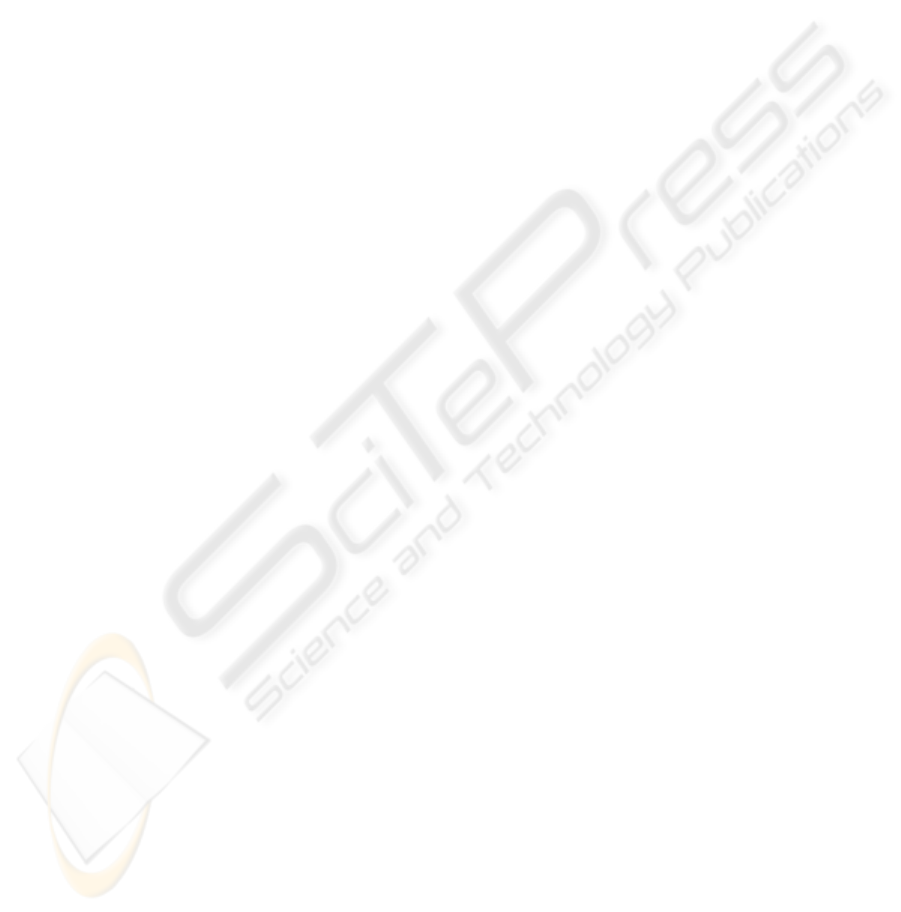
dimensions; we can notice that this effect should
probably be corrected by adjusting correctly the
global trust computation function.
But, globally, we can conclude from these
experiments that the two experimental criteria that
we had expressed are satisfied, and so, that our
model seems to be an adequate solution to the
problem we wanted to address.
5 CONCLUSIONS AND
PERSPECTIVES
In this work, we have explored the possibility of
associating trust and personalization paradigms in an
agent network. We have done this in order to give to
agents the ability to handle both the intrinsic
uncertainty of a partial-knowledge, evolving
network, and the also evolving requirements of a
user’s set of preferences. Indeed, agents would have
to face them both in a social network in which each
user has one or more agent to represent him.
Knowing that just putting together the two
reasoning methods leads to heavy problems of
optimization but also to problems for mixing the
results given by each one, we have looked for a
solution to integrate both notions in a single
reasoning process. We first gave some theoretical
criteria to choose every component of a global
agent’s reasoning method that could handle both
trust and personalization: the trust model, the
personalization model and the integration model. We
then proposed a complete solution that is acceptable
according to those criteria.
Our solution involves the Falcone and
Castelfranchi trust model, to which we added a new
trust dimension, that we called degree of similarity.
It also involves a cardinal preferences model such as
weighted conjunction of literals, which is used by
agents to evaluate results and alternatives and learn
the degree of similarity they have with other ones.
The obtained experimental results for
optimization and accuracy criteria seemed to
validate these criteria. That is why even if these
experimentations were done on a simplified version
of the trust and personalization integration model,
we can say that the solution we proposed seems to
be viable and to be applicable to the kind of
networks we described. This model was developed
in order to improve the behavior of agents in these
open, partial-knowledge and user centered networks,
and it seems to achieve this goal.
Future work on this solution is to test it with a
full and multi-source implementation with dynamic
personalization from real users. As the Falcone and
Castelfranchi model is very powerful and because of
the large scale of different cardinal preference
implementations that can fit in the theoretical criteria
of this solution, we can foresee very different
solutions for various domains and the need to find
the adequate personalization evaluation and trust
evaluation functions to each model.
REFERENCES
Camps, V., & Gleizes, M.-P., Principes et évaluation d'une
méthode d'auto-organisation. 3
èmes
Journées
Francophones IAD & SMA, pp. 337-348. St Baldoph,
1995.
Castelfranchi, C., & Falcone, R., Principles of trust for
mas : cognitive anatomy, social importance, and
quantification. 3rd Int. Conf. on Multi-Agent Systems,
ICMAS’98, pp. 72-79, Paris, 1998.
Castelfranchi, C., & Falcone, R. Trust dynamics : How
trust is influenced by direct experiences and by trust
itself. 3rd Int. J. Conf. on Autonomous Agents and
Multiagent Systems, AAMAS’04, 2. New-York, 2004.
Endriss, U., Preference Representation in Combinatorial
Domains. Institute for Logic, Language and
Computation, Univ. of Amsterdam, 2006.
Gauch, S., Speretta, M., Chandramouli, A., & Micarelli,
A., User Profiles for Personalized Information Access.
The Adaptive Web, pp. 54-89, 2007.
Maximilien, E. M., & Singh, M. P., Agent-Based Trust
Model Involving Multiple Qualities. 4th Int. J. Conf.
on Autonomous Agents and Multiagent Systems,
AAMAS’05, Utrecht, 2005.
Melaye, D., Demazeau, Y. & Bouron, Th. “Which
Adequate Trust Model for Trust Networks?”, 3
rd
IFIP
Conference on Artificial Intelligence Applications and
Innovations, AIAI’2006, eds, IFIP, Athens, 2006.
Montaner, M., López, B., & De La Rosa, J. L., A
Taxonomy of Recommender Agents on the Internet.
Artificial Intelligence Review , 19, pp. 285–330, 2003.
Rao, A., & Georgeff, M. BDI Agents : From theory to
practice, Tech. Rep. 56, Australian AI Institute,
Melbourne, 1995.
PERSONALIZATION OF A TRUST NETWORK
415