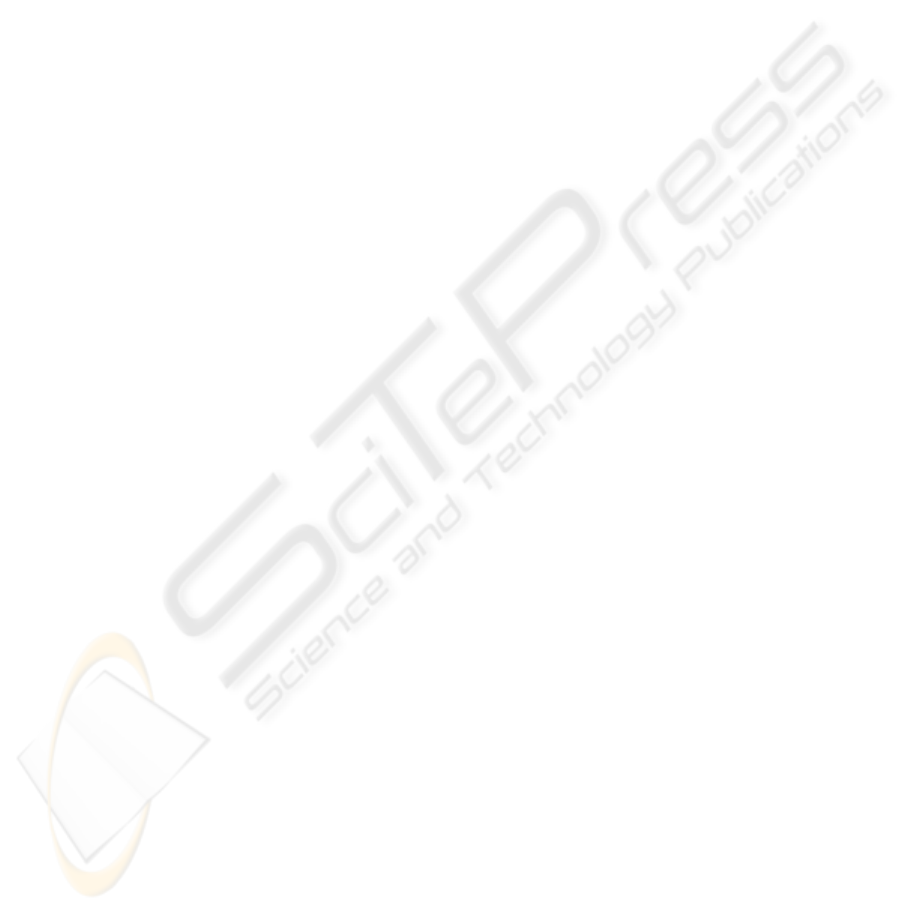
bor search is applied to assign it to the correct clus-
ter and hidden class, respectively. To demonstrate
the benefits of our approach we applied it for two
large publicly available datasets (i.e., COIL-100 and
ALOI). In fact, compared to a single model LDA we
get a much better classification results, which are even
competitive for large datasets containing up to 1000(!)
classes. Moreover, since the resulting data matrices
are much smaller the memory requirements and the
computational costs are dramatically reduced. Fu-
ture work will include to apply a more sophisticated
clustering, which, in fact, would further increase the
separability and thus the classification power of the
method.
ACKNOWLEDGEMENTS
This work was supported by the FFG project AUTO-
VISTA (813395) under the FIT-IT programme, and
the Austrian Joint Research Project Cognitive Vision
under projects S9103-N04 and S9104-N04.
REFERENCES
Belhumeur, P. N., Hespanha, J. P., and Kriegman, D. J.
(1997). Eigenfaces vs. Fisherfaces: Recognition Us-
ing Class Specific Linear Projection. IEEE Trans. on
Pattern Analysis and Machine Intelligence, 19(7):711
– 720.
Dempster, A. P., Laird, N. M., and Rubin, D. B. (1977).
Maximum Likelihood from Incomplete Data via the
EM Algorithm. Royal Statistical Society, 39(1):1 –
38.
Fisher, R. A. (1936). The Use of Multiple Measurements
in Taxonomic Problems. Annals of Eugenics, 7:179–
188.
Fukunaga, K. (1990). Introduction to Statistical Pattern
Recognition. Academic Press.
Geusebroek, J. M., Burghouts, G. J., and Smeulders, A.
W. M. (2005). The Amsterdam Library of Object Im-
ages. Computer Vision, 61(1):103 – 112.
Hunt, M. (1979). A statistical approach to metrics for word
and syllable recognition. In Meeting of the Acoustical
Society of American, volume 66, pages 535 – 536.
Kim, H., Kim, D., and Bang, S. Y. (2002). Face Recognition
Using LDA Mixture Model. In Proc. Intern. Conf. on
Pattern Recognition, volume 2, pages 486 – 489.
Kim, H., Kim, D., and Bang, S. Y. (2003). Extensions
of LDA by PCA Mixture Model and Class-Wise Fea-
tures. Pattern Recognition, 36(5):1095 – 1105.
Kim, T.-K., Kittler, J., and Cipolla, R. (2007). Discrimina-
tive Learning and Recognition of Image Set Classes
Using Canonical Correlations. IEEE Trans. on Pat-
tern Analysis and Machine Intelligence, 29(6):1005 –
1018.
Loog, M., Duin, R. P. W., and Haeb-Umbach, R. (2001).
Multiclass Linear Dimension Reduction by Weighted
Pairwise Fisher Criteria. IEEE Trans. on Pattern Anal-
ysis and Machine Intelligence, 23(7):762 – 766.
Mart
´
ınez, A. M. and Zhu, M. (2005). Where are Lin-
ear Feature Extraction Methods Applicable? IEEE
Trans. on Pattern Analysis and Machine Intelligence,
27(12):1934 – 1944.
Nene, S. A., Nayar, S. K., and Murase, H. (1996). Columbia
Object Image Library (COIL-100). Technical Report
CUCS-006-96, Columbia University.
Rao, C. R. (1948). The Utilization of Multiple Measure-
ments in Problems of Biological Classification. Royal
Statistical Society – Series B, 10(2):159 – 203.
Strang, G. (2006). Linear Algebra and Its Applications.
Brooks/Cole.
Swets, D. L. and Weng, J. (1996). Using Discriminant
Eigenfeatures for Image Retrieval. IEEE Trans. on
Pattern Analysis and Machine Intelligence, 18(8):831
– 837.
Tipping, M. E. and Bishop, C. M. (1999). Mixtures of
Probabilistic Principal Component Analyzers. Neural
Computation, 11(2):443 – 482.
Zhou, D. and Yang, X. (2004). Face Recognition Using
Direct-Weighted LDA. In Proc. of the Pacific Rim
Int. Conference on Artificial Intelligence, pages 760 –
768.
VISAPP 2009 - International Conference on Computer Vision Theory and Applications
306