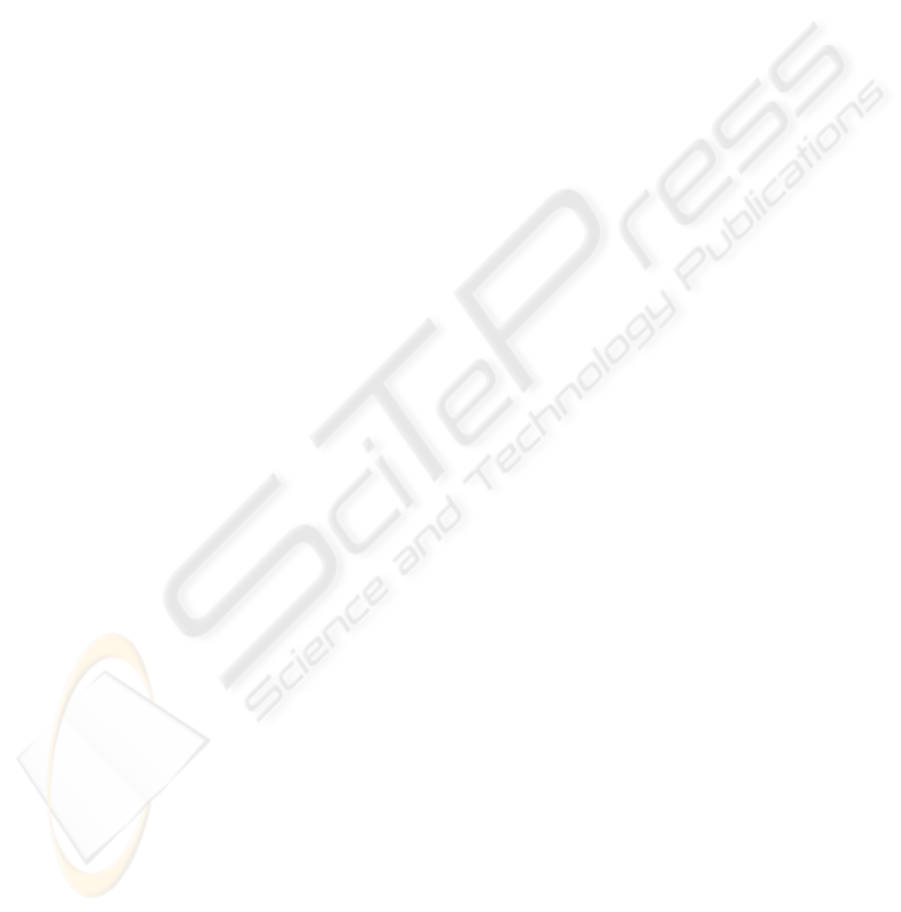
sent to the “Linking Agent 1” (step 5). For example,
the reactive agent C monitors an eye gaze
component. The reports about the application of the
task are sent to the reasoning agent in order to be
analyzed (step 6). The reasoning agent contains the
rule on the modalities. If the condition, on the
modalities, is not satisfied, the stimulus condition is
activated and the application of the role is started, by
consequence, the new task “task 2” is sent to the
linking agent (step 7). The linking agent applies the
“task 2”: primitives actions are sent to the reactive
agent (step 8). The actions are accomplished by the
reactive agents (Agents E, F and G) (step 9). The
feedback of the last actions is accomplished by the
reactive agent D (step 10). The information is
charged to reports and sent up to the reasoning agent
through the linking agent (step 11). Finally, the sum
of the reports is sent to the manager agent in order to
be transformed to documentations. Safety of the MA
and priority of the others scenarios are continually
checked during the gradual process of
reconfiguration.
2.2 Example of Quality Tradeoff
In real situations, we don’t know in which hardware
the M application will be installed (the CPU
performance, memory performance, etc. are
unknown). The EAg must maintain the software
performances regarding to user’s impairment and
hardware’s performances of the computer, for
example. In this scenario, the EAg employs the
modalities’ activations to drive up the application in
a resource-less way. Clearly, certain modalities
consume more resources than others. The scenario 1
specifies to the EAg to choose the modalities that
consume less resources in order to maintain a high
degree of performance in the M application. Another
scenario (named scenario 2) identifies a major goal
of one characteristic of usability that the expert
agent can maintain. But in certain cases one
characteristic is not sufficient to assume acceptable
work of the application. Priority of the scenarios is
then used and parameterized by the users (also a
default parameterization is available in the EAg). In
our example case, the Scenario 2 has priority 8/10,
whereas the scenario 1 (performance) has priority
10/10. The comparison between the two qualities is
occurred in a section “quality trade-off” defined in
each scenario. Automatically, the EAg executes the
scenario 2 at detriment of the scenario 1 (if the
condition of the trade-off quality is satisfied). In the
scenario 1, the expert agent monitors the consumed
resources, if the CPU charges exceed the 60% the
scenario 1 is applied and scenario 2 is stopped. For
example, if we use the computer including Pentium
III with 850 MHz CPU frequency, and if the user
some modalities, the scenario 1 is executed (because
the consumed resources are 70%). The Scenario 1
starts procedures to activate the modality belonging
to the same group of the eye gaze system like haptic
screen to satisfy the requirement for the performance
of the application. In this case, the M application
consumes 30% of resources. The EAg was modeled
in CPN-tools for the two scenarios. The results of
the simulation of the nets are presented at Figure 2.
A reasoning agent 2 (like the one in Figure 1 but for
scenario 1) randomly inhibits one of the three
modalities involved in the process described by the
scenario 1, when the CPU charge exceeds 100%,
with respect to the constraints of the M interaction.
The network is conceived for always guaranteeing
that the CPU resources are not saturated when the M
events occur (in parallel or sequential) and doesn’t
disturb the running interaction in the MA. It thus
fulfils its function of monitoring and control in an
intelligent and automatic way. The intelligence
comes from the fact that we know that the agent will
carry out its role, even if we are not able to affirm, in
advance, at every moment, in a procedural way,
which of the modalities will be inhibited by the
agent. The automatism comes from the behavioral
cyclic invariants in the Petri nets which allow to
control and to monitor the MA. While the scenario 1
is performed, the EAg supervises and controls the
input modalities and applies the scenario 2. The part
of the EAg, in charge of the application of the
scenario 2, uses the beginning instant of the events
captured on the input modalities and their respective
durations for performing activation and/or inhibition
rules on the antagonist modalities. The A of the
network is an automaton which applies the scenario
2 with respect to cyclic invariants. When inhibition
actions are applied by the EAg to modalities, the
cognitive agent sends messages to the user. During
the step by step simulation of the networks with
CPN-Tools (Jensen et al. 2008), we observe the
correct behavior of the EAg performing the scenario
1 and the scenario 2. The graphs on Figure 2 show,
during time, how the EAg reacts to the variation of
the input signals. Those signals are the input
modalities' ones. The inhibitor signals in Figure 2
are generated by the EAg, with respect to the MA’s
reliability, to apply the scenarios. CPN-ML
inscriptions on the various arcs, in the codes
(performed by the transitions), of conditions
(checked by the transitions) and also the dynamic
links (arcs) between the various instances of agents
ADAPTATIVE MULTIMODAL ARCHITECTURES MANAGING SOFTWARE QUALITIES
351