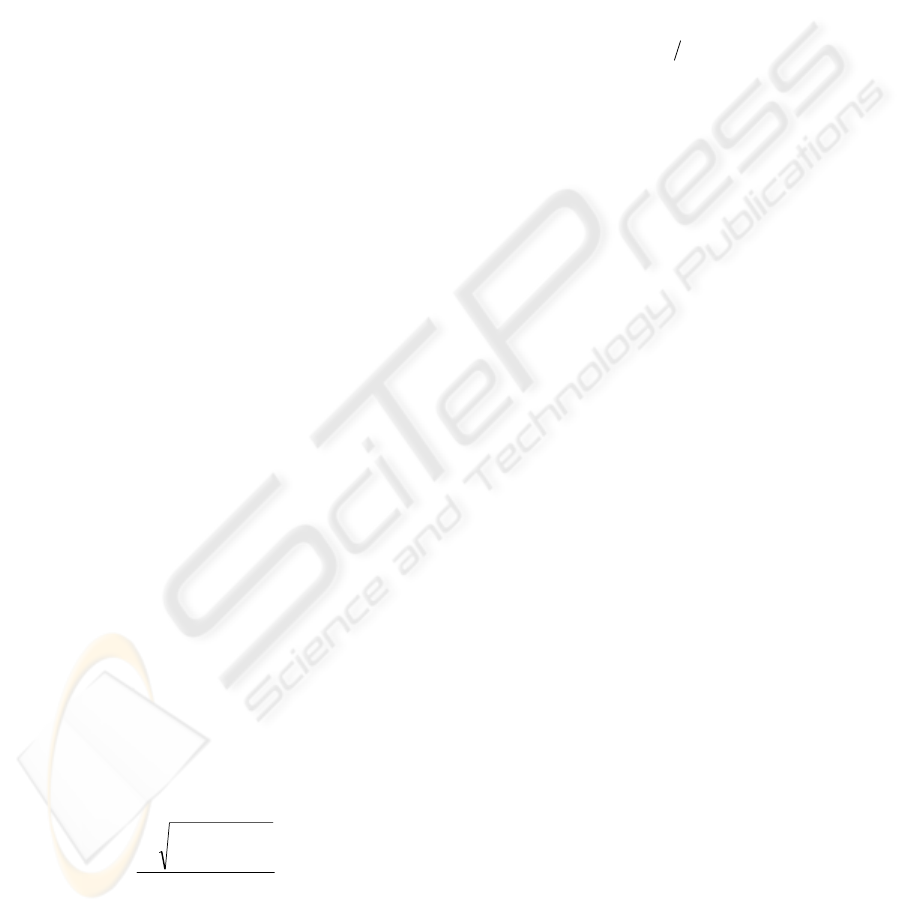
2 KOHONEN NEURAL
NETWORK
Kohonen neural networks typically consist of a
single layer of neurons arranged as a map, with the
number of outputs equal to the number of neurons,
and the number of inputs equal to the number of
weights in neurons. In practical applications 2-D
maps are the most commonly used
, since they allow
for good data visualization (Kohonen 2001).
Training data files in such networks consist of m
n-elements patterns X, where n is the number of the
network inputs. The competitive learning in KNN is
an iterative process. During each iteration, called an
epoch, all m vectors are presented to the network in
a random order. The full learning process requires
even hundreds epochs, which means even several
hundreds thousands presentations of a single pattern.
Once a single pattern is presented to the network,
several calculation steps may be performed by the
network. In the first step a distance between a given
pattern X and the weights vector W in every neuron
in the map is calculated using, for example, the
Euclidean or the Manhattan metric. In the next step
the winning neuron is identified, and this neuron in
the following step is allowed to adapt its weights.
In the Winner Takes All (WTA) learning method
only the winning neuron, whose vector W is the
closest to the pattern X, is allowed to adapt the
weights, while in the Winner Takes Most (WTM)
approach also neurons that belong to the winner’s
neighborhood change the weights.
The WTA algorithm offers poor convergence
properties, especially in case of large number of
neurons. In this approach some neurons remain dead
i.e. they absorb the computational resources, but
never win and never become representatives of any
data class. The WTM algorithm, on the other hand,
is more complex, since it additionally involves the
neighborhood mechanism, which increases the
computational complexity, but this mechanism
usually activates all neurons in the network (Mokriš
2004), thus minimizing the quantization error. This
error is defined as follows (w
w
are weights of the
winning neuron):
m
wx
Qerr
m
j
n
l
wl
l
j
∑∑
==
−
=
11
2
)(
(1)
The main problem in the WTM algorithm is very
large number of operations, especially in case of
large number of patterns and epochs. In hardware
implementations effective methods to minimize the
number of operations are therefore required.
3 THE PROPOSED TECHNIQUE
In a typical WTM learning algorithm the neighbor-
hood radius R at the beginning of the training
process is set up to the maximum possible value so it
covers an entire map. After each epoch the radius R
decreases linearly by a small value to zero. In
practice, as number of epochs usually is much larger
than the maximum value (R
max
) of the neighborhood
radius R, therefore the radius decreases always by
‘1’ after the number of epochs equals to:
)
MAX
MAX
round Rll
(2)
In equation (2) l
max
is the total number of epochs in
the learning phase. Value of the l parameter usually
is in the range between 20 and 200
, depending on the
network’s dimensions. In case of an example map
with 10x10 neurons and the rectangular neighbor-
hood R
max
equals to 19 (Długosz and Kolasa, 2008).
To verify this ‘linear’ approach authors designed
a software model of the WTM KNN. Simulations
have been performed for different network dimen-
sions and different training data files. Observation of
the quantization error in the time domain shows that
the ‘linear’ approach is not optimal. The example
illustrative waveforms of the Q_error are in this case
shown in Fig. 1 for an example training data file
with 1000 patterns X, for selected network dimen-
sions 20x20, 10x10 and 4x4 neurons. The quantiza-
tion error is calculated after each epoch i.e. always
after presentation of 1000 training patterns X. This
does not increase significantly the computational
complexity of an entire learning process.
The first important observation is that when the
neighborhood radius R is larger than some critical
value, the quantization error does not decrease,
which means that in this period the network does not
make the progress in training. This critical value is
usually small, between 4 and 7 for different network
dimensions as illustrated in Fig. 1. The important
conclusion at this point is that the learning process
may start with the value of the radius R, which is
much smaller than the maximal value R
max
. This
significantly shorts the entire training process.
The second important observation is that the
error does not decrease monotonously with time, but
there are some distinct activity phases, just after the
radius R is switched to the smaller value, in which it
decreases abruptly and then some stagnation phases,
in which it does not decrease. The length of a single
activity phase usually is between 2-4 epochs inde-
pendently on the network dimensions.
The optimization technique proposed by authors
eliminates these stagnation phases by incorporation
of the multistage filtering of the quantization error in
NEW FAST TRAINING ALGORITHM SUITABLE FOR HARDWARE KOHONEN NEURAL NETWORKS
DESIGNED FOR ANALYSIS OF BIOMEDICAL SIGNALS
365