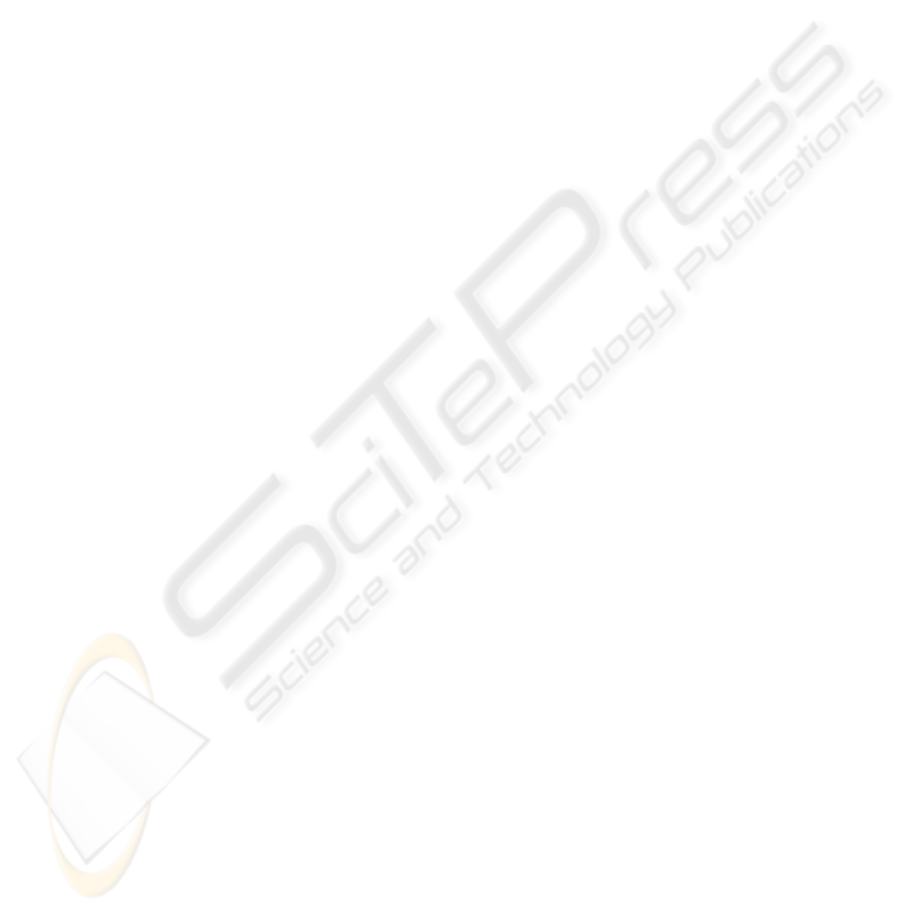
ACKNOWLEDGEMENTS
S¨uleyman Baykut’s work is supported by “The
Scientific and Technological Research Council of
Turkey-The Department of Science Fellowships and
Grant Programmes (TUBITAK-BIDEB)” with the
programme # 2214.
REFERENCES
Cohen, J. (1960). A coeeficient of agreement for nominal
scales. In Educational and Psychological Measure-
ment. Vol. 20, pp. 37-46.
Corsi-Cabrera, M., P´erez-Garci, E., Rio-Portilla, Y. D.,
Ugalde, E., and Guevara, M. A. (2001). EEG bands
during wakefulness, slow-wave, and paradoxical sleep
as a result of principal component analysis in the rat.
In Sleep. Vol. 24, No. 4.
Estrada, E., Nazeran, H., Nava, P., Behbehani, K., Burk,
J., and Lucas, E. (2004). EEG feature extraction for
classification of sleep stages. In Proc. of the 26th An-
nual International Conference of the IEEE EMBS. San
Francisco, USA.
Flandrin, P., Rilling, G., and Goncalves, P. (2003). Empiri-
cal mode decomposition as a filter bank. In IEEE Sig.
Proc. Lett. Vol. 11, No. 2, Part 1, pp. 112- 114.
Gervasoni, D., Lin, S., Ribeiro, S., Soares, E., Pantoja, J.,
and Nicolelis, M. (2004). Global forebrain dynam-
ics predict rat behavioral states and their transitions.
In The Journal of Neuroscience. Vol. 24, No. 49, pp.
11137-11147.
Goeller, C. J. and Sinton, C. M. (1989). A microcomputer-
based sleep stage analyser. In Computer Methods and
Programs in Biomedicine. Vol. 29, No. 1, 316.
Gottesmann, C., Lacoste, G., Rodrigues, L., Kirkham, P.,
Arnaud, C., and Rallo, J. L. (1976). Method of auto-
matic analysis and quantification of wakefulness-sleep
behavior in the rat. In Rev. Electroencephalogr. Neu-
rophysiol. Clin. Vol. 6, pp. 37-49.
Hese, P. V., Philips, W., Koninck, J. D., de Walle, R. V.,
and Lemahieu, I. (2001). Automatic detection of sleep
stages using the EEG. In Proc. of the 23rd Annual
International Conference of the IEEE EMBS. Istanbul,
Turkey.
Huang, N., Shen, Z., Long, S., Wu, M., Shih, H., Zheng,
Q., Yen, N. C., Tung, C. C., and Liu, H. (1998). The
empirical mode decomposition and hilbert spectrum
for nonlinear and nonstationary time series analysis.
In Proc. Roy. Soc. London A. Vol. 454, pp. 903-995.
Neckelmann, D., Olsen, O. E., Fagerland, S., and Ursin,
R. (1994). The reliability and functional validity of
visual and semi-automatic sleep:wake scoring in the
moll-wistar rat. In Sleep. Vol. 17, No. 2, pp. 12031.
Ning, T., Joseph, D., and Bronzino, D. (1990). Bispectral
analysis of the rat EEG during REM sleep. In Proc.
of the Annual International Conference of the IEEE
EMBS. Vol. 12, No. 5.
Rilling, G., Flandrin, P., and Goncalves, P. (2003). On em-
pirical mode decomposition and its algorithms. In
IEEE-EURASIP Workshop on Nonlinear Signal and
Image Processing NSIP-03. Grado.
Robert, C., Guilpin, C., and Limoge, A. (1999). Automated
sleep staging systems in rats. In Journal of Neuro-
science Methods. Vol. 88, No.2, pp. 111-122.
Ruigt, G. S. F., Proosdij, J. N. V., and Delft, A. M. L. V.
(1989). A large scale, high resolution, automated sys-
tem for rat sleep staging i. In Methodology and techni-
cal aspects. Electroencephalogr. Clin. Neurophysiol.
Vol. 73, pp. 52-63.
Sharabaty, H., Martin, J., Jammes, B., and Esteve, D.
(2006). Alpha and theta wave localisation using
hilbert-huang transform: Empirical study of the ac-
curacy. In Proc. of IEEE Information and Communi-
cation Technologies-ICTTA. Vol. 1, pp. 1159-1164.
Timo-Iaria, C., Negrao, N., Schmidek, W. R., Hoshino, K.,
de Menezes, C. E. L., and da Rocha, T. L. (1970).
Phases and states of sleep in the rat. In Physiol. Behav.
Vol. 5, pp. 1057-1062.
Torres, A., Fiz, J. A., Jan´e, R., Galdiz, J. B., Gea, J., and
Morera, J. (2007). Application of the empirical mode
decomposition method to the analysis of respiratory
mechanomyographic signals. In Proc. of the 29th
Annual International Conference of the IEEE EMBS.
Lyon, France.
Vivaldi, E. A. and Bassi, A. (2006). Frequency domain
analysis of sleep EEG for visualization and automated
state detection. In Proc. of the 28th Annual Interna-
tional Conference of the IEEE EMBS. New York City,
USA.
Vyazovskiy, V. V., Borb´ely, A. A., and Tobler, I. (2002).
Interhemispheric sleep EEG asymetry in the rat is en-
hanced by sleep deprivation. In J. Neurophysiology.
Vol. 88, pp. 2280-2286.
Winson, J. (1974). Patterns of hippocampal theta rhythm
in the freely moving rat. In Electroencephalogr. Clin.
Neurophysiol. Vol. 36, pp. 291-301.
Ziqiang, Z. and Puthusserypady, S. (2007). Analysis of
schizophrenic EEG synchrony using empirical mode
decomposition. In Proc. of 15th Int. Conference on
Digital Signal Processing. pp. 131-134, Cardiff, Eng-
land.
EMD-BASED ANALYSIS OF RAT EEG DATA FOR SLEEP STATE CLASSIFICATION
123