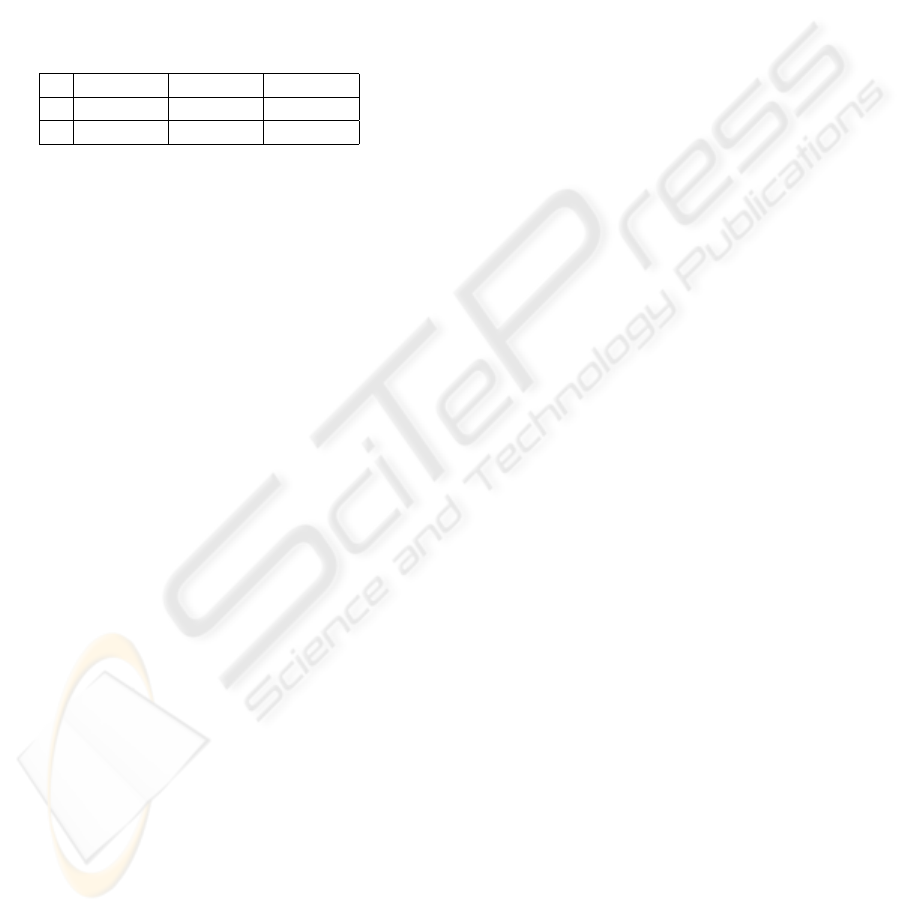
To verify our EM algorithm we have also used the
chosen parameters as initial values. The maxima of
the relative deviations between the chosen and ex-
tracted parameters of the twofold Gaussian mixture
models are given in Table 3. So, if the chosen pa-
rameters of the twofold Gaussian mixture models are
used as initial values, the EM algorithm result in very
small relative errors.
Table 3: The maxima of the relative deviations between the
chosen and extracted parameters of the twofold Gaussian
mixture models. Chosen parameters used as initial values.
k is the number of the Gaussian component.
k π µ σ
1 0.0015% 0.0000% 0.0000%
2 0.0020% 0.0031% 0.0249%
The number of iterations for the experiments with
the initial values derived from the histograms, varied
between 4 and 910 with a mean value of 77.3. As
can be expected, the number of iterations for the ex-
periments with the initial values equal to the chosen
parameters was much lower, namely always 2.
4.3 Discussion
As is clear from Fig. 5 upto and including Fig. 10,
the relative errors in the extracted parameters of the
twofold Gaussian mixture models are related to the
differences between the chosen mean coefficients µ
1
and µ
2
. So, as can be expected, the EM algorithm may
give better results if the mean coefficients µ
1
and µ
2
of the two Gaussian components differ more.
But for our gray value segmentation algorithms
the only important parameter is the segmentation
threshold (i.e. the intersection point of the two Gaus-
sian components). The extracted versus the ”chosen”
threshold is shown in Fig. 13. The maxima of the ab-
solute and relative deviations are 0.3922 (also a bin
number between 1 and 256) respectively 1.1111%.
So, the deviations in the threshold are also small.
To facilitate value judgment of the EM threshold
deviations, we extracted the Kittler threshold (Kit-
tler and Illingworth, 1986) from the generated his-
tograms. The Kittler versus the ”chosen” threshold
is shown in Fig. 16. The maxima of the absolute and
relative deviations are 2.7451 (also a bin number be-
tween 1 and 256) respectively 7.3684%. Comparing
Fig. 16 with Fig. 13, and comparing the Kittler de-
viations with the EM deviations, reveals that the EM
threshold is more accurate than the Kittler threshold.
REFERENCES
Bilmes, J. (1997). A gentle tutorial on the EM algorithm and
its application to parameter estimation for gaussian
mixture and hidden markov models. Technical Re-
port ICSI-TR-97-021, University of Berkeley, Berke-
ley, CA, USA.
Dempster, A., Laird, N., and Rubin, D. (1977). Maxi-
mum likelihood from incomplete data via the EM al-
gorithm. J. Royal Statist. Soc. Series B (Methodologi-
cal), 39(1):1–38.
Frederix, G. (2005). Beyond Gaussian Mixture Models: Un-
supervised Learning with applications to Image Anal-
ysis. PhD thesis, Katholieke Universiteit of Leuven,
Belgium.
Frederix, G. and pauwels, E. (2004). A statistically princi-
pled approach to histogram segmentation. Technical
Report Report PNA-E0401, CWI, Amsterdam, The
Netherlands.
Gan, R., Chung, A., Wong, W., and Yu, S. (2004a). Vascular
segmentation in three-dimensional rotational angiog-
raphy based on maximum intensity projections. In
Proc. IEEE International Symposium on Biomedical
Imaging: From Nano to Macro, pages 133–136, Ar-
lington, VA, USA.
Gan, R., Wong, W., and Chung, A. (2005). Statistical cere-
brovascular segmentation in three-dimensional rota-
tional angiography based on maximum intensity pro-
jections. Med. Phys., 32(9):3017–3028.
Gan, R., Wong, W., Chung, A., and Yu, S. (2004b).
Statistical cerebrovascular segmentation in three-
dimensional rotational angiography based on maxi-
mum intensity projections. In Proc. CAR, pages 195–
200, Chicago, USA.
Kemkers, R., de Beek, J. O., Aerts, H., Koppe, R., Klotz,
E., Grasse, M., and Moret, J. (1998). 3D-rotational
angiography: First clinical application with use of a
standard philips C-Arm system. In Proc. CAR, pages
182–187, Tokyo, Japan.
Kittler, J. and Illingworth, J. (1986). Minimum error thresh-
olding. Pattern Recognition, 19(1):41–47.
Liao, P., Chen, T., and Chung, P. (2001). A fast algorithm
for multilevel thresholding. Journal of Information
Science and Engineering, 17(5):713–727.
Moret, J., Kemkers, R., de Beek, J. O., Koppe, R., Klotz,
E., and Grass, M. (1998). 3D rotational angiography:
Clinical value in endovascular treatment. Medica-
mundi, 42(3):8–14.
Otsu, N. (1979). A threshold selection method from gray
level histograms. IEEE Trans. Syst., Man, Cybern.,
9(1):62–66.
Philips-Medical-Systems-Nederland (2001). INTEGRIS
3D-RA. instructions for use. release 2.2. Techni-
cal Report 9896 001 32943, Philips Medical Systems
Nederland, Best, The Netherlands.
Wilson, D. and Noble, J. (1999). An adaptive segmentation
algorithm for time-of-flight MRA data. IEEE Trans.
Med. Imag., 18(10):938–945.
VISAPP 2009 - International Conference on Computer Vision Theory and Applications
234