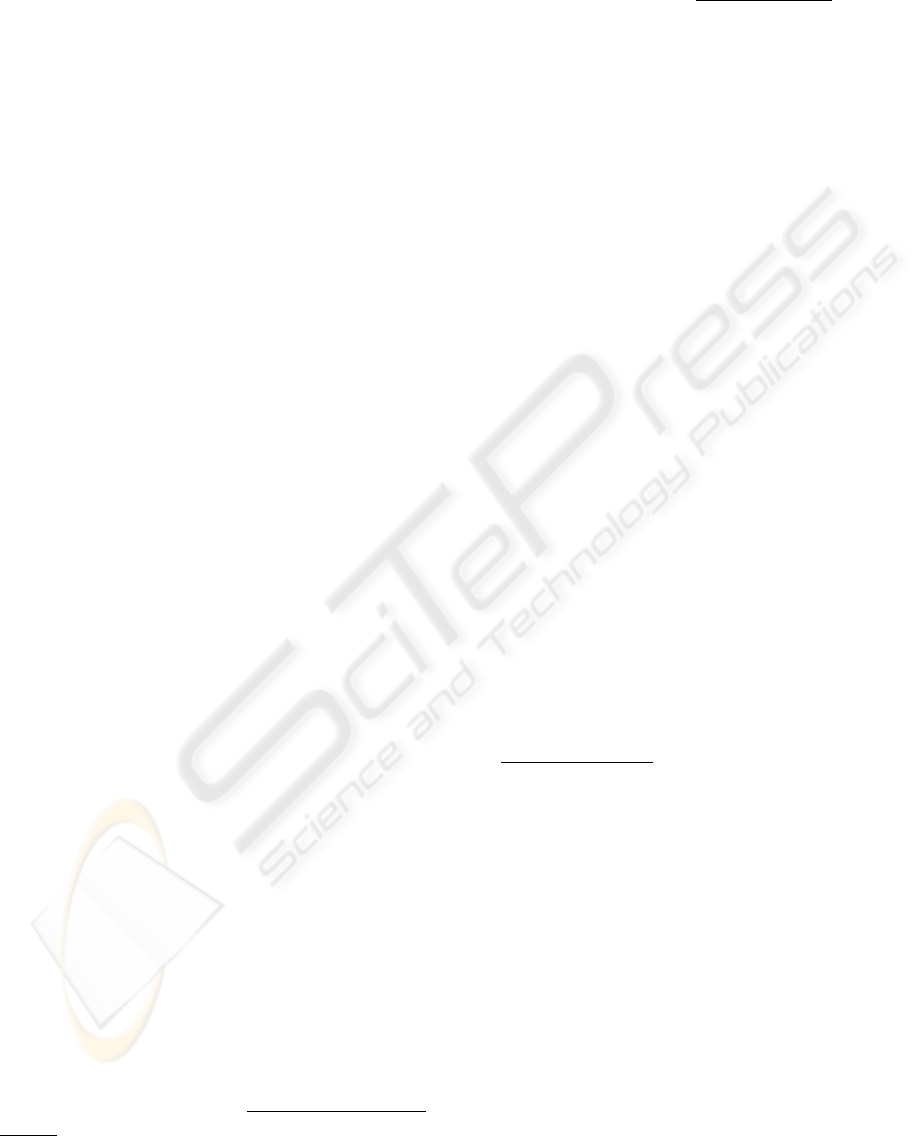
medical area of each course, but even if some
medical semantics is added, the granularity of the
description is still global. It is not appropriate to
adapt on the contents of the courses, taking into
account their semantic and their cultural aspects.
This issue is not faced when using other standards
for educational purpose as LOM, or SCORM. We
wanted to add some contextual indexes on the
courses, so that we can adapt the information. In
particular, cultural difference should be treated.
Moreover, if we are able firstly to provide such
indexes and secondly to capture the context of use
for the resources, we can think about ubiquitous and
pervasive learning resources, able to dynamically
adapt themselves according to the settings in which
they are used. This paper will present the CAPLA
(Contextual Adaptation and Pervasive Learning for
Alzheimer) model for adaptation. Our model is used
to index the variability of the content of medical
courses. This work is part of a French national
project called p-LearNet (pervasive Learning
Network) whose objectives are to explore the human
learning potential, in the framework of pervasive
communication and the use of Technology
Enhanced Learning (TEL). Then will briefly
describe the Alois Software which is proposed for
indexing and re-creating the courses.
2 THE CAPLA MODEL FOR
ADAPTATION
2.1 The CAPLA Needs for Adaptation
We notice there is room for a specific description of
the variability of the resources. When we move from
a context (e.g. Country=France) to another context
(e.g. Country=Mali), pertinent adaptation of the
content are often necessary. In this paper, we will
illustrate it by the “vaccum-cleaner example”: to
detect Alzheimer, during cognitive tests it is not
pertinent to propose to an individual living in the
bush in Mali to recognize a vacuum-cleaner even if a
French resource proposes to do so. The concept of
“recognize a vacuum cleaner” as “an easy task to
do” depends on culture. Introducing such a resource
in a course induces cultural variability. In fact the
need for adaptation is very frequent.
Two different notions must be distinguished.
Variability: it describes criteria which induce
change. For example, each time the notion of the
prevalence of a disease appears in a course, it
induces variability as the prevalence is not the same
everywhere; the related information can depend on a
geographic and/or epidemiological context.
Context: it describes the environment
: when,
who, where an individual is producing/using a
course are some of the obvious context knowledge.
(Example of context description: a course, written
for France, for a general practitioner, the teacher will
use it in an amphitheatre equipped with a data
projector and a PC with high speed broadband
network).
We have made the following hypothesis to
propose our model: (i)Indexing the resources with
variability could help to explicit some knowledge on
these resources (e.g. it’s interesting to notice that
"vacuum-cleaner" is not always easy to understand
according to the culture); (ii) Once a resource is
indexed with variability, it should ease the
adaptation to another context (for example, someone
from Senegal could benefit from the indexation
made for Mali); (iii) The explicit knowledge of
variability AND of context can help to propose a
smart adaptation of the courses (“vacuum cleaner”
as “culturally variable” will be changed for Mali but
not for Belgium);. (iv) pervasive learning can use
such models and be proposed as soon as we are able
to detect the context.
2.2 Variability and Courses
Re-Documentation
With the emergence of the Web2.0 applications,
documents are more and more used in collective
practice. Zacklad focuses on situations where
documents serve to coordination (Zacklad, 2006),
we use his work for our model.
Documentarisation
can be made on this course,
in our case through the annotation of the variability.
“Documentarisation consists of endowing the
substrates with specific attributes making it possible:
(i) to manage them along with other substrates, (ii)
to handle them physically, which is a prerequisite to
be able to browse semantically among the semiotic
content, and lastly, (iii) to guide not only the
recipients, but also the producers themselves to an
increasing extent, around the substrate by providing
one or several maps of the semiotic contents”
(Zacklad, 2006). It doesn’t affect the document. We
use some annotation of the variability to enrich the
knowledge we have on the semiotic content of a
document. In this paper, we focus on the specific
area of our multi-countries e-learning application but
other criteria can come from more general
knowledge. We propose a first typology for the
variability of the Alzheimer’s disease domain,
THE CAPLA MODEL FOR MULTI-CULTURAL ADAPTATION OF LEARNING RESOURCES FOR ALZHEIMER’S
DISEASE
463