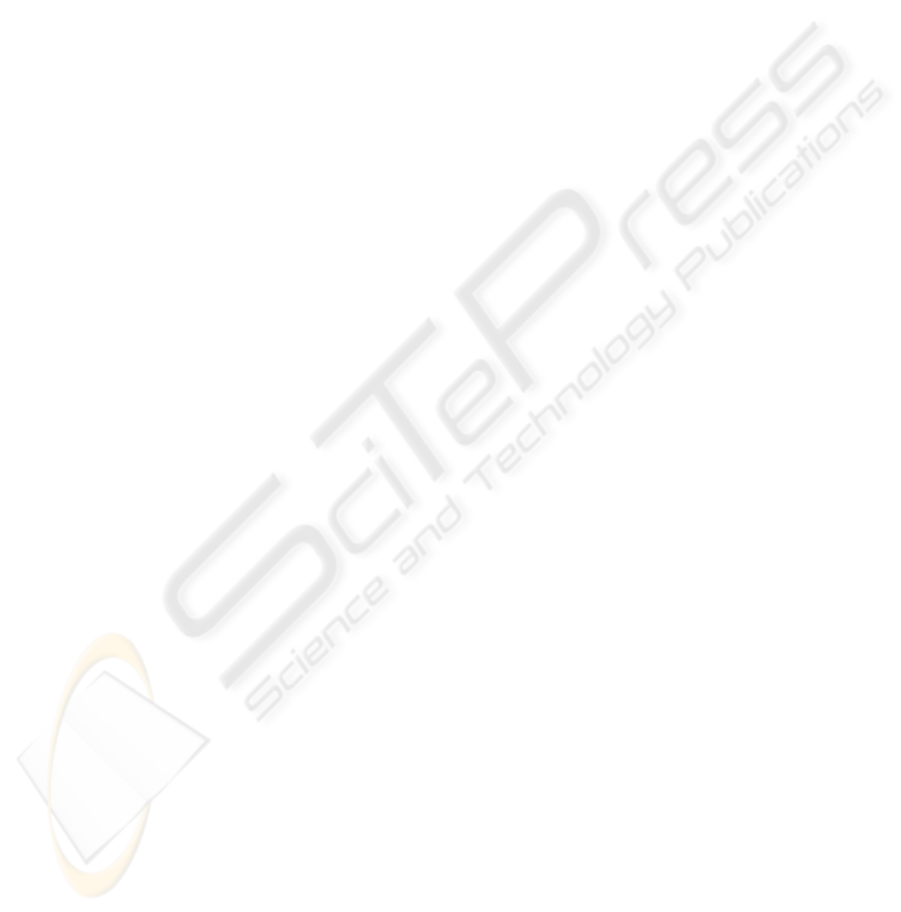
than half of the total APCs, which means that all
the anomalies was detected and, in some cases, both
APCs of an anomalies raised an alert. We was also
succeeded in the last 5 experiments, whose goal was
to evaluate the use of TAT for classification. In the
testing phase we have a high rate of true positive and
a relatively low rate of false alarms. The higher level
of false alarms was 7.5% in the third experiment.
5 DISCUSSION
We have described an algorithm for anomaly detec-
tion based on the TAT theoretical immunological hy-
pothesis. Our main goal was to present a general
architecture of a TAT based AIS and an immune-
inspired algorithm for anomaly detection that could
deal with temporal events. We presented some pre-
liminary results obtained with artificially generated
data-sets that meet some of the characteristics ob-
served on real-world contextual data-sets. We have
also started to analyse the appropriateness of using
TAT in both a detection and classification context.
Despite the limited diversity of the data-sets used,
we believe that the algorithm proposed show that TAT
possesses a handful of promising properties when ap-
plied to temporal anomaly detection. Firstly, each
environment has its own characteristics and therefore
the detection system should reflect this individuality,
through the automatic adjustment of each cell acti-
vation threshold. Secondly, TCELL activation is an
automatic process based on changes in signal inten-
sity and the current values for the K and P. Each
TCELL has its sensitivity adjusted to a baseline that
is characteristic of the past and current activity. Fi-
nally, in TAT, normal activity is manifested by the
presence of recurrent signals and abnormal activities
correspond to exceptional signals for which the reper-
toire of TCELLs should not be adjusted. This is pre-
cisely what is supposed to happen in the detection of
anomalies in real-world applications.
In this phase we neglected some essential prop-
erties of the natural IS that can make adaptation to
evolving normality and anomaly detection more ro-
bust and reliable: immune memory and clonal dy-
namics. Future developments of the TAT-based de-
tector should aim at incorporating these properties.
Less because this would make the AIS in line with
the natural counterpart, but because variation in clonal
size can be a way of adjusting the weight of each
TCELL specificity in the ”committee”, reflecting not
only the history of the signals but also the history of
co-occurrences of those signals.
The preliminary results obtained are in line with
those described by the authors in (Antunes and Cor-
reia, 2008). The results were also promising and the
ongoing research give us confidence to deploy a TAT-
based algorithm for anomaly detection.
ACKNOWLEDGEMENTS
The authors acknowledge the facilities and research
environment gracefully provided by the CRACS
(Center for Research in Advanced Computing Sys-
tems) research unit, an INESC associate of the Fac-
ulty of Science, University of Porto.
REFERENCES
Antunes, M. and Correia, M. (2008). TAT-NIDS: an
immune-based anomaly detection architecture for net-
work intrusion detection. IWPACBB08 - Advances in
Soft Computing (Springer), pages 6067.
Carneiro, J., Paixo, T., Milutinovic, D., Sousa, J., Leon,
K., Gardner, R., and Faro, J. (2005). Immunological
self-tolerance: Lessons from mathematical modeling.
Journal of Computational and Applied Mathematics,
184(1):77100.
de Castro, L. and Timmis, J. (2002). Artificial Immune Sys-
tems: A New Computational Intelligence Approach.
Springer.
Forrest, S., Perelson, A., Allen, L., and Cherukuri, R.
(1994). Self-nonself discrimination in a computer.
Proceedings of the 1994 IEEE Symposium on Re-
search in Security and Privacy, pages 201212.
Greensmith, J., Twycross, J., and Aickelin, U. (2006). Den-
dritic cells for anomaly detection. In Proc. of the IEEE
World Congress on Computational Intelligence, pages
664671.
Grossman, Z. and Paul, W. (1992). Adaptive cellular in-
teractions in the immune system: The tunable acti-
vation threshold and the significance of subthreshold
responses. Proceedings of the National Academy of
Sciences, 89(21):1036510369.
Kim, J., Bentley, P., Aickelin, U., Greensmith, J., Tedesco,
G., and Twycross, J. (2007). Immune system ap-
proaches to intrusion detection - a review. Natural
Computing, 6(4):413466.
Pedroso, J. (2007). Simple Metaheuristics Using the Sim-
plex Algorithm for Non-linear Programming. LNCS,
4638:217221.
Sompayrac, L. (2008). How the Immune System Works.
Blackwell Publishing.
BIOSIGNALS 2009 - International Conference on Bio-inspired Systems and Signal Processing
362