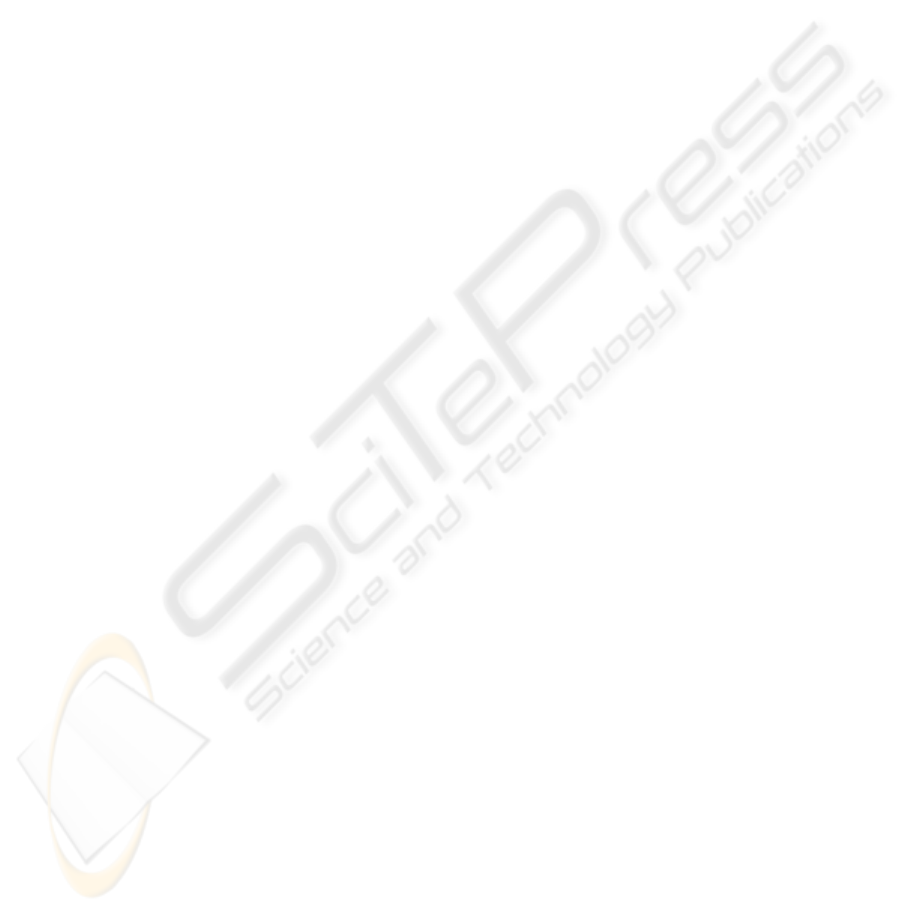
were: simple regression – 4381 models; multiple
regression – 24 models; neural networks – 1329
models; GMDH – 2435 models. The selected
models were included into the committee machines.
We have forecasted diseases and pollutants
values for the period of four years, with a six month
step, and visualized their tendencies, which, in
common, and in agreement with the created models,
are going to overcome the critical levels. Control
under the “significant” factors, which cause impact
upon health indicators, could lead to decrease of
some types of diseases.
5 CONCLUSIONS
The agent-based decision making problem is a
complicated one, especially for a general issue as
environmental impact upon human health. We
should note some essential advantages we have
reached, and some directions for future research.
First, the ADSS supports decision makers in
choosing the behavior line (set of actions) in such a
general case, which is potentially difficult to analyze
and foresee. As for any complex system, ADSS
allows pattern predictions, and the human choice is
to be decisive.
Second, as our work is very time consuming during
the modeling, we are looking forward to both revise
and improve the system and deepen our research.
Third, we consider making more experiments
varying the overall data structure and trying to apply
the system to other but similar application fields.
The ADSS provides all the necessary steps for
standard decision making procedure by using
intelligent agents. The levels of the system
architecture, logically and functionally connected,
have been presented. Real-time interaction with the
user provides a range of possibilities in choosing one
course of action from among several alternatives,
which are generated by the system through guided
data mining and computer simulation. The system is
aimed to regular usage for adequate and effective
management by responsible municipal and state
government authorities.
We used as well traditional data mining
techniques, as other hybrid and specific methods,
with respect to data nature (incomplete data, short
data sets, etc.). Combination of different tools
enabled us to gain in quality and precision of the
reached models, and, hence, in recommendations,
which are based on these models. Received
dependencies of interconnections and associations
between the factors and dependent variables helps to
correct recommendations and avoid errors.
ACKNOWLEDGEMENTS
Marina V. Sokolova is the recipient of a
Postdoctoral Scholarship (Becas MAE) awarded by
the AECI of the Spanish Ministerio de Asuntos
Exteriores y de Cooperación.
REFERENCES
Haykin, S., 1999. Neural Networks: A Comprehensive
Foundation. Prentice-Hall.
Jack™ Intelligent Agents home page.
http://www.agent-software.com/shared/home/.
Liu, L., Qian, L. & Song, H., 2006. Intelligent group
decision support system for cooperative works based
on multi-agent system. In Proceedings of the 10th
International Conference on CSCW in Design,
CSCWD 2006, pp. 574–578.
Madala H.R. & Ivakhnenko A.G. , 1994. Inductive
Learning Algorithms for Complex System Modeling,
CRC Press, ISBN: 0-8493-4438-7.
Ossowski, S., Fernandez, A., Serrano, J.M., Perez-de-la-
Cruz, J.L., Belmonte, M.V., Hernandez, J.Z., Garcia-
Serrano, A. & Maseda, J.M., 2004. Designing
multiagent decision support system: The case of
transportation management. In 3rd International Joint
Conference on Autonomous Agents and Multiagent
Systems, AAMAS 2004, pp. 1470–1471.
Padgham, L. & Winikoff, M., 2004. Developing
Intelligent Agent Systems: A Practical Guide. John
Wiley and Sons.
Padgham, L. & Winikoff, M., 2002. Prometheus: A
pragmatic methodology for engineering intelligent
agents. In Proceedings of the Workshop on Agent
Oriented Methodologies (Object-Oriented
Programming, Systems, Languages, and
Applications), pp. 97-108.
Petrov, P.V. & Stoyen, A.D., 2000. An intelligent-agent
based decision support system for a complex
command and control application. In Sixth IEEE
International Conference on Complex Computer
Systems, ICECCS’00, pp. 94–104.
Prometheus Design Tool home page.
http://www.cs.rmit.edu.au/agents/pdt/.
Sokolova, M.V. & Fernández-Caballero, A., 2007. A
multi-agent architecture for environmental impact
assessment: Information fusion, data mining and
decision making. In 9th International Conference on
Enterprise Information Systems, ICEIS 2007, vol. 2,
pp. 219-224.
Urbani, D. & Delhom, M., 2005. Water management
policy selection using a decision support system based
on a multi-agent system. In Lecture Notes in Computer
Science, 3673, pp. 466–469.
Weiss, G., 1999. Multi-agent Systems: A Modern
Approach to Distributed Artificial Intelligence. The
MIT Press.
DATA MINING DRIVEN DECISION MAKING
225