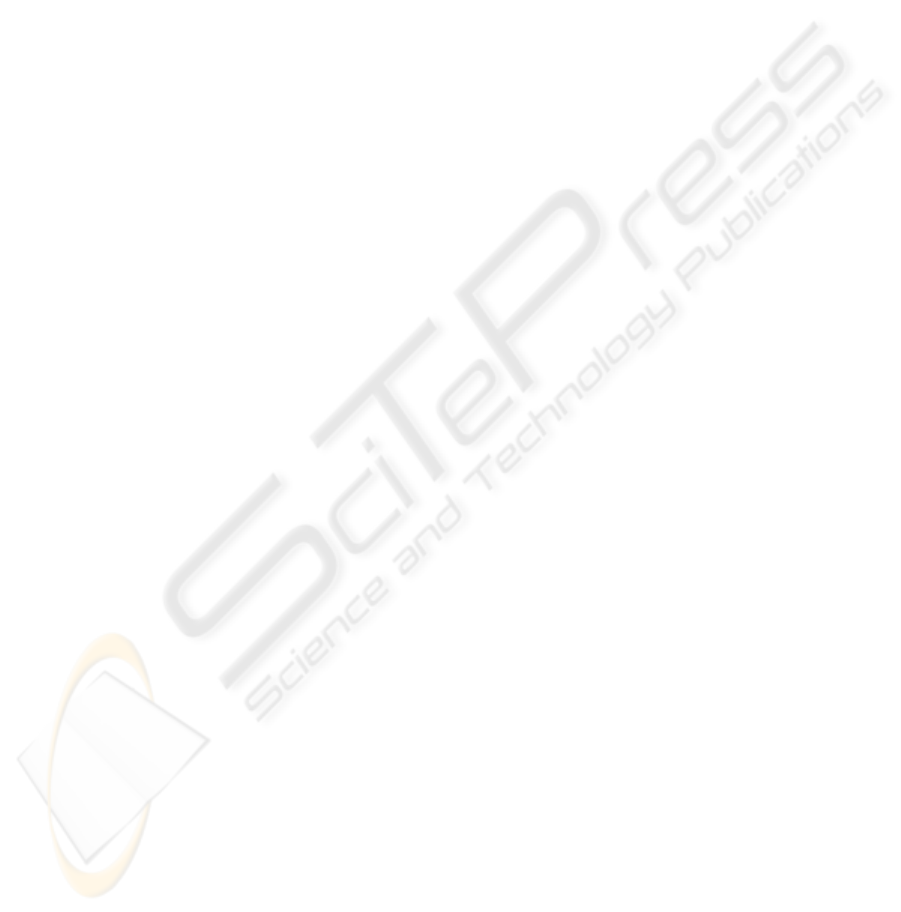
The BDP Agent will be implemented within the
MAS in the future.
8 CONCLUSIONS
This study has presented machine learning
techniques to follow and track the learner’s
brainwaves frequency bands amplitudes. It
completes many previous works that assess
emotional parameters from brainwaves by using an
EEG. This can be useful for some particular learners
as taciturn, impassive and disabled learners. We do
not consider the whole cases of disabled learners.
We will consider only disabled learner who cannot
express facial emotions or body gestures due to an
accident or a surgery and also those who lost their
voice or cannot talk. Here we are talking about
physical disability and not mental disability. This
procedure allowed us to record the brainwaves
amplitudes of the learners exposed to emotional
stimuli from the International Picture System. These
data were used to predict the future dominant
amplitude knowing the picture category and the
actual brainwaves frequency band amplitudes.
We acknowledge that the use of EEG has some
potential limitations. In fact, any movement can
cause noise that is detected by the electrodes and
interpreted as brain activity by Pendant EEG.
Nevertheless, we gave a very strict instructions to
our participants. They were asked to remain silent,
immobile and calm. We believe that the instructions
given to our participants, their number (17) and the
database size (33106 records) can considerably
reduce this eventual noise. Results are encouraging,
a potential significant impact of emotional stimuli
and the brainwave amplitudes. The decision tree
analyses resulted in accurate predictions 93.82% and
the Yuden’s J-Index is 73.22%. If the method
described above proves to be effective in tracking
the learner’s brainwaves amplitudes, we can direct
our focus to a second stage. An ITS would select an
adequate pedagogical strategy that adapt to certain
learner’s mental states correlated to the brainwaves
frequency bands in addition to cognitive and
emotional states. This adaptation would increase the
bandwidth of communication and allow an ITS to
respond at a better level. If this hypothesis holds in
future replication, then it would give indications on
how to help those learners to induce positive mental
states during learning.
ACKNOWLEDGEMENTS
We acknowledge the support of the FQRSC (Fonds
Québécois de la Recherche sur la Société et la
Culture) and NSERC (National Science and
Engineering Research Council) for this work.
REFERENCES
Bear, M.F., Connors, B.W., Paradiso, M. A, 2001.
Neuroscience: Exploring the Brain, second ed.
Lippincott Williams & Williams, Baltimore, MD.
Bellifemine, F., Poggi, A., Rimassa, G., 1999. JADE - A
FIPA-compliant Agent Framework, In PAAM'99,
London, UK.
Bradley, M.M., Codispoti, M., Cuthbert, B.N., Lang, P.J.,
2001. Emotion and motivation: Defensive and
appetitive reactions in picture processing. Emotion, 1.
Cantor, D.S., 1999. An overview of quantitative EEG and
its applications to neurofeedback. In Introduction to
Quantitative EEG and Neurofeedback. J. R. Evans and
A. Abarbanel, Eds. Academic Press.
Conati, C., 2002. Probabilistic assessment of user's
emotions in educational games. Journal of Applied
Artificial Intelligence.
Conati, C., 2004, How to evaluate models of user affect?
In Proceedings of ADS 04, Tutorial and Research
Workshop on Affective Dialogue Systems. Kloster
Irsee, Germany.
Conati, C., Mclaren H., 2005. Data-driven Refinement of a
Probabilistic Model of User Affect. In Proceedings of
UM2005 User Modeling: Proceedings of the Tenth
International Conference, Lecture Notes in Computer
Science, Springer Berlin / Heidelberg..
D'Mello, S.K., Craig, S.D., Gholson, B., Franklin, S.,
Picard, R.W., Graesser, A.C., 2005. Integrating Affect
Sensors in an Intelligent Tutoring System. In Affective
Interactions: The Computer in the Affective Loop
Workshop at 2005 International conference on
Intelligent User Interfaces. AMC Press, New York.
Ekman, P., 1992. Are there basic emotions? Psychological
Review.
Fan, C., Sarrafzadeh, A., Overmyer, S., Hosseini, H. G.,
Biglari-Abhari, M., Bigdeli, A., 2003. A fuzzy
approach to facial expression analysis in intelligent
tutoring systems. In Antonio Méndez-Vilas and
J.A.Mesa González Eds.
Heraz, A., Frasson, C., 2008. Predicting the three major
dimensions of the learner’s emotions from brainwaves.
International Journal of Computer Science.
Heraz, A., Razaki, R. Frasson, C., 2007. Using machine
learning to predict learner emotional state from
brainwaves. 7th IEEE conference on Advanced
Learning Technologies: ICALT2007, Niigata, Japan.
Kort, B., Reilly, R., Picard, R., 2001. An affective model
of interplay between emotions and learning:
Reengineering educational pedagogy—building a
learning companion. In T. Okamoto, R. Hartley,
HOW DO EMOTIONAL STIMULI INFLUENCE THE LEARNER’S BRAIN ACTIVITY? - Tracking the Brainwave
Frequency Bands Amplitudes
19