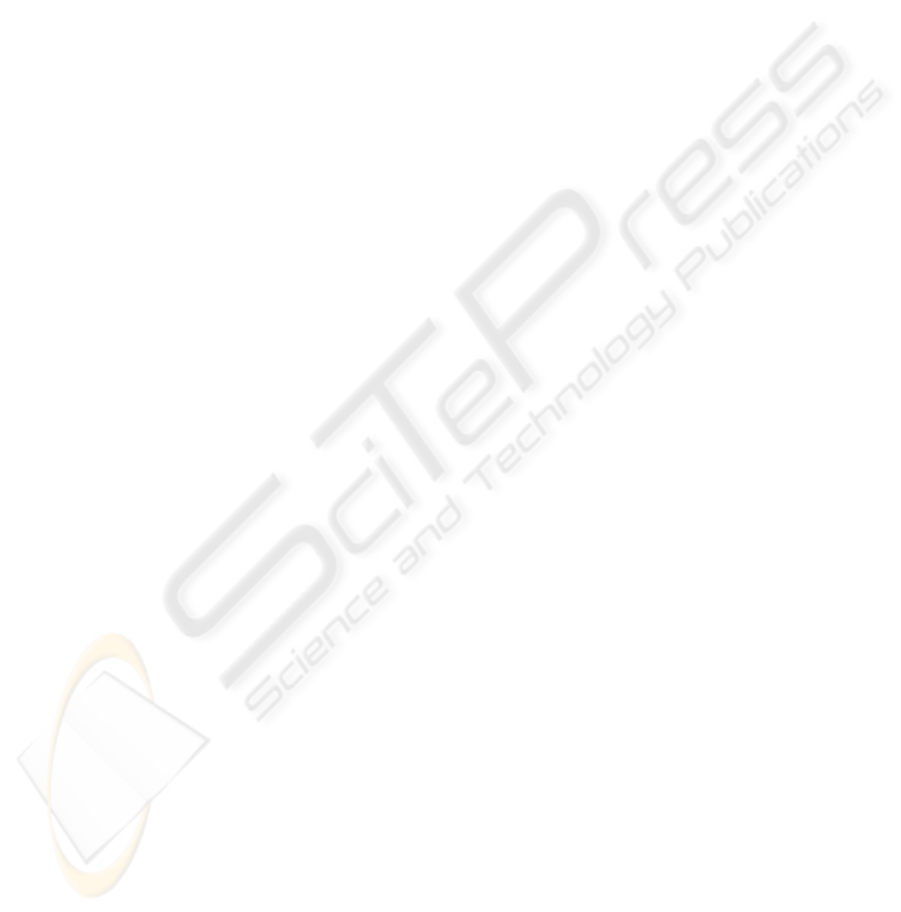
Apparently, the detection performances on the
feature sets with COMB features are better than the
corresponding feature sets with Markov features.
6 CONCLUSIONS
In this paper, we expand the well-known Markov
features into the neighboring on the inter-blocks of
the DCT domain and the wavelet domain. We also
propose the joint distribution features of the
differential neighboring in the DCT domain and the
DWT domain, and calculate the difference of these
features from the testing image and the calibrated
version. We successfully improve the blind
steganalysis performance in multi-class JPEG
images. Since different hiding systems show
different sensitivities to the same feature set, a
method for selecting the optimal feature set is
critical to maximize detection performance, and this
topic is being addressed and it is possible to come
out in the final version of this manuscript.
ACKNOWLEDGEMENTS
The authors would like to acknowledge the support
for this research from ICASA (Institute for Complex
Additive Systems Analysis, a division of New
Mexico Tech).
REFERENCES
Duda, R., Hart, P. and Stork, D., 2001. Pattern
Classification, 2ed. New York, NY: Wiley.
Fridrich, J., 2004. Feature-Based Steganalysis for JPEG
Images and its Implications for Future Design of
Steganographic Schemes, Lecture Notes in Computer
Science, vol. 3200, Springer-Verlag, pp. 67-81.
Fridrich J., Goljan, M. and Hogeam, D., 2002.
Steganalysis of JPEG Images: Breaking the F5
Algorithm. Proc. of 5
th
Information Hiding Workshop,
pp. 310-323.
Guyon, I., Weston, J., Barnhill, S. and Vapnik, V., 2002.
Gene Selection for Cancer Classification using
Support Vector Machines. Machine Learning, 46(1-
3):389-422.
Harmsen, J and Pearlman, W., 2003, Steganalysis of
Additive Noise Modelable Information Hiding. Proc.
of SPIE Electronic Imaging, Security, Steganography,
and Watermarking of Multimedia Contents V. 5020,
pp.131-142.
Harmsen, J. and Pearlman, W., 2004. Kernel Fisher
Discriminant for Steganalysis of JPEG Hiding
Methods. Proc. of SPIE, Security, Steganography,
and Watermarking of Multimedia Contents VI, vol
5306, pp.13-22.
Hetzl, S. and Mutzel, P., 2005. A Graph-Theoretic
Approach to Steganography. Lecture Notes in
Computer Science, vol. 3677, pp. 119-128.
Katzenbeisser, S. and Petitcolas, F., 2000. Information
Hiding Techniques for steganography and Digital
Watermarking. Artech House Books.
Ker, A., 2005. Improved Detection of LSB Steganography
in Grayscale Images. Lecture Notes in Computer
Science, Springer-Verlag, 3200, 2005, pp.97-115.
Liu, Q. and Sung, A., 2007. Feature Mining and Nuero-
Fuzzy Inference System for Steganalysis of LSB
Matching Steganography in Grayscale Images. Proc.
of 20
th
International Joint Conference on Artificial
Intelligence, pp. 2808-2813.
Liu Q, Sung A, Chen, Z and Xu J, 2008a. Feature Mining
and Pattern Classification for Steganalysis of LSB
Matching Steganography in Grayscale Images. Pattern
Recognition, 41(1): 56-66.
Liu, Q., Sung, A., Xu, J. and Ribeiro, B., 2006. Image
Complexity and Feature Extraction for Steganalysis of
LSB Matching Steganography., Proc. of 18
th
International Conference on Pattern Recognition,
ICPR (1), pp. 1208-1211.
Liu, Q., Sung, A., Ribeiro, B., Wei, M., Chen, Z. and Xu,
J., 2008b. Image Complexity and Feature Mining for
Steganalysis of Least Significant Bit Matching
Steganography, Information Sciences, 178(1): 21-36.
Lyu, S. and Farid, H., 2005. How Realistic is
Photorealistic. IEEE Trans. on Signal Processing,
53(2): 845-850.
Marvel, L., Boncelet, C. and Retter, C., 1999. Spread
Spectrum Image Steganography. IEEE Trans. Image
Processing, 8(8): 1075-1083.
Pevny, T. and Fridrich, J., 2007. Merging Markov and
DCT Features for Multi-Class JPEG Steganalysis.
Proc. SPIE Electronic Imaging, Electronic Imaging,
Security, Steganography, and Watermarking of
Multimedia Contents IX, vol. 6505.
Sallee P, 2004. Model based steganography. Lecture Notes
in Computer Science, vol. 2939, pp. 154-167.
Sharifi K and Leon-Garcia A, 1995. Estimation of Shape
Parameter for Generalized Gaussian Distributions in
Subband Decompositions of Video. IEEE Trans.
Circuits Syst. Video Technol., 5: 52-56.
Shi, Y., Chen, C. and Chen, W., 2007. A Markov process
based approach to effective attacking JPEG
steganography. Lecture Notes in Computer Sciences,
vol.437, pp.249-264.
Vapnik, V., 1998. Statistical Learning Theory, John
Wiley.
Westfeld, A., 2001. High Capacity Despite Better
Steganalysis (F5–A Steganographic Algorithm).
Lecture Notes in Computer Science, vol. 2137, pp.289-
302.
USING EXPANDED MARKOV PROCESS AND JOINT DISTRIBUTION FEATURES FOR JPEG STEGANALYSIS
231