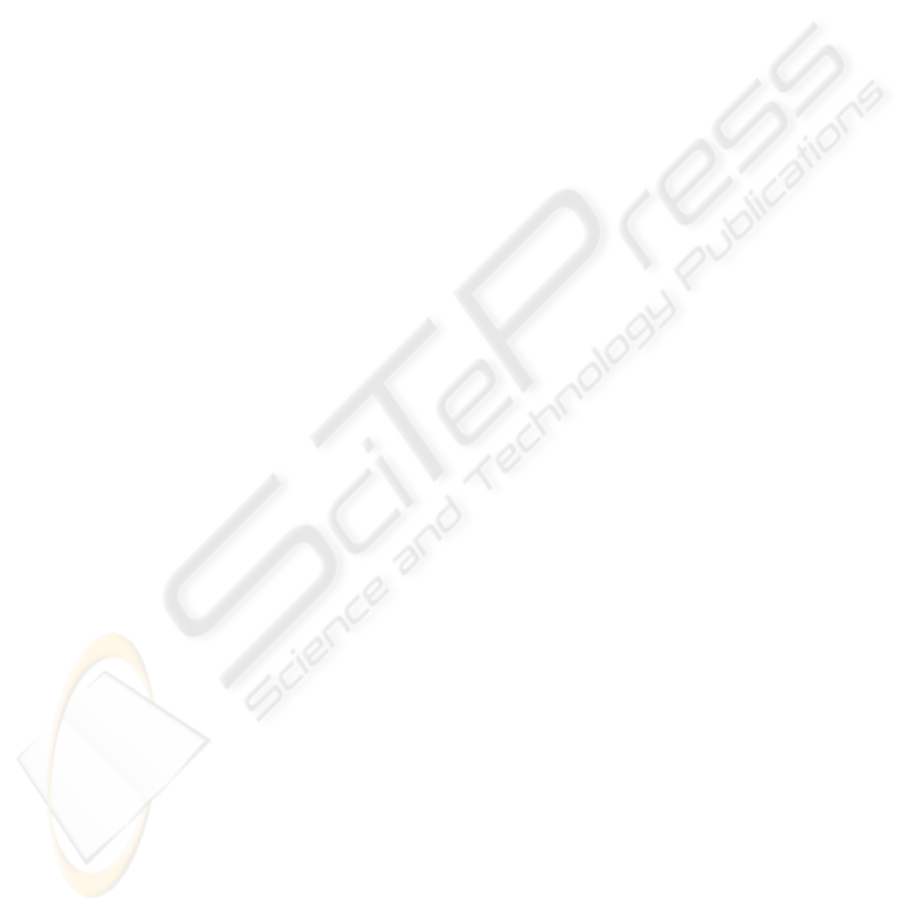
nology (CONACYT) and the Argentinian Ministry of
Science and Technology (SECYT) for their support to
the project ME/PA03-EXI/031.
REFERENCES
Charbuillet, C., Gas, B., Chetouani, M., and Zarader, J.
(2007a). Complementary features for speaker veri-
fication based on genetic algorithms. In Acoustics,
Speech and Signal Processing, 2007. ICASSP 2007.
IEEE International Conference on, volume 4, pages
IV–285–IV–288.
Charbuillet, C., Gas, B., Chetouani, M., and Zarader, J.
(2007b). Multi Filter Bank Approach for Speaker Ver-
ification Based on Genetic Algorithm, pages 105–113.
Davis, S. V. and Mermelstein, P. (1980). Comparison
of parametric representations for monosyllabic word
recognition in continuously spoken sentences. IEEE
Transactions on Acoustics, Speech and Signal Pro-
cessing, 28:57–366.
Deller, J. R., Proakis, J. G., and Hansen, J. H. (1993).
Discrete-Time Processing of Speech Signals. Macmil-
lan Publishing, NewYork.
Garofalo, J. S., Lamel, L. F., Fisher, W. M., Fiscus, J. G.,
Pallett, D. S., and Dahlgren, N. L. (1993). Darpa
timit acousticphonetic continuous speech corpus cd-
rom. Technical report, U.S. Dept. of Commerce,
NIST, Gaithersburg, MD.
Goldberg, D. E. (1989). Genetic Algorithms in Search, Op-
timization, and Machine Learning. Addison-Wesley
Professional.
Holland, J. H. (1975). Adaptation in natural and artificial
systems: An introductory analysis with applications to
biology, control, and artificial intelligence. University
of Michigan Press.
Huang, X. D., Ariki, Y., and Jack, M. A. (1990). Hidden
Markov Models for Speech Recognition. Edinburgh
University Press.
Jankowski, C. R., Vo, H. D. H., and Lippmann, R. P. (1995).
A comparison of signal processing front ends for auto-
matic word recognition. IEEE Transactions on Speech
and Audio Processing, 4(3):251–266.
Jelinek, F. (1999). Statistical Methods for Speech Recogni-
tion. MIT Press, Cambrige, Masachussets.
Lai, Y.-P., Siu, M., and B., M. (2006). Joint optimiza-
tion of the frequency-domain and time-domain trans-
formations in deriving generalized static and dynamic
mfccs. Signal Processing Letters, IEEE, 13:707–710.
Rabiner, L. and Juang, B.-H. (1993). Fundamentals of
Speech Recognition. Prentice Hall PTR.
Skowronski, M. and Harris, J. (2002). Increased mfcc fil-
ter bandwidth for noise-robust phoneme recognition.
Proceedings of the IEEE International Conference on
Acoustics, Speech, and Signal Processing (ICASSP),
1:I–801–I–804.
Skowronski, M. and Harris, J. (2003). Improving the filter
bank of a classic speech feature extraction algorithm.
In Proceedings of the 2003 International Symposium
on Circuits and Systems (ISCAS), volume 4, pages IV–
281–IV–284.
Skowronski, M. and Harris, J. (2004). Exploiting indepen-
dent filter bandwidth of human factor cepstral coeffi-
cients in automatic speech recognition. The Journal of
the Acoustical Society of America, 116(3):1774–1780.
Stevens, K. N. (2000). Acoustic Phonetics. Mit Press.
Tang, K., Man, K. F., Kwong, S., and He, Q. (1996). Ge-
netic algorithms and their applications. IEEE Signal
Processing, 13(6):22–29.
Vignolo, L., Milone, D., Rufiner, H., and Albornoz, E.
(2006). Parallel implementation for wavelet dictio-
nary optimization applied to pattern recognition. In
Proceedings of the 7th Argentine Symposium on Com-
puting Technology, Mendoza, Argentina.
Young, S., Kershaw, D., Odell, J., Ollason, D., Valtchev, V.,
and Woodland, P. (2000). HMM Toolkit. Cambridge
University.
GENETIC OPTIMIZATION OF CEPSTRUM FILTERBANK FOR PHONEME CLASSIFICATION
185