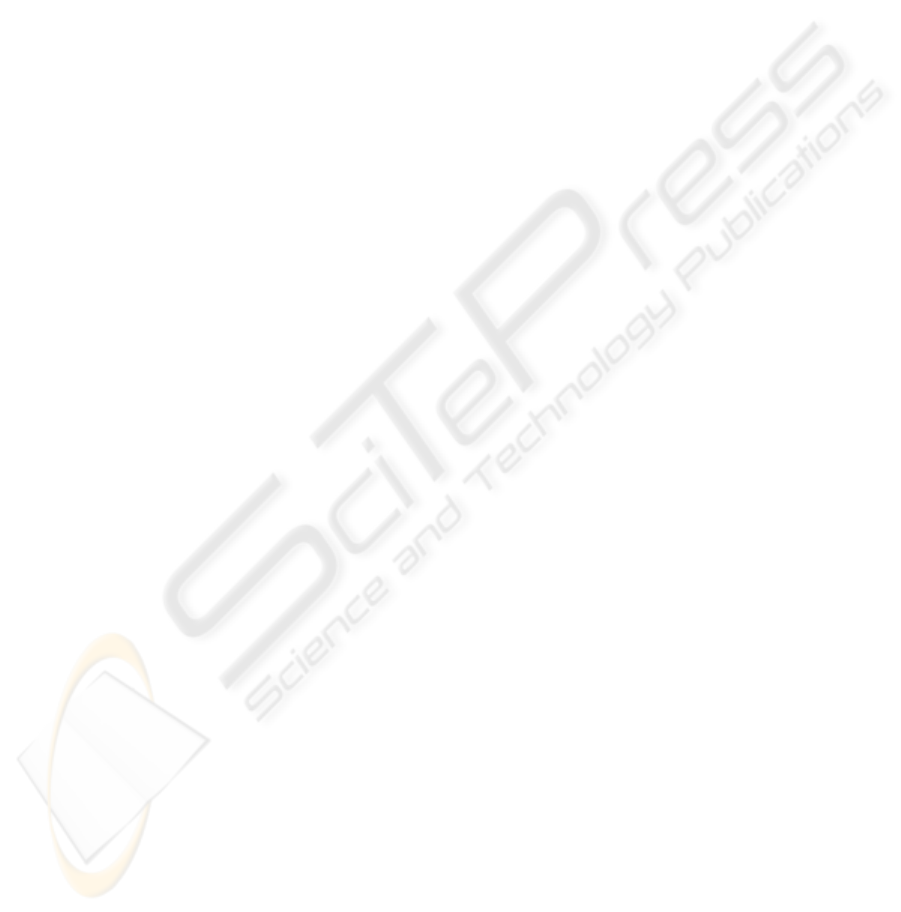
ke. Moreover, there are also psychological factors
influencing the actual level of vigilance, e.g. motiva-
tion, stress, and monotony. The last is believed to
play a major role in driving, because it is mostly a
simple lane-tracking task with a low event rate.
Therefore, vigilance is considered as a psychophy-
siological variable not always increasing monotoni-
cally during driving. It shows slow waxing and
waning patterns, which can be observed in driving
performance and repeatedly self-reported sleepiness.
There are many biosignals which contain more
or less information on hypovigilance. Among them,
EEG is a relatively direct, functional reflection of
mainly cortical and to some low degree also sub-
cortical activities. EOG is a measure of eye and
eyelid movements and reflects activation / deactiva-
tion as well as regulation of the autonomous nervous
system.
Until recently, for the assessment of driver’s hy-
povigilance the analysis of EEG and EOG was based
on a variety of definitions involving PSD summation
in a few spectral bands which proved in clinical pra-
ctice. The same applies to the location of EEG elec-
trodes. Separate analysis of EEG of different electro-
des and of alternative definitions of spectral bands
led to inconsistent and sometimes contradicting re-
sults. Large inter-individual differences turned out to
be another problematic issue.
Therefore, adaptive methods with less predefi-
ned assumptions are needed for comprehensive hy-
povigilance assessment. Here we propose a combi-
nation of different brain (EEG) and oculomotoric
(EOG) signals whereby parameters of pre-proces-
sing and summation in spectral bands were optimi-
zed empirically. Moreover, modern concepts of dis-
criminant analysis such as computational intelligen-
ce and concepts of data fusion were utilized. Using
this general approach ensures optimal information
gain even if unimodal data distributions are existent
(Golz et al. 2007).
As a first step solution, we utilized SVM in order
to map feature vectors extracted from EEG / EOG of
variable segment lengths to two, independent types
of class labels. For their generation a subjective as
well as an objective measure was applied. Both ref-
lect different facets of hypovigilance: sleepiness and
performance decrements, respectively.
For the first type of labels, an orally spoken self-
report of sleepiness on a continuous scale, the so-
called Karolinska Sleepiness Scale (KSS), was
recorded every two minutes during driving. The
second type of labels was determined through
analyzing driving performance. In previous studies it
was found that especially the variation of lane
deviation (VLD) correlates well with hypovigilance
and attention state of drivers (Pilutti et al. 1999).
2 METHODS
2.1 Experiments
16 participants drove two nights (11:30 p.m. – 8:30
a.m.) in our real car driving simulation lab. One
overnight experiment comprised of 8 x 40 min of
driving. EEG (FP1, FP2, C3, Cz, C4, O1, O2, A1,
A2) and EOG (vertical, horizontal) were recorded at
a sampling rate of 256 Hz. PERCLOS as another
oculomotoric measure was recorded utilizing an
established eye tracking system at a sampling rate of
60 Hz. Also several variables of driving simulation,
like e. g. steering angle and lane deviation, were re-
corded at a sampling rate of 50 Hz. Lane deviation is
a good measure of driving performance and is used
here as an objective and independent measure of
hypovigilance as described below. Variation of lane
deviation (VLD) is the difference between two sub-
sequent samples of lane deviation normalized to the
width of lane. For example, moving the car from the
left most to the right most position of the lane results
in VLD = 100 %. The KSS was mentioned above
and is a standardized, subjective, and independent
measure of hypovigilance on a numeric scale bet-
ween 1 and 10. KSS was asked at the beginning and
after finishing driving. During driving only relative
changes in percent of the full range were asked
because subjects are more aware of relative than on
absolute changes.
2.2 Procedure Steps
To allow a comparison of the selected biosignals
regarding hypovigilance, pre-possessing and feature
extraction were performed due to the same concept
for all biosignals (Golz et al. 2007). First, non-over-
lapping segmentation with variable segment length
was carried out, followed by linear trend removal
and estimation of power spectral densities (PSD)
utilizing the modified periodogram method. Other
estimation techniques, such as Welch’s method, the
Multi-Taper method, and a parametric estimation
(Burg method), were also applied, but resulted in
slightly higher discrimination errors. It seems that
these three methods failed due to reduced variance
of PSD estimation at the expense of bias. In contra-
diction to explorative analysis, machine learning
algorithms are not such sensitive to higher variances.
Second, PSD values of all three types of signals
DETERMINATION OF DRIVER’S HYPOVIGILANCE FROM BIOSIGNALS
131