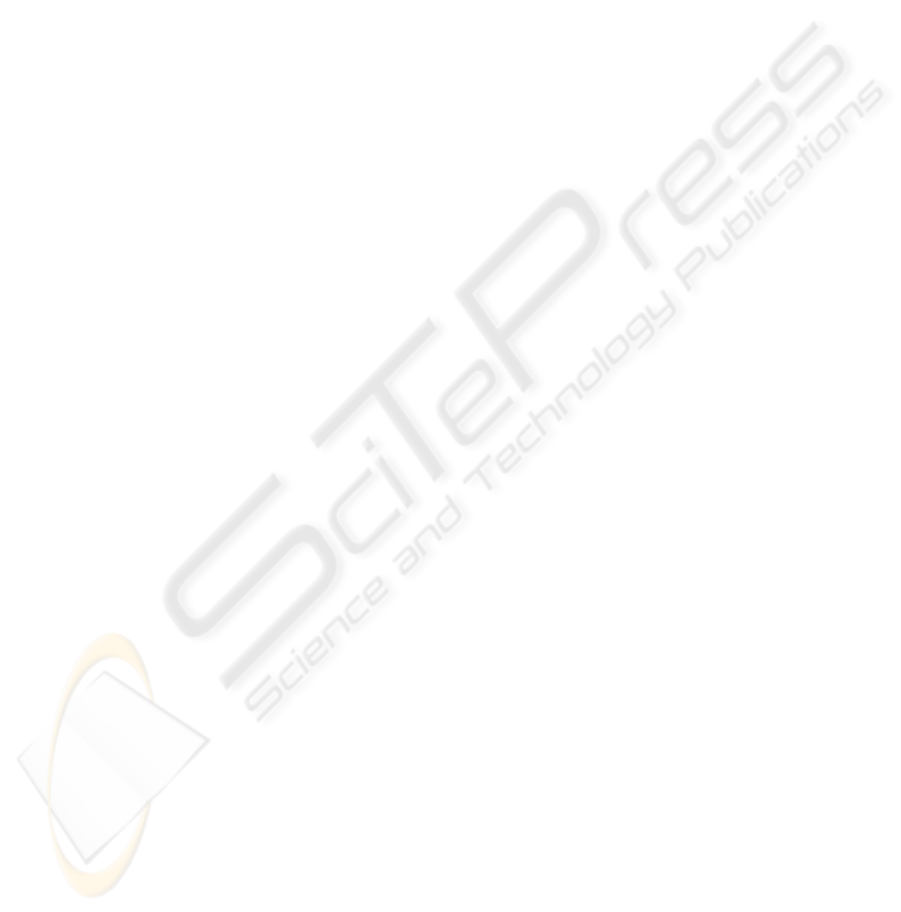
Moreover, BNCLVQ is easily extended to fuzzy
case to solve the prototype under-utilization problem,
i.e., only the BMU is updated for each input (Zhang
et al., 2004), simply replacing the indicative function
by the membership function (Bezdek, 1981). The
membership assignment of fuzzy projection implies
the specification to classes, and can be used for the
validity estimation of classification (Vuorimaa, 1994).
In the future work, the impact of overfitting prob-
lem will be further studied using early stopping strat-
egy on an independent data set and the benefit of
fuzzy strategies in BNCLVQ will be investigated by
cross-validation experiments on both UCI data sets
and state-of-art real world problems. In a first real
world case study, we are currently applying NCSOM
topological maps to fine tune mixed numeric and cat-
egorical data in a natural language processing prob-
lem (Marques et al., 2007). In this domain we have
some pre-labeled data available and NCSOM is help-
ing to investigate accurateness and consistency of
manual data labeling. However, already known cor-
rect cases (and possible some previously available
prototypes) should be included in the previously NC-
SOM trained topological map. For that we intend to
use BNCLVQ as the appropriate tool. According to
our results, BNCLVQ can achieve good precision in
most domains. Moreover BNCLVQ is more than a
classification algorithm. Indeed BNCLVQ is also a
fine-tuning tool for topological features maps, and,
consequently, a tool that will help the data mining
process when some labeled data is available.
ACKNOWLEDGEMENTS
This work was supported by project C2007-
FCT/442/2006-GECAD/ISEP (Knowledge Based,
Cognitive and Learning Systems).
REFERENCES
Asuncion, A. and Newman, D. J. (2007).
UCI machine learning repository. URL:
http://www.ics.uci.edu/∼mlearn/MLRepository.html.
Bader, S., Holldobler, S., and Marques, N. (2008). Guid-
ing backprop by inserting rules. In d’Avila Garcez,
A. S. and Hitzler, P., editors, Proceeding of 18th Eu-
ropean Conference on Artificial Intelligence, the 4th
International Workshop on Neural-Symbolic Learning
and Reasoning, volume 366 of ISSN 1613-0073, Pa-
tras, Greece.
Bezdek, J. C. (1981). Pattern recognition with fuzzy objec-
tive function algorithms. Plenum Press, New York.
Chen, N. and Marques, N. C. (2005). An extension of self-
organizing maps to categorical data. In Bento, C., Car-
doso, A., and Dias, G., editors, EPIA, volume 3808 of
Lecture Notes in Computer Science, pages 304–313.
Springer.
Geng, X., Zhan, D.-C., and Zhou, Z.-H. (2005). Su-
pervised nonlinear dimensionality reduction for vi-
sualization and classification. IEEE Transactions on
Systems, Man, and CyberneticsCPart B: Cybernetics,
35(6):1098–1107.
Huang, Z. (1998). Extensions to the k-means algorithms
for clustering large data sets with categorical values.
Data Mining and Knowledge Discovery, 2:283–304.
Kohonen, T. (1997). Self-organizing maps. Springer Verlag,
Berlin, 2nd edition.
Kohonen, T. (2005). Som toolbox 2.0. URL:
http://www.cis.hut.fi/projects/somtoolbox/.
Kohonen, T. and Somervuo, P. (1998). Self-organizing
maps of symbol strings. Neurocomputing, 21(10):19–
30.
Kotsiantis, S. B. (2007). Supervised machine learning:
a review of classification techniques. Informatica,
31:249–268.
Marques, N., Bader, S., Rocio, V., and Holldobler, S.
(2007). Neuro-symbolic word tagging. In Proceed-
ings of 13th Portuguese Conference on Artificial In-
telligence (EPIA’07), 2nd Workshop on Text Mining
and Applications, Portugal. IEEE Guimaraes.
Matheus, C. (1990). Adding domain knowledge to sbl
through feature construction. In Proceedings of the
Eighth National Conference on Artificial Intelligence,
pages 803–808, Boston. MA: AAAI Press.
Silva, B. and Marques, N. C. (2007). A hybrid parallel
som algorithm for large maps in data-mining. In Pro-
ceedings of 13th Portuguese Conference on Artificial
Intelligence (EPIA’07), Workshop on Business Intelli-
gence, Portugal. IEEE Guimaraes.
Solaiman, B., Mouchot, M. C., and Maillard, E. (1994).
A hybrid algorithm (hlvq) combining unsupervised
and supervised learning approaches. In Proceed-
ings of IEEE International Conference on Neural Net-
works(ICNN), pages 1772–1778, Orlando, USA.
Somervuo, T. and Kohonen, T. (1999). Self-organizing
maps and learning vector quantization for feature se-
quences. Neural Processing Letters, 10(2):151–159.
Stephen, D. B. (1999). Nearest neighbor classification from
multiple feature subsets. Intelligent Data Analysis,
3(3):191–209.
Vuorimaa, P. (1994). Use of the fuzzy self-organizing
map in pattern recognition. In Proceedings of the
Third IEEE Conference on Computational Intelli-
gence, pages 478–801.
Witten, H. and Frank, E. (2005). Data mining: practical
machine learning tools and techniques. Morgan Kauf-
mann, San Francisco, 2nd edition.
Zhang, D., Chen, S., and Zhou, Z.-H. (2004). Fuzzy-kernel
learning vector quantization. In Yin, F., Wang, J., and
Guo, C., editors, ISNN (1), volume 3173 of Lecture
Notes in Computer Science, pages 180–185. Springer.
ICAART 2009 - International Conference on Agents and Artificial Intelligence
84