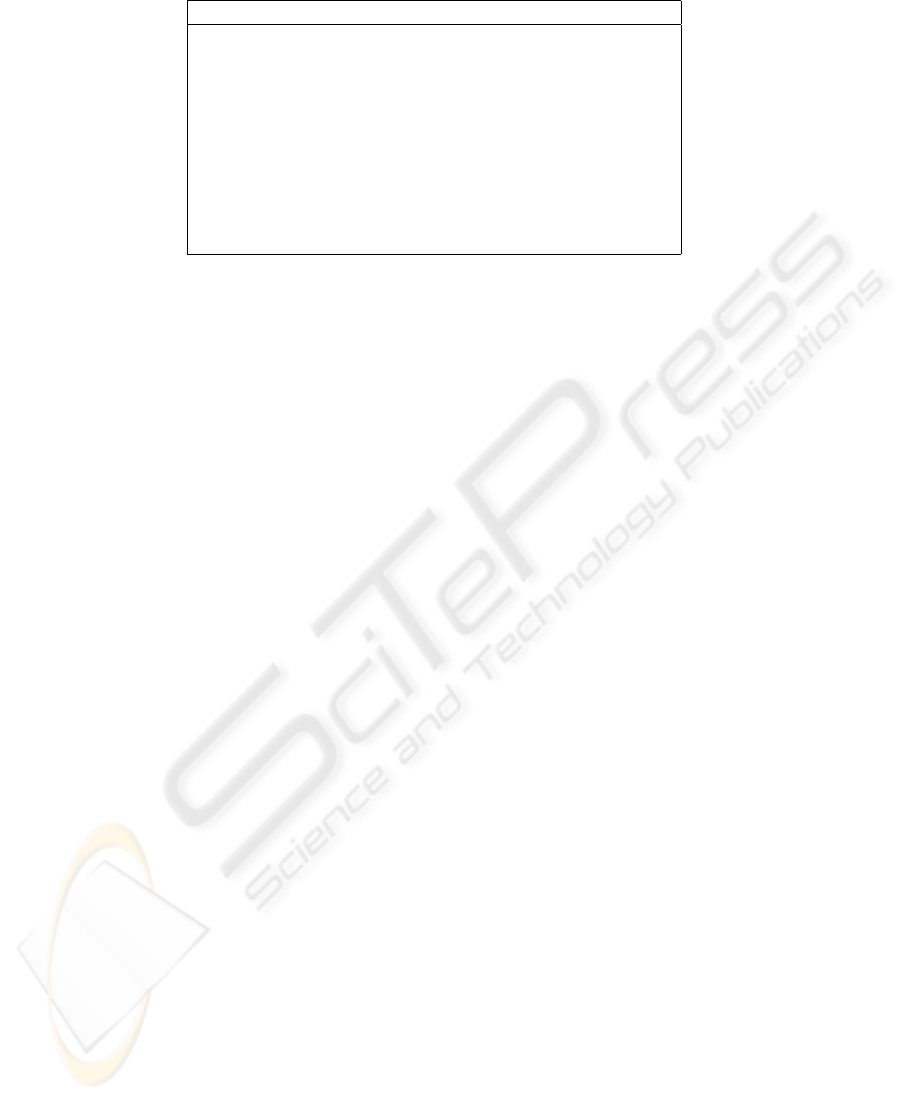
Table 4: The top 10 ranked variables for diverticulitis vs. non-specific abdominal pain.
Variable Weight
Age 1.51921
C-reactive protein level 1.12222
Initial pain localization; left lower quadrant 1.03731
Current pain localization; lower left quadrant 0.944773
Tenderness on palpation; left lower quadrant 0.885332
Development of pain intensity; increase 0.762057
Current pain localization; right upper quadrant 0.677884
Local muscular defence 0.674493
Leukocyte level 0.664661
Initial pain localization; right upper quadrant 0.657553
gories with inherent similarities and differences, as
was evidenced by the findings in our study. The one-
against-all scheme, on the other hand, is more relevant
to the problem of diagnosis.
The class imbalance was adjusted by simple
under-sampling of the majority class. More sophis-
ticate methods could be employed to this end, such as
the SMOTE algorithm (Chawla et al., 2002).
The simple feature ranking and subsequent selec-
tion utilized in this study proved to be effective in
boosting classifier performance. However, more suit-
able approaches can be used to obtain optimal vari-
able subsets, such as evolutionary algorithms (Mar-
chiori et al., 2007;
˚
Aberg et al., 2008). Moreover,
there is reason to believe that non-linear relation-
ships pertaining to the disease category exist between
some parameters, and reducing the complexity of the
data structures can potentially allow for better perfor-
mance with non-linear classifiers (
˚
Aberg and Wess-
berg, 2007).
5 CONCLUSIONS
Automatic computer-based disease classification is a
promising tool for the diagnosis of acute abdominal
pain, but requires substantial research before a clin-
ical implementation is feasible. The support vector
machine is highly suitable for the discrimination be-
tween binary disease categories, and achieved results
comparable to the medical doctor. Moreover, the clas-
sifier obtained higher sensitivity and specificity than
the physician in the distinction between diverticulitis
and non-specific abdominal pain. Age and C-reactive
protein level, as well as left-hand side pain sensations,
were identified as important factors for the classifica-
tion of diverticulitis.
REFERENCES
˚
Aberg, M. C., L
¨
oken, L., and Wessberg, J. (2008). An evo-
lutionary approach to multivariate feature selection for
fMRI pattern analysis. Proceedings of the Interna-
tional Conference on Bio-inspired Systems and Signal
Processing.
˚
Aberg, M. C. and Wessberg, J. (2007). Evolutionary op-
timization of classifiers and features for single trial
EEG discrimination. BioMedical Engineering Online,
6(32).
Adams, I. D., Chan, M., Clifford, P. C., Cooke, W. M.,
Dallos, V., de Dombal, F. T., Edwards, M. H., Han-
cock, D. M., Hewett, D. J., and McIntyre, N. (1986).
Computer aided diagnosis of acute abdominal pain: a
multicentre study. British Medical Journal (Clinical
research ed.), 293(6550):800–804.
Ambrosetti, P., Robert, J., Witzig, J., Mirescu, D., Mathey,
P., Borst, F., and Rohner, A. (1994). Acute left colonic
diverticulitis: a prospective analysis of 226 consecu-
tive cases. Surgery, 115(5):546–50.
Bellman, R. E. (1961). Adaptive Control Processes. Prince-
ton University Press, Princeton, NJ.
Blum, A. and Langley, P. (1997). Selection of relevant fea-
tures and examples in machine learning. Artificial In-
telligence, 97(1-2):245–271.
Chawla, N., Bowyer, K., Hall, L., and Kegelmeyer, W.
(2002). Smote: synthetic minority over-sampling
technique. Journal of Artificial Intelligence Research,
16:321357.
de Dombal, F., Leaper, D., Staniland, J., McCann, A.,
and Horrocks, J. (1972). Computer-aided diagnosis
of acute abdominal pain. British Medical Journal,
2(5804):9–13.
Ferzoco, L., Raptopoulos, V., and Silen, W. (1998). Acute
diverticulitis. The New England Journal of Medicine,
338(21):1521–6.
Hansson, L.-E. (2002). Akut Buk. Lund: Studentlitteratur.
Laurell, H., Hansson, L., and Gunnarsson, U. (2006). Acute
abdominal pain among elderly patients. Gerontology,
52(6):339–44.
HEALTHINF 2009 - International Conference on Health Informatics
56