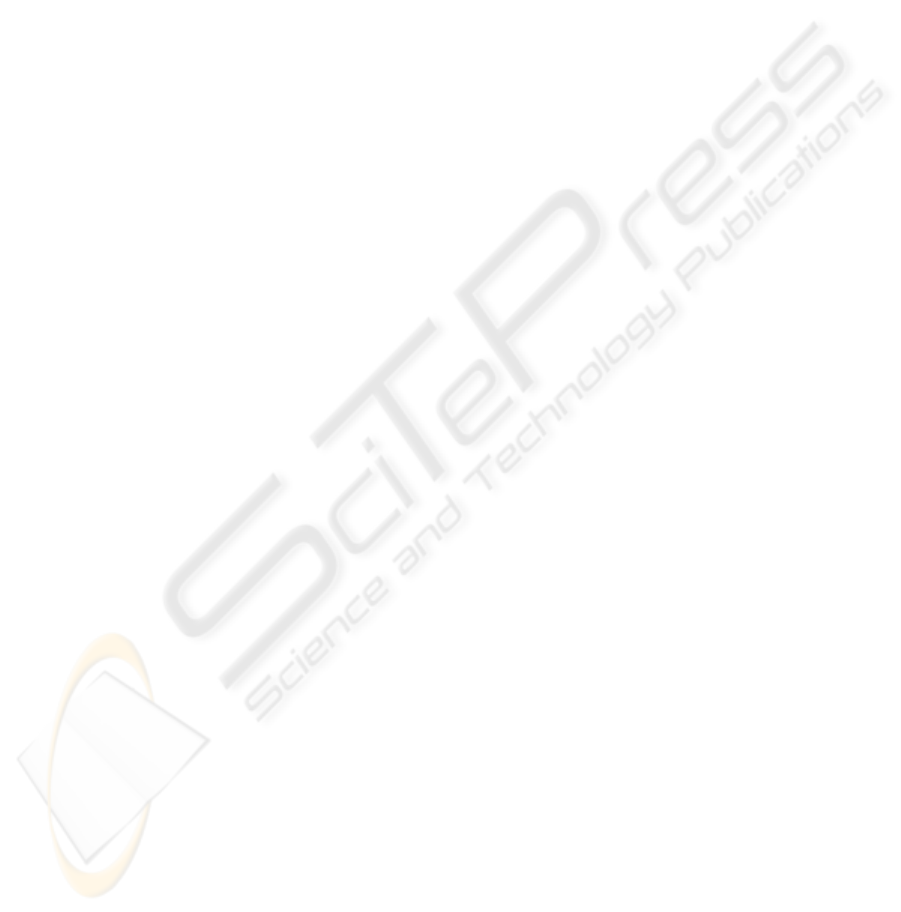
yeast spectra has completely different features when
responding to UV excitation, especially in the region
of 350-450 nm. Such high resolution and the dif-
ferences between the two light sources spectra, al-
lows us to conclude that UV-VIS-SWNIR is capa-
ble of high performance discrimination of the yeast
metabolic states.
However, as spectroscopy is a non-target ap-
proach, it is not possible identify directly the chem-
ical compounds and the transcripted genes that dif-
ferentiate the colonies. In order to understand deeper
the potential of UV-VIS-SWINR spectroscopy, it is
necessary to correlate spectroscopy data against high-
precision analytical techniques, such as mass spec-
troscopy (e.g. LC-MS/MS, GC-MS or Maldi-TOF),
NMR and transcriptomics (e.g. DNA/RNA Microar-
rays).
4 CONCLUSIONS
This work has shown that after appropriate prepro-
cessing and signal classification, UV-VIS-SWNIR
spectroscopy is a high resolution technique capable
of attaining interesting possibilities in non-destructive
metabolomics in the near future. Further insights will
be gained when spectral information is deeper under-
stood, not only by correlating with other high resolu-
tion analytical chemistry and molecular biology tech-
niques, but also in understanding of the spectra shape
of the differents microorganisms.
ACKNOWLEDGEMENTS
Part of this work was supported by the project Open-
MicroBio (PTDC/BIO/69310/2006) - ’A Framework
for Computational Simulation of Cellular Commu-
nities during BioProcess Engineering’; and partially
supported by CBMA, IBB/CEB and ISR/IST pluri-
anual funds through the POS-Conhecimento Program
that includes FEDER funds.
REFERENCES
Avantes, I. (2007). Users manual.
Baig, S. and Rehman, F. (2006). Signal modeling using sin-
gular value decomposition. In Advances in Computer,
Information, and Systems Sciences, and Engineering.
Springer Netherlands.
Bhatta, H., Goldys, E., and Learmonth, R. (2005). Rapid
identification of microorganisms by intrinsicfluores-
cence. In Imaging, Manipulation, and Analysis of
Biomolecules and Cells: Fundamentals and Applica-
tions III,. SPIE.
Burns, D. and Ciurczak, E. (2001). Handbook of near in-
frared analysisI. Marcel Dekker, Inc, New York, 2nd
edition edition.
Choi, S., Lee, C., Lee, J., Park, J., and Lee, I. (2005).
Fault detection and identification of non-linear pro-
cesses based on kernel pca. Chemometrics and intel-
ligent laboratory systems, 75:55–67.
Conlin, A., Martin, E., and Morris, A. (2000). Confidence
limits for contribution plots. Journal of Chemomet-
rics, 14:725–736.
Coyle, J. (1989). Introduction to Organic PhotoChemistry.
John Wiley & Sons, London.
Denney, R. and Sinclair, R. (1987). Visible and ultraviolet
spectroscopy. John Wiley & Sons, London.
Devices, A. S. (2005). Near-ir absorption bands.
Dickinson, J. (1994). Irreversible formation of pseudohy-
phae by haploid Saccharomyces cerevisiae. FEMS Mi-
crobiol. Lett., 119:99–104.
Dickinson, J. (2008). Filament formation in Saccharomyces
cerevisiae - a review. Folia Microbiol., 53(1):3–14.
Doytchinova, F. and Flower, D. (2006). Modeling the
peptide t-cell receptor interaction by the compara-
tive molecular similarity indices analysis-soft inde-
pendent modeling of class analogy technique. Journal
of Medicinal Chemistry, 49(7):2193–2199.
Dunia, R., Qin, S., Edgar, T., and T.J., M. (1996). denti-
fication of faulty sensors using principal component
analysis. American Institute of Chemical Engineers,
42:2797–2812.
Dziuba, B., Babuchowski, A., Naleczb, D., and Niklewicz,
M. (2007). Identification of lactic acid bacteria using
ftir spectroscopy and cluster analysis. In International
Dairy Journal 17: 183189. Elsevier.
Gallager, N. B., Blake, T., and Gassman, P. (2005). Ap-
plication of extended inverse scattering correction to
mid-infrared reflectance of soil. Journal of Chemo-
metrics, 19:271–281.
Gerard, J., Berdell, R., and Christine, L. (2006). Microbi-
ology: An Introduction. Benjamin Cummingsc, New
York, 2th edition edition.
Gimeno, C. J., Ljungdahl, P., Styles, C., and Fink, G.
(1992). Unipolar cell divisions in yeast Saccha-
romyces cerevisiae lead to filamentous growth: reg-
ulation by starvation and ras. Cell, 68:1077–1090.
Jackson, J. and Mudholkar, G. (1979). Control proce-
dures for residuals associated with principal compo-
nent analysis. Technometrics, 21:341–349.
Jolliffe, I. (1986). Principal Component Analysis. Springer,
New York, USA.
Klessinger, M. and Michl, J. (1995). Exited states and pho-
tochemistry of organic molecules. VCH Publishers,
New York.
Kron, S. and Gow, N. (1995). Budding yeast mor-
phogenesis: gignaling, cytoskeleton and cell cycle.
Curr.Opin.Cell Biol., 7:845–855.
YEAST METABOLIC STATE IDENTIFICATION BY FIBER OPTICS SPECTROSCOPY
177