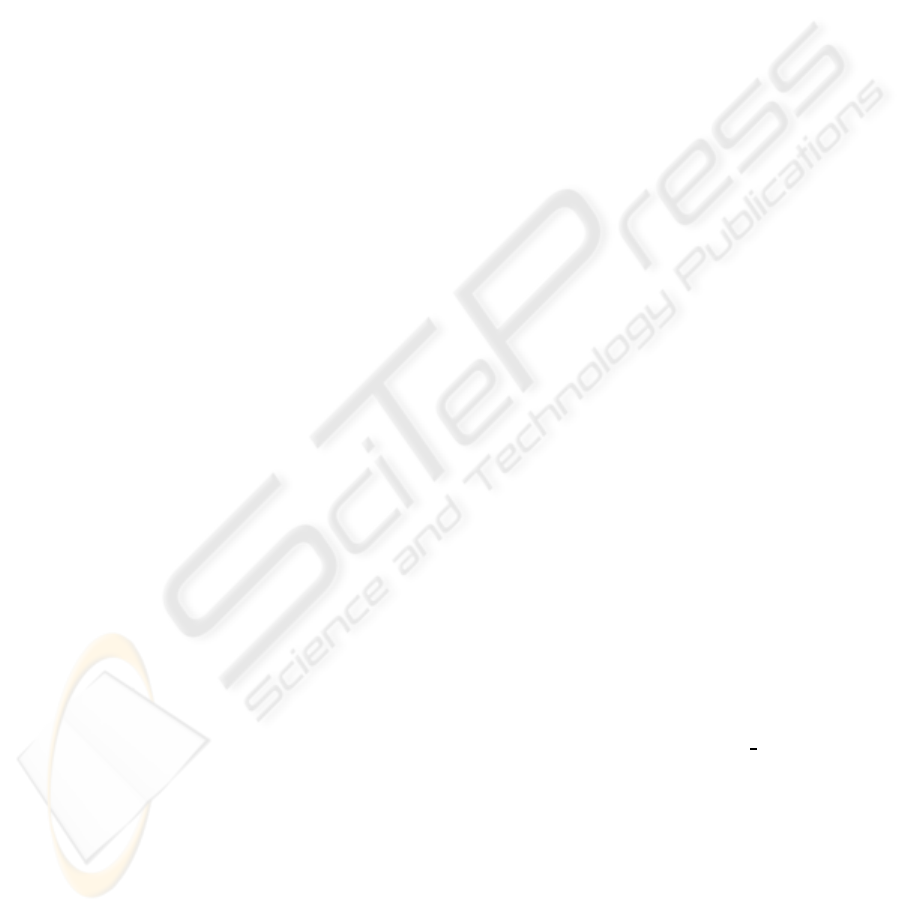
shows that the “education level” has a high impact
on the number of errors for the scenarios Curr.Scenar
and RNA Auto.. RFID technologies removemany crit-
ical processes like handwriting and manual computer-
izations, so the “education level” has less impact for
the two scenarios including auto-ID.
Table 2 shows that the proportion of used re-
sources is reduced by 10.56% when adding the RNA
automation within the biobank, because the RNA ex-
traction needs less human intervention and the time
needed to transport the samples to the place where
this transformation takes place is highly reduced as
soon as the automation is in the same clean area. The
gains related to RFID technologies are very large. The
saved time is mainly composed of computerization,
handwriting and bringing information leafs. The job
of the technicians would consist of tasks that are more
specific to their core competences. As mentioned in
Section 3.1, we believe this is an indicator of the em-
ployee welfare. The difference between the use of a
pen reader or a matrix antenna is small. Thus, using
the matrix antenna would at first require to compare
the earnings with the cost of such a device. On the
other hand, the use of a 2D position reading antenna
could help to reorganize boxes when performing in-
ventories, thus leading to potential large savings.
Our results do not include a financial part yet for
two reasons. First, the costs of emerging technolo-
gies decrease quickly with the increase of their use.
Second, the market for tagged cryotubes and matrix
readers is not very large, so the prices are not stabi-
lized yet.
4 CONCLUSIONS
In this paper, we address the impact of RFID tech-
nologies in a specific healthcare application using
Discrete Event Dynamic Systems (DEDS) simulation
used as a decision support system. Our study helps to
quantify the benefits of integrating new auto-ID tech-
nologies and devices at different levels of a biobank.
The results show that auto-ID technologies may con-
siderably improve the performances of the biobank
when taking into account the percentages of errors as
well as the reduction of resource uses and processing
times as performance indicators. We still have data to
analyze in order to configure the simulation and vali-
date the relevance of some of our assumptions. This
part of our work should be possible only after the pi-
lot results have been observed for a long enough time
period so they are stabilized.
The proposed model should allow us to model
other biobanks and/or material configurations quite
quickly, including organ sample arrivals. This can
be made using the same basic model file and only
modifying the specific configuration file. A graphi-
cal user interface could allow biobanks managers to
model themselves their biobank. These types of tools
could be very helpful when auditing and expertizing
structures that must adopt new technologies because
of new legislations.
REFERENCES
Boginski, V., Mun, I., Y. Wu, K. M., and Zhang, C. (2007).
Simulation and analysis of hospital operations and
ressource utilization using RFID data. In IEEE In-
ternational Conference on RFID, Grapevine, Texas,
USA, pages 199–204.
Bonnabry, P. (2007). How can the labelling and the packag-
ing of drugs impact on the drug safety? In Swissmedic,
Bern, Suisse.
Dalton, G. D., Samaropoulos, X. F., and Dalton, A. C.
(2008). Improvements in the safety of patient care can
help end the medical malpractice crisis in the united
states. In Health Policy, volume 86, pages 153–162.
Garnerin, P., Pellet-Meier, B., Chopard, P., Pergener, T.,
and Bonnabry, P. (2007). Measuring human-error
probabilities in drug preparation : a pilot study. In
European Journal of Clinical Pharmacology, volume
63(8), pages 769–776.
Hedgepeth, W. O. (2007). RFID Metrics. CRC Press.
Housseman, S., Absi, N., and Dauz`ere-P´er`es, S. (2008a).
Simulating RFID applications in healthcare. Techni-
cal report, EMSE-CMP.
Housseman, S., Absi, N., Dauz`ere-P´er`es, S., Daufresne, L.-
M., and Hofman, P. (2008b). Utilisation des NTIC
dans la gestion d’un stock d’´echantillons biologiques:
la simulation pour aider `a la d´ecision. In GISEH’08:
Gestion et Ing´enierie des Syst`emEs Hospitaliers, Lau-
sanne, Switzerland, pages 167–174.
Howard, D. H., Meltzer, D., Kollman, C., Maiers,
M., Logan, B., Gragert, L., Setterholm, M.,
and Horowitz, M. M. (2005). Optimal Size
of a National Cord Blood Bank. http://www.
sph.emory.edu/ dhhowar/cordblood 05162005.pdf.
Kleijnen, J. (2005). Supply chain simulation tools and tech-
niques: a survey. In International Journal of Simula-
tion and Process Modeling, volume 1(1/2), pages 82–
89.
Kohn, L., Corrigen, J., and Donaldson, M. (2000). To err
is human. building a safer health system. In The Na-
tional Academies Press, Washington DC, USA.
Law, A. and Kelton, W. (2000). Simulation Modeling and
Analysis. Mc Graw Series in Industrial Engineering
and Management Science.
Marcellis-Warin, N. D. (2003). Les risques hospitaliers. In
La lettre des cyndiniques, number 40.
HEALTHINF 2009 - International Conference on Health Informatics
342