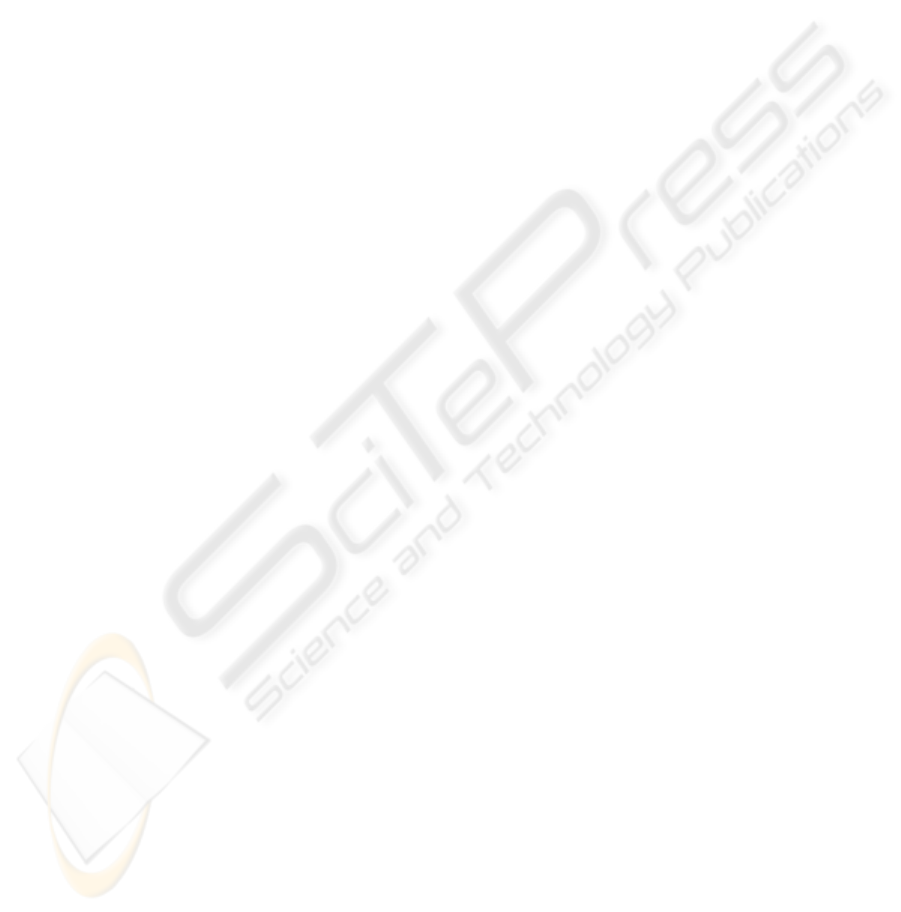
troscopy has been used widely due to ease of sam-
pling using fiber optics, and inexpensive and robust
instrumentation (Cooper et al., 1997; Yano et al.,
1997; Majara et al., 1998; Yeung et al., 1999; Alison
et al., 2000; Rhiel et al., 2002).
UV-VIS spectroscopy has not been used in bio-
process engineering, where the use of near infrared
(NIR) has been preferred due to the association to
specific vibrations of molecular groups (Workman,
1993). It has been widely accepted that vibrational
spectroscopy is more adequate for organic chemistry
measurement than transitional spectroscopy (Faust,
1992). However, NIR spectroscopy has as high in-
convenience the high absorbance of water in this re-
gion, which greatly decreases the sensitivity, being
more difficult to detect metabolites in lower concen-
trations. Furthermore, NIR and MIR equipments are
more expensive, and today’s cheap miniaturized spec-
trometers are capable to detect with high precision in
the UV-VIS-SWNIR region.
UV-VIS-SWNIR has not been used for a fer-
mentation monitoring. This is perhaps attributed to
the fact that UV-VIS spectroscopy records transmi-
tions between electron energy levels from molecu-
lar orbitals, instead of vibrational or structural os-
cillation of molecular groups as in the infrared re-
gion. Electronic transitions in the UV-VIS region
depend upon the energy involved. For any molecu-
lar bound (sharing a pair of electrons), orbitals are
a mixture of two contributing orbitals σ and π, with
corresponding anti-bounding orbitals σ
∗
and π
∗
, re-
spectively (Bruice, 2006). Many organic molecules
present conjugated unsaturatedand carbonylsbounds,
such as aminoacids, phospholipids, free fatty acids,
phenols and flavonoids, peroxides, peptides and pro-
teins, sugars and their polymers absorbance in these
bands. Furthermore, many biological molecules
present chromophore groups, which increase the ab-
sorption in the UV-VIS region, such as: nitro, ni-
troso, azo, azo-amino, azoxy, carbonyl and thiocar-
bonyl, which can be used to characterize the different
metabolomic stages that occur on a bioprocess. Fur-
thermore, UV-VIS-SWNIR registers many features
such as fluorescence and vibrational resonances due
to energy decay of exited electrons, which may pro-
vide highly accurate fingerprinting of metabolites and
metabolic state of the fermentation.
In this research we explore the use of fiber op-
tics sensors for monitoring the yeast metabolic ac-
tivity during beer fermentation. Beer its the final re-
sult of wort fermentation with selected yeast. As the
metabolism is dependent on several internal and ex-
ternal variables the main objectives of this work were:
i) implement a fibber optics sensor capable of re-
producibility and immunity to processing conditions;
ii) determine the capacities of UV-VIS-SWNIR spec-
troscopy in capturing detailed metabolic features dur-
ing fermentation; and iii) determine the capacity for
detecting key metabolites by partial least squares re-
gression of the signal (biomass, extract, pH and sug-
ars).
2 MATERIALS AND METHODS
2.1 Fermentation
Two complete fermentations were monitored in this
exploratory study. The conical cylinder fermentor,
with maximum volume 1.9 L and a work volume of
1.4 L in Figure 1 (a) was used. To maintain a con-
stant temperature of 18
o
C a refrigeration serpentine
involves the cylindrical part of the bioreactor, being
possible to achieve a complete fermentation in 5 days.
The fermentation starts by adding the inoculum to the
malt wort (13.4
o
Brix) previously put inside the biore-
actor. The inoculum is composed of Saccharomyces
pastorianus (carsbergensis) (brewing yeast) with a
concentration of 12 to 16x10
6
cells / ml of wort. An
inoculum stock was stored in a freezer at −80
o
C in
order to obtain reproducibility between batches. In-
oculum was prepared in the following fashion: the
microorganisms were incubated in wort (previously
aired) under anaerobic conditions on a rotary shaker
(120 rpm) at 27
o
C for 72h. After this period of time
the yeast were collected from the wort by centrifu-
gation (5000 rpm for 10 minutes) in Sigma 4K15
(Sigma, 2008), and then diluted in NaCl (0,9% v/v)
in the reason of 4 ml/g of yeast, to form a paste.
Once obtained this paste it’s necessary determine, by
direct counting in the microscope of the yeast, the
correct volume of inoculum, to ensure the necessary
initial concentration of yeast on the fermentor (12 to
16x10
6
cells / ml). After this, the required volume
of inoculum is calculated and added to the wort to
begin fermentation. Monitorization was performed
at-line by removing 15 ml samples with a frequency
of three samples per day during the consecutive five
days of fermentation. These samples were thereafter
subjected to both spectroscopy and physical-chemical
measurements.
2.2 Spectroscopy
UV-VIS-SWNIR spectroscopy measurements
were performed using the fiber optic spec-
trometer AvaSpec-2048-4-DT (2048 pixel, 200-
1100nm)(Avantes, 2005). Standard Transmition
BIOSIGNALS 2009 - International Conference on Bio-inspired Systems and Signal Processing
328