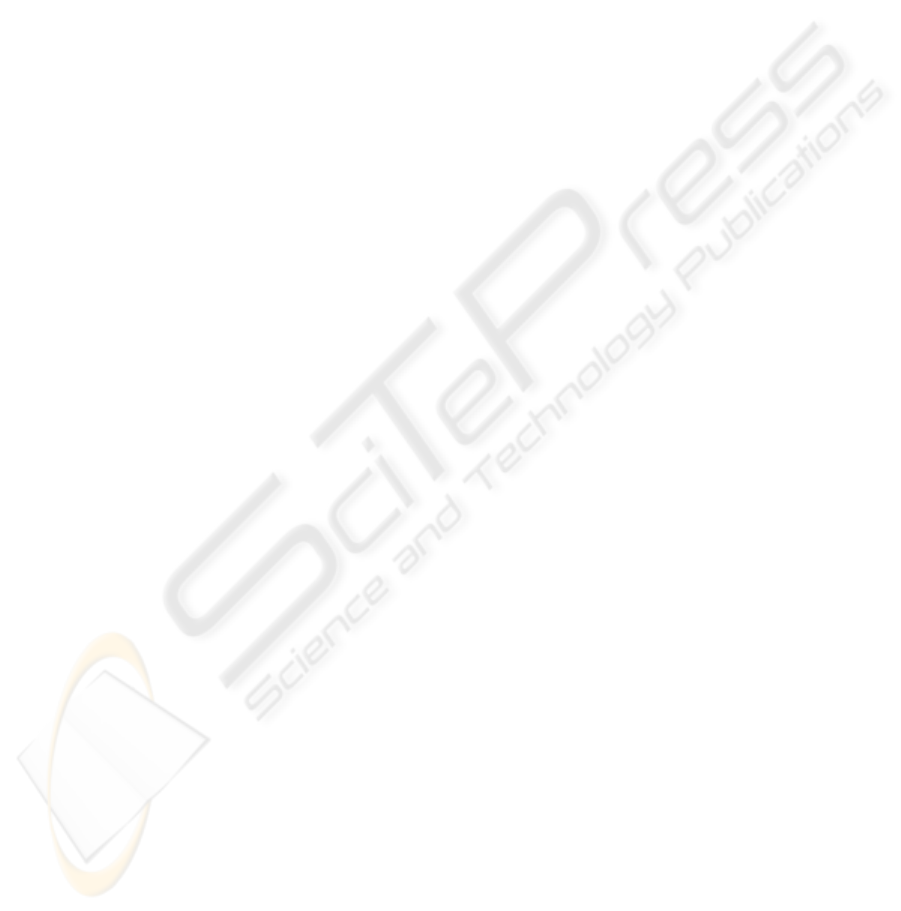
classified and misclassified patterns for quadratic dis-
criminant classifier. This experiment showed that the
DCR and TBM measures are not suitable for using
in aggregation of ensembles of quadratic discriminant
classifiers.
We showed a possible way how local classification
confidence can be used in classifier aggregation. The
performance of these methods was compared to com-
monly used methods for classifier aggregation of an
ensemble of quadratic discriminant classifiers on four
artificial and five real-world benchmark datasets. The
results show that incorporating local classifier confi-
dence into classifier aggregation can bring significant
improvements in the classification quality.
In our future work, we plan to study local clas-
sification confidence measures for other classifiers
than quadratic discriminant classifier, mainly decision
trees and support vector machines, and to incorporate
local classification confidence into more sophisticated
classifier aggregation methods.
ACKNOWLEDGEMENTS
The research presented in this paper was partially sup-
ported by the Program “Information Society” under
project 1ET100300517 (D.
ˇ
Stefka) and by the Institu-
tional Research Plan AV0Z10300504 (M. Hole
ˇ
na).
REFERENCES
Aksela, M. (2003). Comparison of classifier selection meth-
ods for improving committee performance. In Multi-
ple Classifier Systems, pages 84–93.
Avnimelech, R. and Intrator, N. (1999). Boosted mixture of
experts: An ensemble learning scheme. Neural Com-
putation, 11(2):483–497.
Bay, S. D. (1999). Nearest neighbor classification from
multiple feature subsets. Intelligent Data Analysis,
3(3):191–209.
Breiman, L. (1996). Bagging predictors. Machine Learn-
ing, 24(2):123–140.
Cheetham, W. and Price, J. (2004). Measures of Solu-
tion Accuracy in Case-Based Reasoning Systems. In
Calero, P. A. G. and Funk, P., editors, Proceedings
of the European Conference on Case-Based Reason-
ing (ECCBR-04), pages 106–118. Springer. Madrid,
Spain.
Delany, S. J., Cunningham, P., Doyle, D., and Zamolot-
skikh, A. (2005). Generating estimates of classifi-
cation confidence for a case-based spam filter. In
Mu
˜
noz-Avila, H. and Ricci, F., editors, Case-Based
Reasoning, Research and Development, 6th Interna-
tional Conference, on Case-Based Reasoning, ICCBR
2005, Chicago, USA, Proceedings, volume 3620 of
LNCS, pages 177–190. Springer.
Duda, R. O., Hart, P. E., and Stork, D. G. (2000). Pattern
Classification (2nd Edition). Wiley-Interscience.
Freund, Y. and Schapire, R. E. (1996). Experiments with a
new boosting algorithm. In International Conference
on Machine Learning, pages 148–156.
Hand, D. J. (1997). Construction and Assessment of Clas-
sification Rules. Wiley.
Kittler, J., Hatef, M., Duin, R. P. W., and Matas, J. (1998).
On combining classifiers. IEEE Trans. Pattern Anal.
Mach. Intell., 20(3):226–239.
Kuncheva, L. I. (2004). Combining Pattern Classifiers:
Methods and Algorithms. Wiley-Interscience.
Kuncheva, L. I., Bezdek, J. C., and Duin, R. P. W.
(2001). Decision templates for multiple classifier fu-
sion: an experimental comparison. Pattern Recogni-
tion, 34(2):299–314.
Newman, D., Hettich, S., Blake, C., and Merz, C.
(1998). UCI repository of machine learning databases.
http://www.ics.uci.edu/∼mlearn/MLRepository.html.
Robnik-
ˇ
Sikonja, M. (2004). Improving random forests.
In Boulicaut, J., Esposito, F., Giannotti, F., and Pe-
dreschi, D., editors, ECML, volume 3201 of Lecture
Notes in Computer Science, pages 359–370. Springer.
Tsymbal, A., Pechenizkiy, M., and Cunningham, P.
(2006). Dynamic integration with random forests. In
Frnkranz, J., Scheffer, T., and Spiliopoulou, M., edi-
tors, ECML, volume 4212 of Lecture Notes in Com-
puter Science, pages 801–808. Springer.
UCL MLG (1995). Elena database.
http://www.dice.ucl.ac.be/mlg/?page=Elena.
Wilson, D. R. and Martinez, T. R. (1999). Combining
cross-validation and confidence to measure fitness. In
Proceedings of the International Joint Conference on
Neural Networks (IJCNN’99), paper 163.
Woods, K., W. Philip Kegelmeyer, J., and Bowyer, K.
(1997). Combination of multiple classifiers using lo-
cal accuracy estimates. IEEE Trans. Pattern Anal.
Mach. Intell., 19(4):405–410.
Zhu, X., Wu, X., and Yang, Y. (2004). Dynamic classifier
selection for effective mining from noisy data streams.
In ICDM ’04: Proceedings of the Fourth IEEE In-
ternational Conference on Data Mining (ICDM’04),
pages 305–312, Washington, DC, USA. IEEE Com-
puter Society.
ICAART 2009 - International Conference on Agents and Artificial Intelligence
178