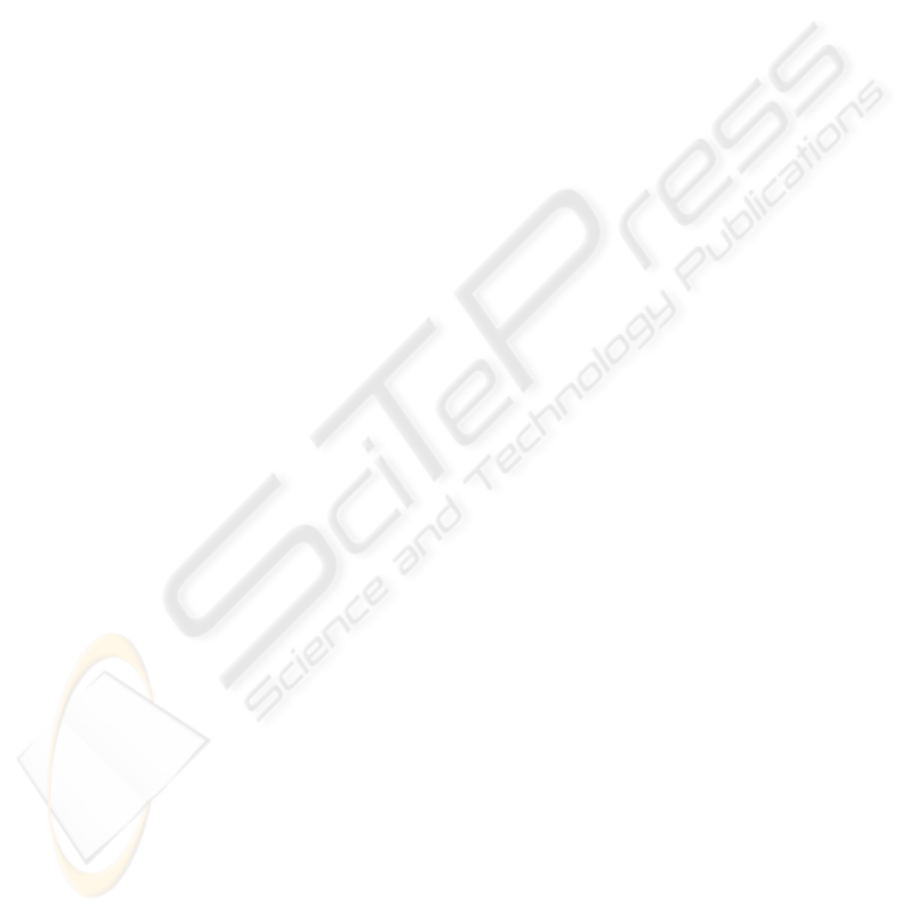
7 CONCLUSIONS AND FUTURE
RESEARCH
In this paper we proposed an ontology-base
summarization system that can abstract key concepts
and can extract key sentences to summarize text
documents including Web pages. We introduced
unique methods that have two advantages over
existing methods. One advantage is the use of multi-
level upward propagation to solve word sense
disambiguation problem. The other is that the
propagation process provides a method for the
generalization of concepts. We have implemented
and tested the proposed system. Our test results
show that the system is able to abstract key
concepts, generalize new concepts, and extract key
sentences. In addition to summarization of
documents, the system can be used for semantic
Web, information retrieval, and knowledge
discovery applications.
Based on our approaches, there are great
potentials for future research. One challenging
research is to create new abstract sentences to
summarize a document. In this task, we are requiring
computers to write meaningful sentences. This is not
an easy task. We have been working on this task for
years. Now, we are able to create simple sentences.
We will report this work after more testing and fine-
tuning. We are also working to incorporate
automatic Web page summarization with Web page
classification (Choi & Yao, 2005) and clustering
(Yao & Choi, 2007) to create the next generation of
search engine (Choi, 2006). Much research remains
to be done to address the problem of information
overload and to make effective use of information
contained on the Web.
REFERENCES
Barzilay R. and Elhadad M, “Using lexical chains for text
summarization,” Proceedings of the ACL Workshop on
Intelligent Scalable Text Summarization, pp. 10-17,
1997.
Cañas A. J., Valerio A, Lalinde-Pulido J., Carvalho M, &
Arguedas M., “Using WordNet for Word Sense
Disambiguation to Support Concept Map
Construction,” Lecture Notes in Computer Science:
String Processing and Information Retrieval, Vol.
2857/2003, pp. 350-359, 2004.
Choi B. & Yao Z., “Web Page Classification,”
Foundations and Advances in Data Mining, Springer-
Verag, pp. 221 - 274, 2005.
Choi B., “Method and Apparatus for Individualizing and
Updating a Directory of Computer Files,” United
States Patent # 7,134,082, November 7, 2006.
Cycorp, ResearchCyc, http://research.cyc.com/,
http://www.cyc.com/, 2008.
Doran W., Stokes N., Carthy J., & Dunnion J.,
“Comparing lexical chain-based summarisation
approaches using an extrinsic evaluation,” In Global
WordNet Conference (GWC), 2004.
Hahn U. & Mani I., “The Challenges of Automatic
Summarization”, IEEE Computer, Vol. 33, Issue 11,
pp. 29-36, Nov. 2000.
Kupiec J., Pedersen J., & Chen F., “A Trainable
Document Summarizer,” In Proceedings of the
Eighteenth Annual International ACM Conference on
Research and Development in Information Retrieval
(SIGIR), 68–73. Seattle, WA, 1995.
Lin C.Y., “ROUGE: A Package for Automatic Evaluation
of Summaries,” Text Summarization Branches Out:
Proceedings of the ACL-04 Workshop, Barcelona,
Spain, pp. 74-81, July, 2004.
Mann W.C. & Thompson S.A., “Rhetorical Structure
Theory: Toward a Functional Theory of Text
Organization,” Text 8(3), 243–281. Also available as
USC/Information Sciences Institute Research Report
RR-87-190, 1988.
Manning C. & Jurafsky D., The Stanford Natural
Language Processing Group, The Stanford Parser: A
statistical parser, http://nlp.stanford.edu/software/lex-
parser.shtml, 2008.
Mittal V.O. & Witbrock M. J., "Language Modeling
Experiments in Non-Extractive Summarization,"
Chapter 10 in Croft, W. Bruce and Lafferty, John,
Language Modeling for Information Retrieval, Kluwer
Academic Publishers, 2003.
NIST, “Text Analysis Conference”,
http://www.nist.gov/tac/, National Institute of
Standards and Technology, 2008.
Salton G., Singhal A., Mitra M., & Buckley C.,
“Automatic text structuring and summarization,”
Information Processing and Management, 33, 193-20,
1997.
Silber G. & McCoy K., “Efficiently Computed Lexical
Chains as an Intermediate Representation for
Automatic Text Summarization,” Computational
Linguistics, 2002.
Simón-Cuevas1 A., Ceccaroni L., Rosete-Suárez A.,
Suárez-Rodríguez A., & Iglesia-Campos, M., “A
concept sense disambiguation algorithm for concept
maps,” Proc. of the Third Int. Conference on Concept
Mapping, Tallinn, Estonia & Helsinki, Finland 2008.
Teufel S. & Moens M., “Sentence Extraction as a
Classification Task,” In Proceedings of the Workshop
on Intelligent Scalable Summarization. ACL/EACL
Conference, 58–65. Madrid, Spain, 1997.
Yao Z. & Choi B., “Clustering Web Pages into
Hierarchical Categories,” International Journal of
Intelligent Information Technologies, Vol. 3, No. 2,
pp.17-35, April-June, 2007.
ICAART 2009 - International Conference on Agents and Artificial Intelligence
286