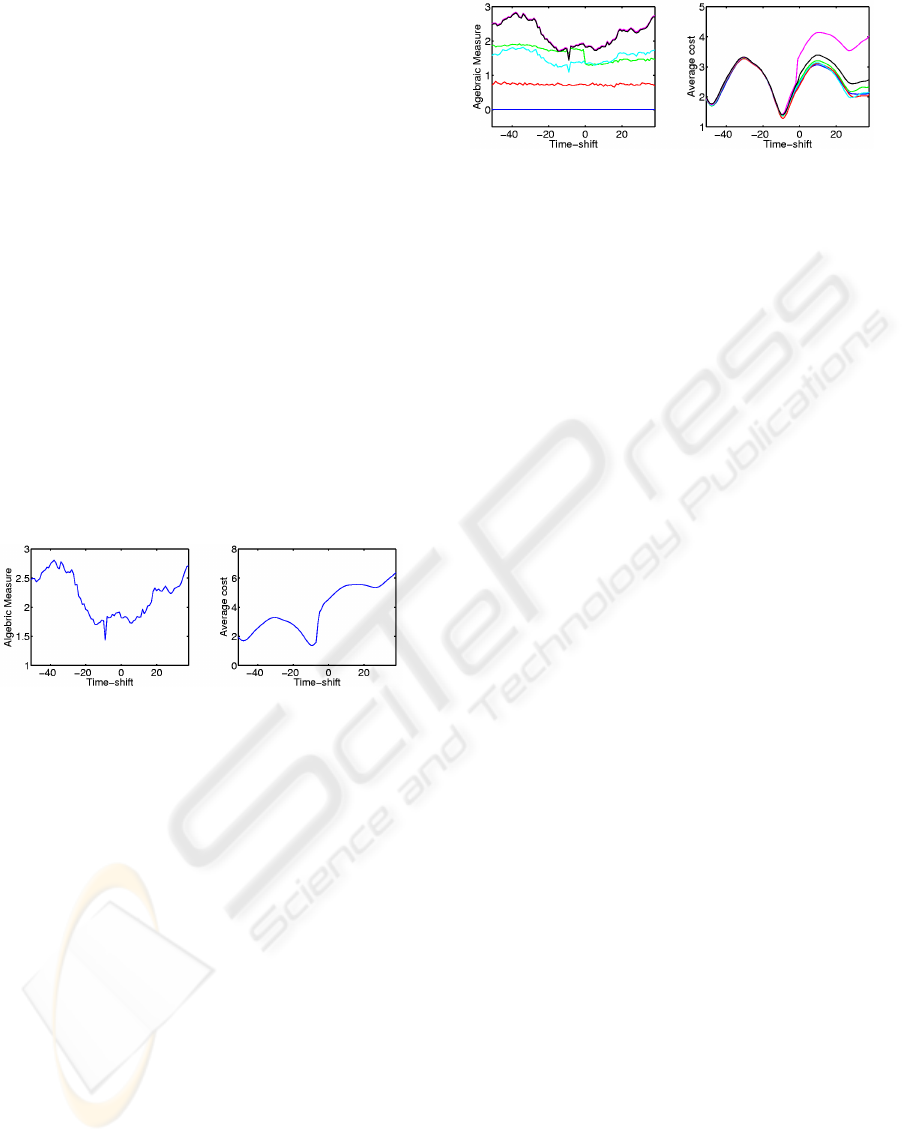
and Fig. 10(c) recover the ground truth transforma-
tions (blue curve).Thesynchronization of the first pair
of image sequences demonstrates that the method can
handle large time-shift, provided that motion in se-
quences is not periodic. In addition, we can observe
that appearances and disappearances of the players
in the second pair of videos do not disturb the time-
warping estimation.
4.4 Comparison
In this subsection, we compare our method with the
approach proposed by (Wolf and Zomet, 2006)(WZ).
Due to the lack of space, we do not describe this
method and invite the reader to refer to the paper for
details. Their approach can be used to align sequences
linked by time-shift transformation. For each possi-
ble time-shift value, they evaluate an algebraic mea-
sure based on rank constraints of trajectory-based ma-
trices. They retain the time-shift that minimizes this
measure. They propose to represent results by a graph
of the computed measure versus the time-shift as il-
lustrated in Fig. 11(a).
(a) (b)
Figure 11: Results on noise-free projected MoCAP point
trajectories (a) WZ result : the algebraic error versus time-
shift (b) Our result : the average cost versus time-shift.
In order to have similar result representation, we
compute the average cost value in the cost matrix on
a path for a given time-shift. We plot this average
value versus the time-shift as illustrated in Fig. 11(b).
Fig. 11 presents results for both methods on MoCAP
dataset for the same example as in Fig. 4(e-h) but
using 20 trajectories randomly chosen for each se-
quence. We re-compute SSMs for these trajectories.
In order to compare robustness of the two ap-
proaches, we apply noises with different variances.
We can observe on Fig. 12 that for low variance noise
(black, magenta and cyan curves) both methods re-
cover the time-shift. However for higher variances,
our method can recover the time-shift whereas their
approach has difficulties (green, red and blue curves).
(a) (b)
Figure 12: Results for noisy data (a) WZ result : the alge-
braic error versus time-shift (b) Our result : the average cost
versus time-shift.
5 CONCLUSIONS
We have presented a novel approach for video syn-
chronization based on temporal self-similarities of
videos. It is characterized by its simplicity and its
flexibility: we do not impose restrictive assumptions
as sufficient background information, or point cor-
respondences between views. In addition, temporal
self-similarities, which are not strictly view-invariant,
supply view-independentdescriptors for synchroniza-
tion. Although our method does not provide syn-
chronization with sub-frame accuracy, it can perform
video synchronization automatically without tempo-
ral misalignment modeling.
We have validated our framework on datasets with
controlled view settings and tested its performance
on challenging real videos. These videos were cap-
tured by static cameras but the method could be ap-
plied to moving cameras, which we will investigate in
future work. Furthermore, as the self-similarity ma-
trix structures are not only stable under view changes
but also specific to actions, the method could address
the problem of action synchronization, i.e. the tempo-
ral alignment of sequences featuring the same action
performed by different people under different view-
points.
REFERENCES
Benabdelkader, C., Cutler, R. G., and Davis, L. S.
(2004). Gait recognition using image self-similarity.
EURASIP J. Appl. Signal Process., 2004(1):572–585.
Carceroni, R., Padua, F., Santos, G., and Kutulakos, K.
(2004). Linear sequence-to-sequence alignment. In
Proc. Conf. Comp. Vision Pattern Rec., pages I: 746–
753.
Caspi, Y. and Irani, M. (2002). Spatio-temporal alignment
of sequences. IEEE Trans. on Pattern Anal. and Ma-
chine Intell., 24(11):1409–1424.
Cha, S. and Srihari, S. (2002). On measuring the dis-
VISAPP 2009 - International Conference on Computer Vision Theory and Applications
390