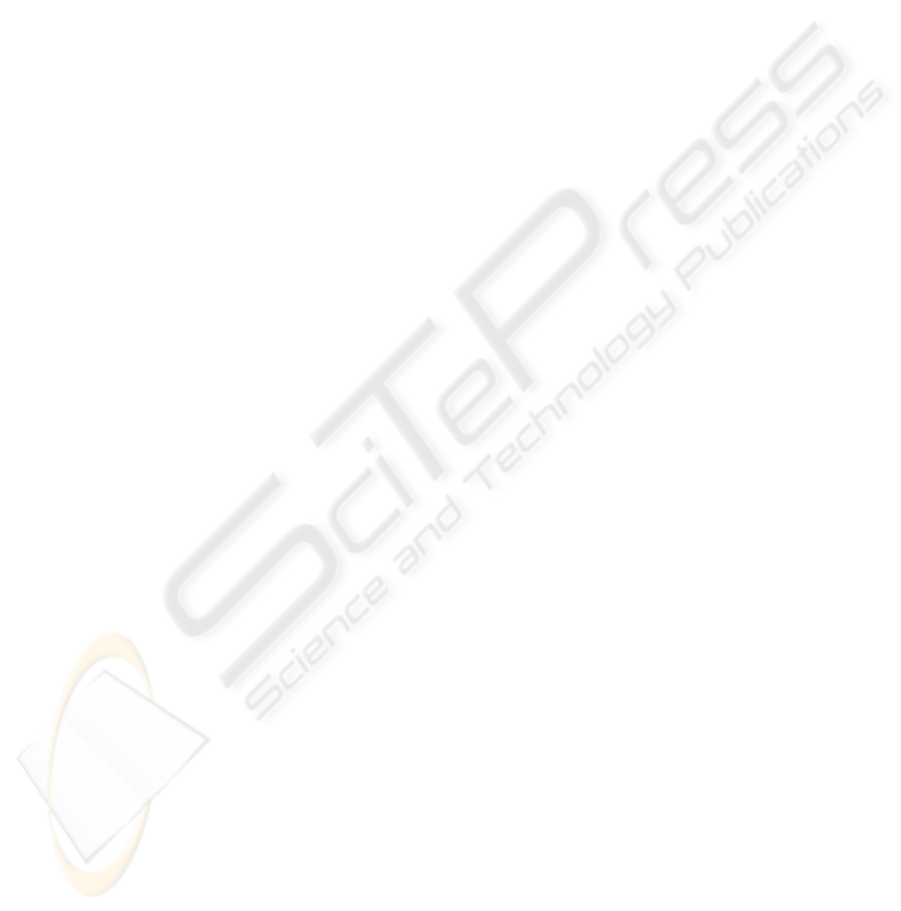
2006) seem most likely to have been caused by an ar-
tifact in the experimental design, that is temporal cor-
related patterns were recognized rather than words.
Thus, we conclude that hypothesis B is probably cor-
rect. Furthermore, our experiments showed that cross
session training (within subjects) only yields recogni-
tion rates at chance level, even if the same word order
was used for the recordings.
Of course, our analysis does not imply that it is
impossible in general to correctly extract (and clas-
sify) unspoken speech from EEG data. It has to be
pointed out that we do not address the general ques-
tion of whether this is feasible. Instead, we focus
on the method proposed in (Wester, 2006) and show
that it is not well suited for the task. Furthermore, it
should be taken into account that some assumptions
are proposed here which we cannot prove so far.
However, the approach taken here could be
changed and improved in several respects. First, using
a vocabulary of words with semantic meaning might
lead to improvements. Apart from this, it would prove
useful to provide JRTk with more training data by
recording a higher number of repetitions per word.
Second, the recognizing system itself needs to be
changed. Due to the high variation of the length of the
utterances, normalization would most probably im-
prove the performance of the system. Furthermore,
a different word model might be more suitable than
HMMs since it turned out that HMMs with just one
state yield fairly good results. A one state HMM how-
ever does not model temporal data anymore.
Third, the subject could be provided with feed-
back on whether a given word was recognized cor-
rectly. It has been shown in (Birbaumer, 2000) that
subjects can indeed be trained to modify their brain
waves for using an EEG-based BCI. Thus, we would
expect that the subject could adapt his/her brain waves
such that they are recognized more easily .
REFERENCES
Becker, K. (2004). Gebrauchsanweisung f
¨
ur VarioPort
TM
.
Birbaumer, N. (2000). The thought translation device (ttd)
for completely paralyzed patients. IEEE Trans Reha-
bil Eng., 8(2):190–3.
Birbaumer, N., Ghanayim, N., Hinterberger, T., Iversen, I.,
Kotchoubey, B., K
¨
ubler, A., Perelmouter, J., Taub, E.,
and Flor, H. (1999). A spelling device for the paral-
ysed. Nature, 398:2978.
Blankertz, B., Dornhege, G., Krauledat, M., M
¨
uller, K.-R.,
Kunzmann, V., Losch, F., and Curio, G. (2006). The
berlin brain-computer interface: Eeg-based communi-
cation without subject training. IEEE Trans. Neural
Sys. Rehab. Eng., 14(2):147–152.
Calliess, J.-P. (2006). Further investigations on unspoken
speech. Institut f
¨
ur Theoretische Informatik Univer-
sit
¨
at Karlsruhe (TH), Karlsruhe, Germany.
Dornhege, G., del R. Millan, J., Hinterberger, T., McFar-
land, D., and M
¨
uller, K.-R., editors (2007). Towards
Brain-Computer Interfacing. MIT Press.
Lotte, F., Congedo, M., Lecuyer, A., Lamarche, F., and
Arnaldi, B. (2007). A review of classification algo-
rithms for eeg-based braincomputer interfaces. J. Neu-
ral Eng., 4:R1–R13.
Maier-Hein, L. (2005). Speech recognition using surface
electromyography. Master’s thesis, Institut f
¨
ur Theo-
retische Informatik Universit
¨
at Karlsruhe (TH), Karl-
sruhe, Germany.
Neuper, C., M
¨
uller, G. R., K
¨
ubler, A., Birbaumer, N., and
Pfurtscheller, G. (2003). Clinical application of an
eeg-based braincomputer interface: a case study in a
patient with severe motor impairment. Clin. Neuro-
physiol., 114:399–409.
Nijholt, A., Tan, D., Pfurtscheller, G., Brunner, C., Millan,
J., Allison, B., Graimann, B., Popescu, F., Blankertz,
B., and M
¨
uller, K.-R. (2008). Brain-computer in-
terfacing for intelligent systems. IEEE Intell. Syst.,
23:7279.
Porbadnigk, A. (2008). Eeg-based speech recognition: Im-
pact of experimental design on performance. Institut
f
¨
ur Algorithmen und Kognitive Systeme, Universit
¨
at
Karlsruhe (TH), Karlsruhe, Germany.
Scherer, R., M
¨
uller, G., Neuper, C., Graiman, B., and
Pfurtscheller, G. (2004). An synchronously con-
trolled eeg-based virtual keyboard: Improvement of
the spelling rate. IEEE Trans. Neural Syst. Rehabil.
Eng., 51(6):979984.
Suppes, P., Lu, Z.-L., and Han, B. (1997). Brain wave
recognition of words. Proc. Natl. Acad. Sci. USA,
94:14965–14969.
Waibel, A., Bett, M., Metze, F., Ries, K., Schaaf, T.,
Schultz, T., Soltau, H., Yu, H., and Zechner, K.
(2001). Advances in automatic meeting record cre-
ation and access. In Proc. ICASSP ’01, volume 1,
pages 597–600.
Wand, M. (2007). Wavelet-based preprocessing of eeg and
emg signals for speech recognition. Institut f
¨
ur Theo-
retische Informatik Universit
¨
at Karlsruhe (TH), Karl-
sruhe, Germany.
Wester, M. (2006). Unspoken speech - speech recogni-
tion based on electroencephalography. Master’s the-
sis, Institut f
¨
ur Theoretische Informatik Universit
¨
at
Karlsruhe (TH), Karlsruhe, Germany.
Wolpaw, J. R., Birbaumer, N., McFarland, D., Pfurtscheller,
G., and Vaughan, T. (2002). Brain-computer inter-
faces for communication and control. Clin. Neuro-
physiol., 113(6):767791.
Wolpaw, J. R., McFarland, D. J., Vaughan, T. M., and
Schalk, G. (2003). The wadsworth center brain-
computer interface (bci) research and development
program. IEEE Trans. Neural Syst. Rehabil. Eng.,
11(2):207–207.
EEG-BASED SPEECH RECOGNITION - Impact of Temporal Effects
381