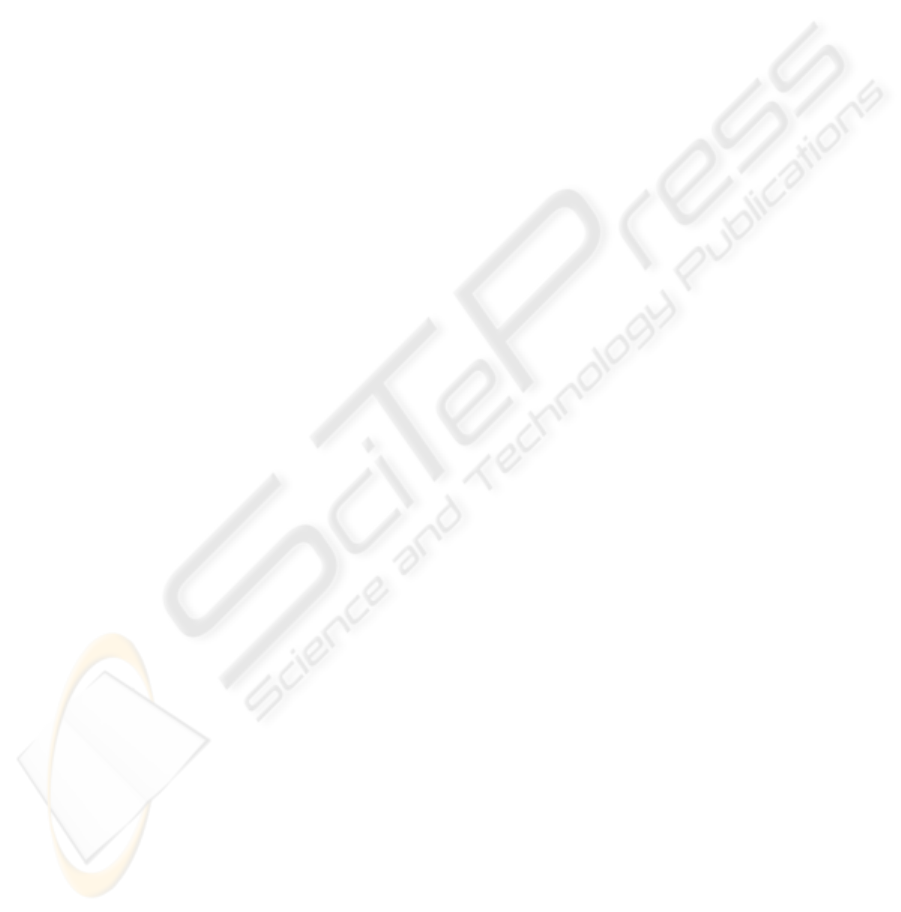
In the image (c.) the segmentation was done without
using the a priori knowledge, one can see that water-
shed basins follow approximately the labels borders.
Using a priori knowlege (image (d.)) enhances the
quality of these borders by forcing pre-existing bor-
ders (e.g. (3) and (4) in figure 3). Of course a label
present in 1999 and not present in 2004 will create in-
correct borders as illustrated by (5) and (6) in figure
3. For both approaches (with and without a priori)
the method is able to segment thin structures (e.g. as
illustrated by (7) in figure 3(c.)). New structures are
well detected (e.g. (1) and (2) in figure 3 with and
without using a priori knowledge. Man made struc-
tures such as houses and roads are well segmented
(a small over-segmentation occurs on different roof
slopes). Globally, all the objects are well detected in
the sense that all object borders are included inside
the segmentation for both methods using or not using
a priori knowledge (pre-existing borders). Most la-
belized objects are over-segmented, as illustrated by
(2) in figure 3 , this is mainly due to the existing con-
trast (robust borders) inside objects of interest. Over-
segmentation, if limited, would be addressed in a fur-
ther classification scheme not illustrated here. The
injection of a priori knowledge is well illustrated in
figure 3 (d.), borders of object (4) are found by the
segmentation procedure while randomized watershed
assembly without using priors gives more irregular
borders (object (3)). In the case of object that dis-
appears from one image to the other (as illustrated by
(6) where an object present in the database is missing
in the new image), injection of a priori knowledge
may generate false borders. This problem is also con-
sidered as over-segmentation and will be tackled in
further classification steps.
5 PARAMETERS SETTINGS
As often the presented segmentation method relies on
several parameters. Practitioner likes to have some
rule of the thumb to have at least a starting point for
the parameters setting. The method we propose re-
quires the settings of basically four parameters : (i.)
the marker density function (DENSITY), (ii.) the ran-
dom slope (MAXSLOPE), (iii.) the number of voters
(NITER) and (iv.) the threshold of the counter value
(COUNT
th
). We tested the variations observed on the
results for a typical range for each of these parameters
and summarized the results below.
Figure 4 (a.) shows how relative missed border
evolves with respect to COUNT
th
. The curve exhibits
a minimum value around 10 on a total number of iter-
ations equal to 35 which means that a border is to be
considered robust if its occurrence is higher that 1/3
of the total number of iterations. DENSITY of the
random markers influences positively the number of
segments as illustrated in figure 4 (b.) which is con-
sistent with the marked watershed properties. Con-
cerning the MAXSLOPE parameter, it is negatively
linked with the number of ADD
rel
borders (figure 4
(c.)), as well as with the number of segments (data not
shown). This is coherent with the fact that small local
gradient can be randomly smoothed by the SLOPE
perturbation. TheCOUNT
th
parameter tunes the level
of over-segmentation, it can be easily set interactively
by the user to select the segmentation granularity.
6 CONCLUSIONS
We propose the use of a weakened assembly of
marked watershed transform for segmenting remote
sensed imagery. This technique relies on the pertur-
bation of the gradient image on one hand and on a
random marking on the other hand. This approach
also allows to take previsouly detected borders into
account, which is useful when applied to map up-
date. Different method parameters are identified and
characterized with respect to the quality of the seg-
mentation. Watershed randomization allows to detect
small (thin) objects but also allows to limit the ob-
tained segments only to the stable ones (i.e. limiting
the over-segmentation). In comparison to manual la-
belling, the proposed method still gives more labels.
However the obtained borders are consistent with the
supervision, meaning that expected labels are well de-
tected, but composed of few sub-labels. We show
that under-segmentation is kept low by evaluating the
missed borders. In this work, we do not use any spec-
tral or contextualinformation. This will be considered
in further automatic classification process.
ACKNOWLEDGEMENTS
The authors want to thank IGN Belgium for giving
access to the TOP10V-GIS database, the DGA for the
remote sensed image, and F. De Groef for the proof-
ing. The image data were funded by the European
Commission and made available by the JRC IPSC
GeoCAP unit through the Ministry of Agriculture
(Walloon Region, Belgium). Debeir O., Simler C.
and Atoui H. are granted by IRSIB-IWOIB Institute
for the encouragement of Scientific Research and
Innovation of Brussels. The authors want to thank the
reviewers for there constructive remarks.
WEAKENED WATERSHED ASSEMBLY FOR REMOTE SENSING IMAGE SEGMENTATION AND CHANGE
DETECTION
133