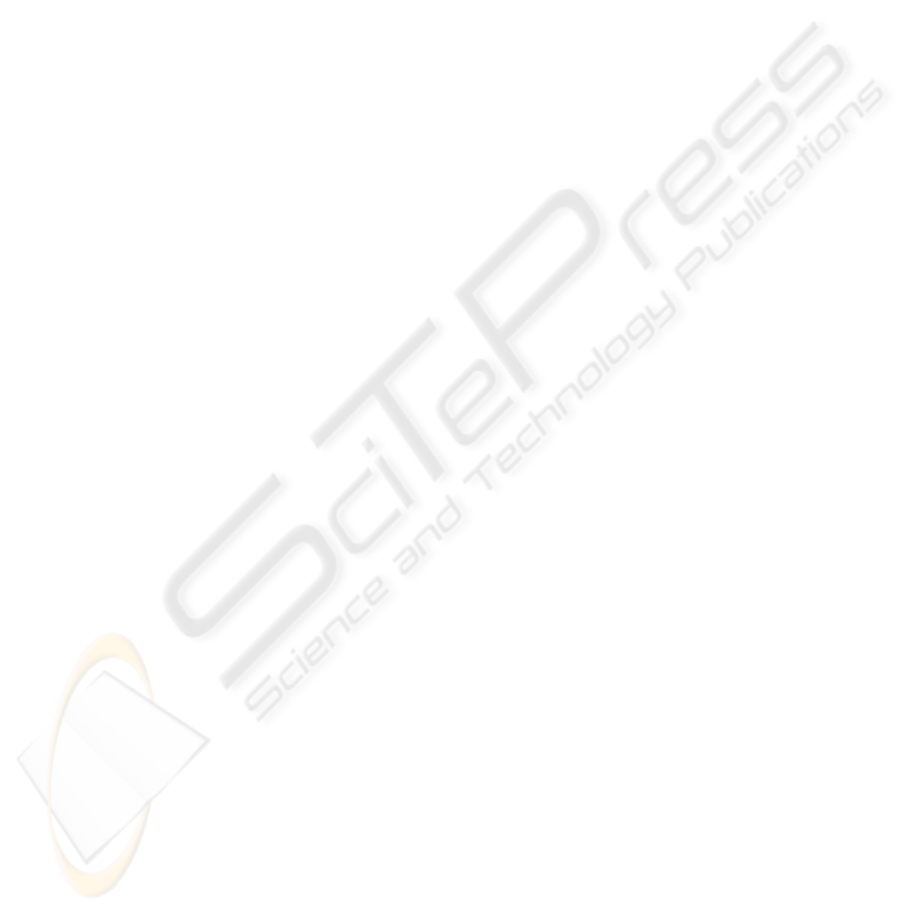
The performance of a given network setting was
measured using two consecutive criteria. The first
welcomes the network that obtained the highest hit
rate of patients with retinal NFL dysfunction, since
the main goal is the correct classification of patients
with an indication of glaucoma. The second criterion
is the highest hit rate of the overall, healthy or
pathological patients, among the best networks
obtained by the first criterion.
The best overall hit rate was 92.19% and it was
obtained with a MLP network with 7 entries, the
data decomposed into its principal components, and
the variables selected by the LSE method. High
performance (90.63%) was also achieved with MLP
neural networks and the variables selected by the
LSE method. The transformation of the database
into its principal components seems not to have
substantially improved the performance of networks.
Various configurations of networks have hit rates
above 84% in patients with an indication of
glaucoma, correctly classifying 16 patients in a total
of 19. All networks that have this kind of
performance were MLP, and therefore one can say
that generally the performance of networks MLP
was higher than of PNN networks in this application.
The LSE method has proved to be very
consistent, making explicit that from 10 variables,
not considering the ones related to retinal NFL
thickness, 4 have much relevance and allow
classification with a hit rate of over 89%: rim width,
excavation diameter, disk diameter and average
width of nerve. The result of the networks shows
that there is a clear link between these 4 variables
and an amendment of retinal NFL that may suggest
the presence of glaucoma, according to the pattern of
this amendment.
According to the specialist, these results are very
interesting. In glaucoma, there is really a loss of
preferential rim nerve in superior and inferior poles,
and so it is consistent the involvement of these
variables in the definition of retinal NFL
dysfunction.
4 CONCLUSIONS
In ophthalmologic exams, the detection of optic
nerve damage by glaucoma involves morphological
characteristics, like size and shape of rim and the
excavation of the disk, which were pointed out as an
important feature in the results. It is known that
these characteristics vary with the size of the disk
(Hoffman et al, 2007).
Moreover, the measure of the disk size varies
with the technique used, among populations. Often it
is easier to detect suggestive changes of
glaucomatous damaged nerves with a larger size
compared to the small disks. This characteristic
affects the likelihood of a doctor to make the
diagnosis, being a major factor of bias, which
characterizes the complexity of the process involved.
According to most specialists, it is not possible
to make the diagnosis of glaucoma without
characteristics changes in the visual field, which
represents a significant loss of neural tissue, in
which functional injuries are most often irreversible.
Therefore, researchers have pursued ways to find
the changes that precede these symptoms for a
diagnosis and early treatment of glaucoma. Such
searches are based, most of them, on morphological
changes of the optical disk and the layer of nerve
fibres, as detected by the OCT.
The neural network can help the doctor in the
diagnosis since these physical measures of disk are
mathematically related, so it is possible to correlate
them and generate an output profile of the
pathology. In this study, through the use of neural
networks, there was a significant hit rate of this
pathological profile characterized by changes in
retinal NFL, which suggests presence of glaucoma.
As discussed in the previous section, it was
expected that the variable width of rim, excavation
diameter, disk diameter and average width of nerve
were relevant to the indication of glaucoma.
However, other studies are still needed to understand
the complex relationship between size of disk,
neural tissue, demographic factors and the
development of glaucoma.
The suppression of the 4 input variables related
to NFL thickness in the second experiment did not
prevent the high performance of networks using the
other variables. The hit rates in patients with
dysfunction in retinal NFL were still satisfactory.
Therefore, this work provided an important result
as it proved that it is possible to detect the presence
of retinal NFL dysfunction, or at least simulate the
result of the statement obtained on OCT, using
neural networks fed by variables that can be
obtained by different and cheaper exams.
Other classification methods besides ANN could
be tested with the available database, such as support
vector machines (Cristianini, 2000) (Haykin, 1999)
and hybrid neuro-fuzzy models (Gonçalves et al,
2006) (Vellasco et al 2007) (which would also allow
one to extract fuzzy rules that could generate
linguistic rules over the classification problem).
However, in this work we chose the ANN models to
BIOSIGNALS 2009 - International Conference on Bio-inspired Systems and Signal Processing
144