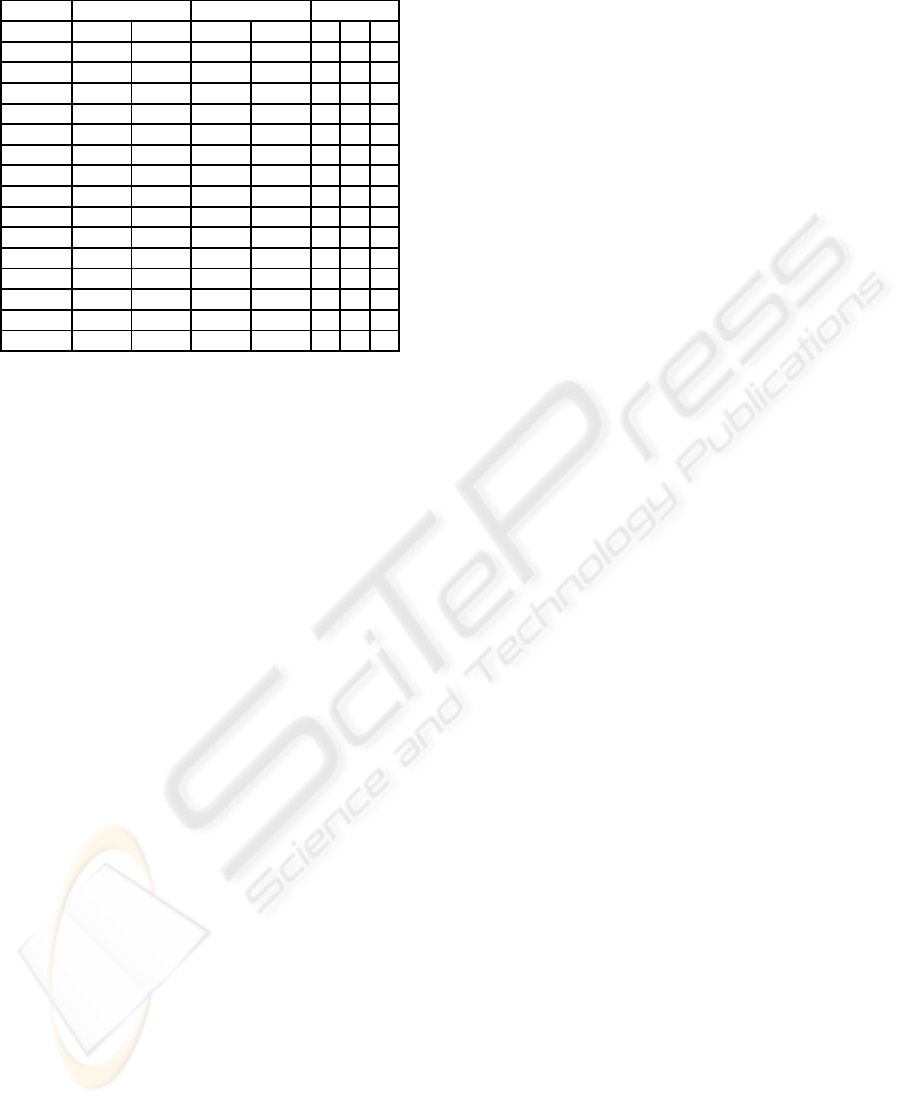
Table 2: Patterns probabilities for 31 and 41.
31 41 Bridges
Pattern The. Obs. The. Obs. 0 1 2
ABCD 0 0 .0938 .2611 0 0 0
ABCA .0741 .15 .0938 .1519 0 0 1
ABCB .0741 .1315 .0938 .0926 0 1 0
ABAC .0741 .1278 .0938 .0926 0 1 0
ABCC .0741 .0667 .0938 .087 1 0 0
ABBC .0741 .0796 .0938 .063 1 0 0
AABC .0741 .0685 .0938 .0722 1 0 0
ABBA .0741 .0611 .0469 .037 1 0 1
ABAB .0741 .0593 .0469 .0333 0 2 0
AABA .0741 .063 .0469 .0222 1 1 1
ABAA .0741 .0574 .0469 .0204 1 1 1
AABB .0741 .0463 .0469 .0222 2 0 0
ABBB .0741 .0352 .0469 .0204 2 1 0
AAAB .0741 .0352 .0469 .0185 2 1 0
AAAA .037 .0185 .0156 .0056 3 2 1
strated only in 5 of 7 games, where at least one of
probabilities for playing with glove significantly de-
viates from case, if it is played with mouse. But, there
is no plausible explanation for this deviation at time.
We counted tuples, which consists of own actual deci-
sion plus own three last decisions plus opponents last
three decisions. In game 31 for example, we have 4
tuples, each of them is observed 4 times. As exam-
ple, the tuple {rock, {rock, paper, scissors}, {paper,
rock, paper}} was observed in threads of four defer-
ent persons. The probability for one tuple in 31 to be
observed more than 3 times is about 0.126h. Persons
play in a rhythm which is far from iid. Further they
transform in consequence of this deviation a repeated
matrix game to an extensive game, in which one must
consider his turns in dependence of own and oppo-
nents last turns or in common speech fill the rhythm
of the game.
The latter brought us to calculate probabilistic
grammatic of human behavior in games 31 and 41.
We used methods of (Budescu and Rapoport, 1994).
Variables A, B, C, D, . . . ∈ gestures have all different
values. Patterns are abstract types of sequences and
constructed of these variables. For instance, the se-
quences {paper,rock} and {fire,water} are both of
pattern AB. We used patterns with length 4, because
of limited size of our data. We calculated theoreti-
cal probabilities of these patterns according to thesis,
that humans used equal distributions in games 31 and
41. Per game we can count (30 − 3) ∗ 2 ∗ 10 = 540
sequences and assign them to patterns. Additionally
we counted bridges (variable repetition) over 0, 1, 2
elements in the patterns for possible explanation of
deviation. Tab.2 shows the results.
8 CONCLUSIONS
Our paper showed a practical way for construction
of a highly scaleable multi-agent system for defini-
tion, providing and recording of SI between real-
world agents, human and artificial respectively. Espe-
cially, we presented a multi-agent system design and
a PN based language. It produced data for a concrete
scenario - using MSE in zero sum by humans. The
recorded data was analyzed and showed results simi-
lar to already alluded in literature. Core elements of
our system can be downloaded from our home page.
REFERENCES
Bellifemine, F., Poggi, A., and Rimassa, G. (2001). Jade: a
fipa2000 compliant agent development environment.
In AGENTS ’01. ACM.
Budescu, D. V. and Rapoport, A. (1994). Subjective ran-
domization in one- and two-person games. Journal of
Behavioral Decision Making, 7.
F.Camerer, C. (2003). Behavioral Game Theory. Princeton
University Press, New Jersey.
Genesereth, M. R., Love, N., and Pell, B. (2005). General
game playing: Overview of the aaai competition. AI
Magazine, 26(2):62–72.
Harary, F. (1957). The number of oriented graphs. Michigan
Math. J., 4.
Heumer, G., Amor, H. B., and Jung, B. (2008). Grasp recog-
nition for uncalibrated data gloves: A machine learn-
ing approach. Presence: Teleoper. Virtual Environ.,
17:121–142.
Priese, L. and Wimmel, H. (2008). Petri-Netze. Springer.
Tagiew, R. (2008). Simplest scenario for mutual nested
modeling in human-machine-interaction. In Advances
in Artificial Intelligence. Heidelberg, Springer.
Tesfatsion, L. and Judd, K. (2006). Handbook of computa-
tional economics, volume 2. Elsevier/North-Holland.
ICAART 2009 - International Conference on Agents and Artificial Intelligence
590