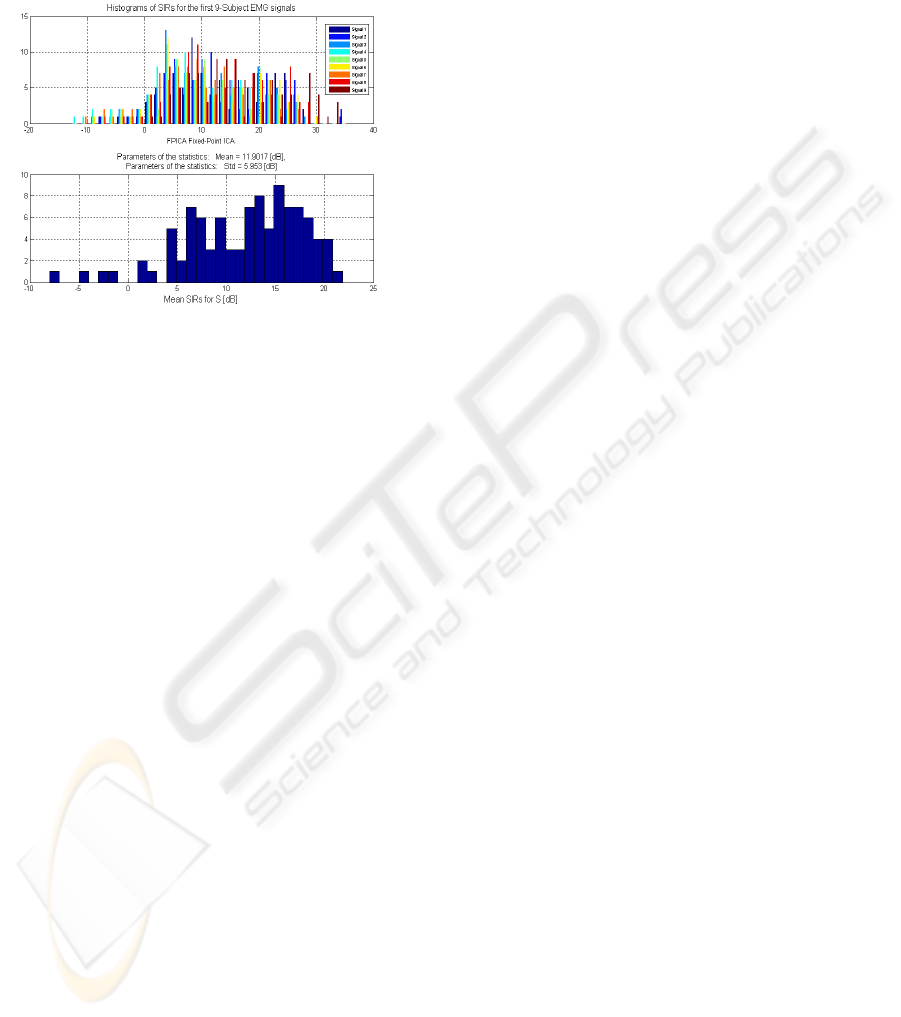
instead of relatively negative value, which mean an
inhibited EMG intensity due to the transition effect
of depolarization wave through the muscle fibers, in
which this obviously considered as a good marked
triggering for online EMG acquisition and diagnosis.
Figure 8: Histogram of ICA decomposed EMG signals
based on fixed-point adapted ICA algorithm.
8 CONCLUSIONS
Pattern clustering and decomposition based on adap-
tive ICA will improve diagnostic performance of
different neuromuscular pathology patterns. EMG
consisted of activity for six different principal pat-
terns which are identified and extracted. The clus-
tered EMG activities notated as (E1,E2,
E3,M1,M2,M3) , E-related to eccentric myoelectric
activity which dominated in near-surface electrode
and M pattern which related to myofibers compart-
ment, each of which have been classified for a 9
subject group. Source localization and identification
are robustly computer through ICA template algo-
rithm, although some pattern in EMG signal the
algorithm were not detected or classified, this due to
the some leakage in optimization cycle of EMG
signals inside ICA algorithm. The overall behavior
of adaptive ICA classifier predicted a considerable
percentage of poor to moderated classified intensity
modulated EMG signals and this due to invalid clas-
sified or decomposed respective EMG coefficient,
which indeed needs improvement for advanced
research and work on EMG pattern classification
optimization. Future perspective of EMG pattern
clustering was introduced, which may use for devel-
oping a cutting edge electrophysiology module. ICA
technique will assist in developing a robust portable
clinical system, by which acquisition, processing,
and diagnosis for several myopathic and neuropathic
EMG pattern could be achieved.
ACKNOWLEDGEMENTS
We acknowledge Aachen University of Applied
Sciences, RWTH-Aachen University and DAAD
(Deutsche Akademische Ausländische Dienst) for
providing accessibility with financial and scientific
support to put this work on the track of success.
REFERENCES
Zazula, D. (1999). Higher-order statistics used for decomposi-
tion of sEMGs. Proceedings of the 12th IEEE Symposium
on Computer Based Medical System, 72–77.
Wang, R., C. Huang, and B. Li (1997). A neural network-
based surface electromyography motion pattern classifier
for the control of prostheses. Proceedings of the 19
th
An-
nual International Conference of the IEEE-EMBS 1277.
Thompson, B., P. Picton, and N. Jones (1996). A comparison
of neural network and traditional signal processing tech-
niques in the classification of EMG signals. IEEE Collo-
quium on Artificial Intelligence Methods for Biomedical
Data Processing , pp 312-319.
McKeown, M. J., Torpey, D.C., Gehm W. C.: Non-Invasive
Monitoring of Functionally Distinct Muscle Activations
during Swallowing. Clinical Neurophysiology (2002).
109, 112
McKeown, M. J.: Cortical activation related to arm move-
ment combinations. Muscle Nerve. 9:19-25 (2000). 110,
112
Jung T.P., Makeig S., McKeown M. J., Bell A. J., Lee T.W.,
Sejnowski T. J.: Imaging brain dynamics using indepen-
dent component analysis. Proc. IEEE. 89(7): 1107-22,
(2001). 112
Andrzej Cichocki,
Shun-ichi AMARI, “ Adaptive Blind
Signal and Image Processing: Learning Algorithms and
Applications”, Wiley Press ,2003.
Bell A. J., Sejnowski T. J.: An information-maximization
approach to blind separation and blind deconvolution,
Neural Computation, 7:1129-1159, (1995). 112
Micera S.,Vannozzi G., Sabatini A.M., Dario P.: Improving
Detection of Muscle Activation intervals, IEEE Engineer-
ing in Medicine and Biology, vol. 20 n.6:38-46 (2001).
112, 113
Roberto Merletti , Philip Parker ," Electromyography Physi-
ology, Engineering, and Noninvasive Applications",
IEEE Press Engineering in Medicine and Biology Socie-
ty, 2004, IEEE press, pp 234-256
Farina, D., and R. Merletti, “A novel approach for precise
simulation of the EMG signal detected by surface elec-
trodes,” IEEE Trans BME 48, 637–645 (2001).
Karhunen J., Oja E.: A Class of Neural Networks for Inde-
pendent Component Analysis, IEEE Transactions on
Neural Network, vol. 8 n. 3:486-504, (1997). 112.
BIOSIGNALS 2009 - International Conference on Bio-inspired Systems and Signal Processing
310