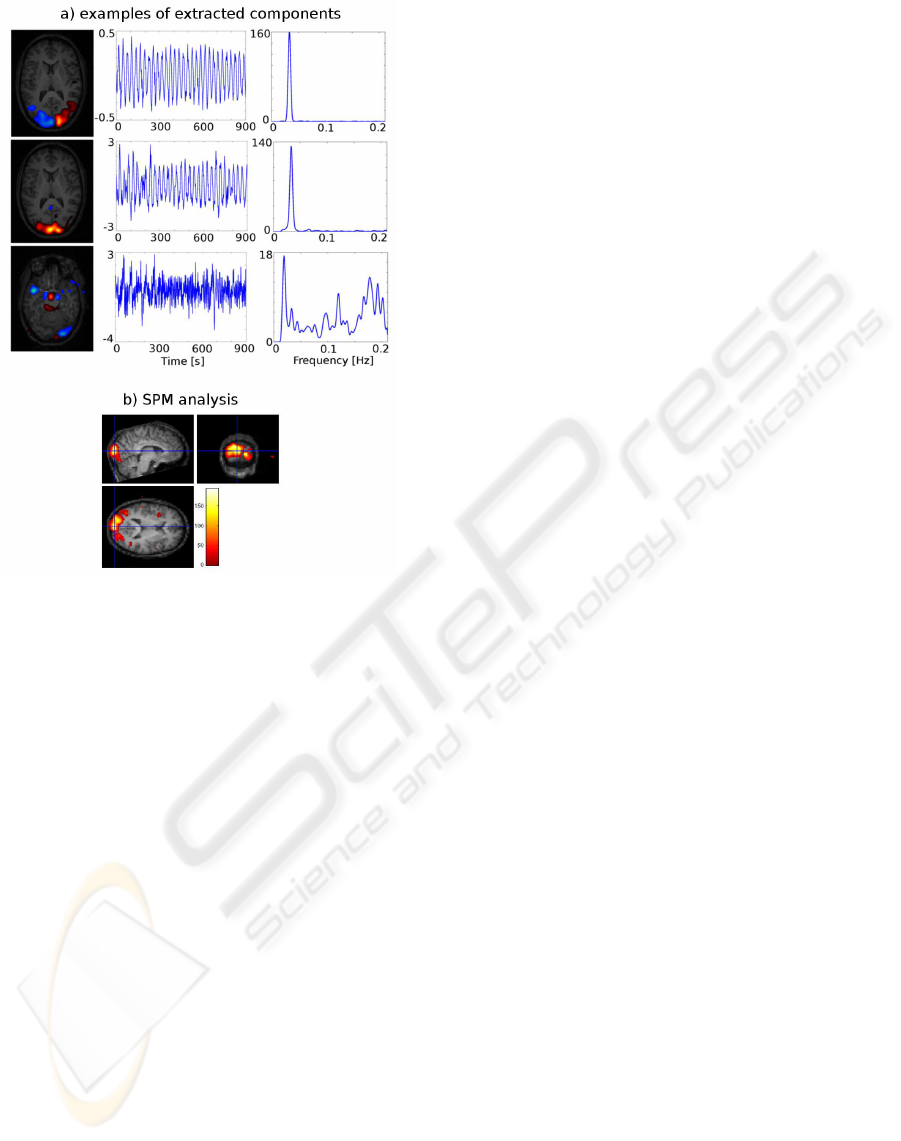
Figure 3: Top; Three selected components from ICA per-
formed on the demonstration dataset. To the left the spatial
map from each component is showed as an overlay on the
anatomical scan. For the first two components a slice near
the calcarine sulcus is shown whereas a slice near the cir-
cle of Willis is shown for the last component. The middle
column of figures shows the temporal profile for each of the
components and the right column shows the power spec-
trum (Welch method). The first two components are clearly
related to the visual paradigm with prominent activity in the
occipital cortex whereas the last component is related to car-
diac nuisance effects. Buttom; F-test conducted in SPM5
on test data set for significant effects of paradigm related
ICs identified in the training data set. Thresholded at p <
0.001 uncorrected.
REFERENCES
Bell, A. and Sejnowski, T. (1995). An information-
maximization approach to blind separation and blind
deconvolution. Neural Computation, 7:1129–1159.
Cardoso, J.-F. (1999). High-order contrasts for independent
component analysis. Neural Comput., 11(1):157–192.
Correa, N., Adali, T., and Calhoun, V. (2007). Performance
of blind source separation algorithms for fmri analy-
sis using a group ica method. Magnetic Resonance
Imaging, 25(5):684–694.
Frackowiak, R., Friston, K., Frith, C., Dolan, R., Price, C.,
Zeki, S., Ashburner, J., and Penny, W. (2003). Human
Brain Function. Academic Press, 2nd edition.
Hansen, L., Larsen, J., and Kolenda, T. (2001a). Blind de-
tection of independent dynamic components. Acous-
tics, Speech, and Signal Processing, 2001. Proceed-
ings. (ICASSP ’01). 2001 IEEE International Confer-
ence on, 5:3197–3200.
Hansen, L., Larsen, J., and Kolenda, T. (2001b). Blind de-
tection of independent dynamic components. Acous-
tics, Speech, and Signal Processing, 2001. Proceed-
ings.(ICASSP’01). 2001 IEEE International Confer-
ence on, 5.
Hansen, L., Larsen, J., Nielsen, F., Strother, S., Rostrup, E.,
Savoy, R., Lange, N., Sidtis, J., Svarer, C., and Paul-
son, O. (1999). Generalizable Patterns in Neuroimag-
ing: How Many Principal Components? Neuroimage,
9(5):534–544.
Højen-Sørensen, P., Winther, O., and Hansen, L. (2002).
Analysis of functional neuroimages using ICA adap-
tive binary sources. Neurocomputing, 49(1):213–225.
Hu, D., Yan, L., Liu, Y., Zhou, Z., Friston, K. J., Tan, C.,
and Wu, D. (2005). Unified spm-ica for fmri analysis.
NeuroImage, 25:746–755.
Hyv¨arinen, A. and Oja, E. (1997). A fast fixed-point al-
gorithm for independent component analysis. Neural
Computation, 9(7):1483–1492.
Hyv¨arinen, A. and Oja, E. (2000). Independent component
analysis: Algorithms and applications. Neural Net-
works, 13(4-5):411–430.
Kolenda, T., Hansen, L., and Larsen, J. (2001). Signal
detection using ICA: Application to chat room topic
spotting. Third International Conference on Indepen-
dent Component Analysis and Blind Source Separa-
tion, pages 540–545.
Lund, T., Madsen, K., Sidaros, K., Luo, W., and Nichols,
T. (2006). Non-white noise in fMRI: Does modelling
have an impact? Neuroimage, 29(1):54–66.
McKeown, M., Hansen, L., and Sejnowsk, T. (2003a). Inde-
pendent component analysis of functional MRI: what
is signal and what is noise? Current Opinion in Neu-
robiology, 13(5):620–629.
McKeown, M., Hansen, L., and Sejnowsk, T. (2003b). Inde-
pendent component analysis of functional mri: what is
signal and what is noise? Current Opinion in Neuro-
biology, 13(5):620–629.
McKeown, M., Makeig, S., Brown, G., Jung, T., Kinder-
mann, S., Bell, A., and Sejnowski, T. (1998). Analysis
of fmri data by blind separation into independent spa-
tial components. Human Brain Mapping, 6(5):160–
188.
Molgedey, L. and Schuster, H. (1994). Separation of
independent signals using time-delayed correlations.
Physical Review Letters, 72(23):3634–3637.
Smith, L. (2002). A tutorial on Principal Components Ana-
lysis. Cornell University, USA, 51:52.
UNIFIED ICA-SPM ANALYSIS OF FMRI EXPERIMENTS - Implementation of an ICA Graphical User Interface for the
SPM Pipeline
321