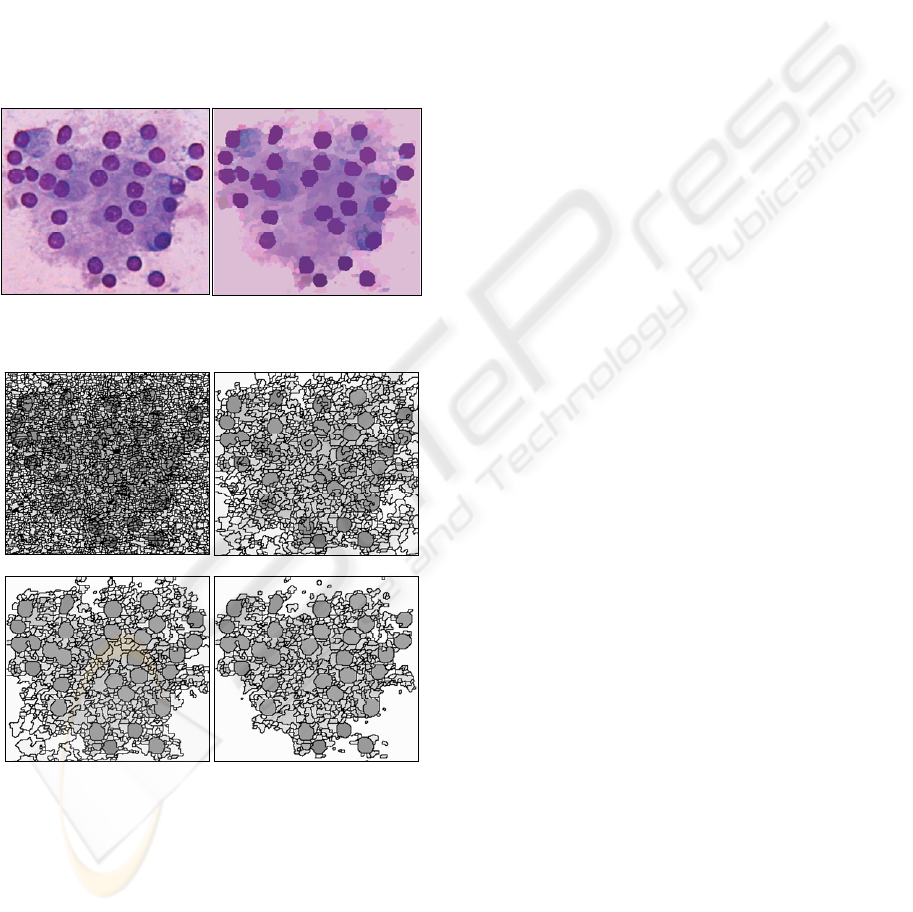
region borders showing the ability of the proposed
segmentation scheme to simplify the original image.
The use of morphological process as opening and
closing operations applied on the RAG yields an
interesting feature extraction as the photometric
value, the area, the perimeter, the compactness
factor, the number of neighbors of a region and their
relationship for each region. This description
represents the simplified information and contains
potentially an elaborate knowledge. After the
interpretation of the image, a list of retained objects
and their associated features are stored in an XML
(eXtensible Markup Langage) file and ready to be
integrated into a medical information system.
Figure 2: The original RGB image and its corresponding
segmented image.
(a)
(b)
(d)
(c)
Figure 3: Hierarchical RAG levels shown the step of
region merging.
6 CONCLUSIONS
We have proposed a new method of microscopic
medical images segmentation using mathematical
morphology applied on RAG and an automatic
clustering method followed by a regularization step
using an automatic hypercube classification. Due to
the unsupervised nature of the procedure especially
the use of automatic thresholds detection, it can be
reliable to the huge variability of intensities and
shapes of the image regions and will be tested as a
part of future work in other color space without
introducing
a priori knowledge and pre-processing
stages.
Results show the effectiveness of our method for
medical image applications as cytology images and
the impact that it introduces on the semantic high
level search for any disease or abnormal cells.
In this paper, the morphological operations
consider only the extrema of region neighborhood.
For future works, we will pursue the aggregation
operations beyond the limits presented by the
morphological processing avoiding the refinement
segmentation step that uses the hypercube
classification.
REFERENCES
Lucchese, L., Mitra, S.K., 2001. Color image
segmentation: A state-of-the art survey. In Image
Process. Vis. Pattern Recog. Proc. Indian Nat. Sci.
Acad. (INSA-A). Vol67 i2.pp. 207-221.
Lezoray, O., Lecluse, M., November 2007. Automatic
segmentation and classification of cells from broncho
alveolar lavage. In Image Analysis and Stereology,
Vol.26, pp. 111-119.
Lezoray, O., Juin 2003. Supervised automatic histogram
clustering and watershed segmentation. Application to
microscopic medical images. In Image Analysis and
Stereology, Vol. 22, Numéro 2, pp.113-120.
El-Khoury, E., Senac, C., André-Obrecht, R., 2007.
Speaker Diarization: Towards a more Robust and
Portable System. Int. Conf. On Acoustics, Speech, and
Signal Processing (ICASSP’2007), Honolulu, Hawaii,
USA.
Hirata Jr., R., César Flores, F., Barrera, J., Lotufo, R.,
Meyer, F., 2000. Color Image Gradients for
Morphological Segmentation. In XIII Brizilian
Symposium on Computer Graphics and Image
Processing (SIBGRAPI'00), pp.316-326.
Beucher, S., Lantuéjoul, C., Sept. 1979. Use of watersheds
in contour detection. In Int.workshop on image
processing, real-time edge and motion detection.
Mestar, A., Vannoorenberghe, P., Flouzat, G., April 2007.
Mathematical Morphology applied to Very High
Resolution Spatial images interpretation. In Urban
Remote Sensing Joint Event, pp. 1-7.
Pesaresi, M., Benediktsson, J.A., Feb. 2001. A new
approach for the morphological segmentation of high-
resolution satellite imagery. In Geoscience and
Remote Sensing,IEEE Transactions, Vol 39, Issue 2.
VISAPP 2009 - International Conference on Computer Vision Theory and Applications
240