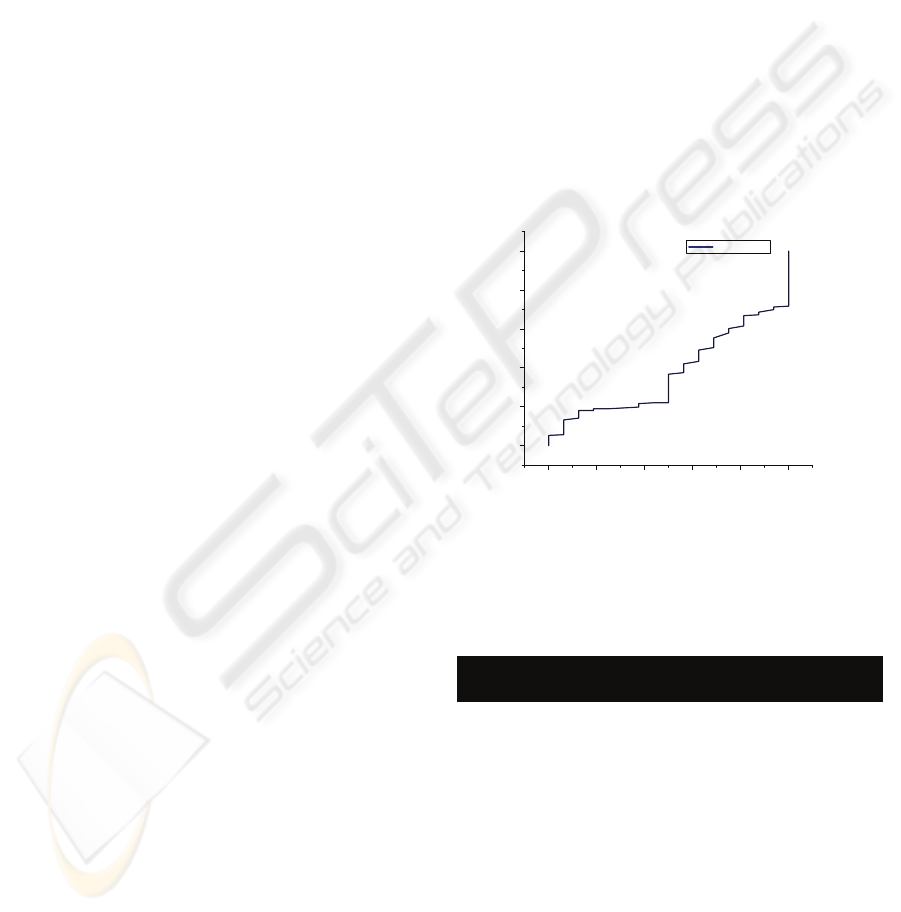
The Daubechies wavelet db5 from Daubechies
(Thompson et. al, 1996; McKeown et. al, 2002) has
been used with 5 decomposition levels. The wavelet
coefficients are roughly classified into two different
classes: a burst zone where artefacts and myoelectric
signals coexist and an inter-burst zone where only
artefact contribution is present. By using hard
thresholding the high-frequency components are set
to zero. In cases where there is no artefact superim-
posed to the myoelectric signal and associated
MUAP’s potentials, the coefficients are supposedly
lower so they will be set to zero with higher prob-
ability. The noisy components of the wavelet de-
composition are truncated and the signal is recon-
structed from the remaining components, addition-
ally the MUAP’s mapping feature with adaptive
wavelets reflects an accurate definition of pre and
post-firing interval identification with related
movement of the subjects (McKeown et. al, 2002;
Fang et. al, 1999).
7 ROC PERFORMANCE
ANALYSIS
As EMG signal inherited a vast number of noise
interference, this will affect result of clustering and
then need to characterize EMG sensor itself for
calibration and buffering purpose. Receiver operat-
ing characteristics (ROC) curve, is analysis of sensor
signals clustering were it is calculated through re-
peatable EMG recording which tend to be classified
in a specific classification algorithm (Jung et. al,
2001). The robustness of wavelet/k-mean algorithm
was tested in contrast to the amplifier gain of inter-
face (Andrzej Cichocki and Shun-ichi Amari, 2003)
.
Intramuscular EMG electrode which is used in clini-
cal experiment is reusable and composed of lined
conductive area used for increasing measurement
stability and reduction of parasitic noise associated
with physiological measurement session. The defini-
tion of sensitivity and selectivity with ROC analysis,
have the following criteria for recursive data cluster-
ing and pattern classification of biomedical and
clinical data.
As table 1. illustrated that the average efficiency
of classified MUAP’s potential in related EMG
signal , the obvious maximum asymptotic properties
Ω
EMG
(t) of 0.97716 and of minimum one of
0.011208 and this reflects high contrast between the
recorded EMG potential ,in which can be considered
as differentiated parameters in classifying associated
MUAP’s signal. For further investigation of this
effect, additional analysis was applied to the classi-
fied EMG signals using the non-negative matrix
decomposition after a k-mean clustering stage, in
which a relevant result of the EMG classification
shows the approximated results in relation to the
MUAP’s intensity. A performance test was applied
to the 9 clustered patterns, by which illustrate that,
the same maximum and minimum asymptotic prob-
abilities for the verified EMG patterns, which in
corresponding 25-test pattern that presented only a 3
EMG-MUAP’s pattern with relevant high voltage
intensity (A. J. Bell and T. J. Sejnowski, 1995),
(Kadefors et. al, 1999).
As observed from the performance index of
adaptive wavelet decomposition could be noticed a
well discriminated EMG pattern such as low firing
contraction, mid –firing contraction , and high firing
contraction and other elated MUAP’s biopotential
action signals associated with muscular fibers firing
schemes (Andrzej Cichocki and Shun-ichi Amari,
2003), (Micera et. al, 2001).
0.0 0.2 0.4 0.6 0.8 1.0
0.0
0.2
0.4
0.6
0.8
1.0
Sensitivity of EMG electrode
Specificity of EMG pattern
ROC curve
Figure 5: ROC curves analysis of 24 EMG pattern using k-
mean clustering algorithm after wavelet decomposition of
MUAP intensity patterns.
Table 1: ROC curve analysis of EMG signal patterns
based on wavelet k-mean clustering technique*.
EMG
pattern*
Area
Under
curve
Std.
Error
SE
Asymptotic
Prob
Ω(EMG)
95.%
LCL
95.%
UCL
EMG1
0.23013 0.1632 0.1083 -0.08975 0.55
EMG2
0.09751 0.29079 0.16498 -0.47243 0.66745
EMG3
0.3714 0.08492 0.08572 0.20496 0.53785
EMG4
0.5083 0.49786 0.97716 -0.46749 1.48409
EMG5
0.60189 0.19039 0.48479 0.22873 0.97505
EMG6
0.49024 0.23233 0.95367 0.03489 0.94559
EMG7
0.12033 0.32535 0.19027 -0.51734 0.758
EMG8
0.53122 0.19993 0.81122 0.13937 0.92307
EMG9
0.33194 0.20502 0.31729 -0.0699 0.73377
EMG10
0.59129 0.4909 0.75282 -0.37085 1.55342
EMG11
0.51452 0.49979 0.96004 -0.46505 1.49409
EMG12
0.09066 0.1331 0.01486 -0.17021 0.35152
EMG13
0.2125 0.24776 0.16159 -0.2731 0.6981
EMG14
0.57797 0.09307 0.42753 0.39556 0.76038
EMG15
0.73418 0.10483 0.07323 0.52872 0.93964
EMG16
0.28801 0.15334 0.20715 -0.01253 0.58854
EMG17
0.48729 0.05748 0.91535 0.37463 0.59995
EMG18
0.03942 0.19281 0.11208 -0.33849 0.41732
*18 subject were tested in the vicinity of ROC curve analysis.
BIOSIGNALS 2009 - International Conference on Bio-inspired Systems and Signal Processing
494